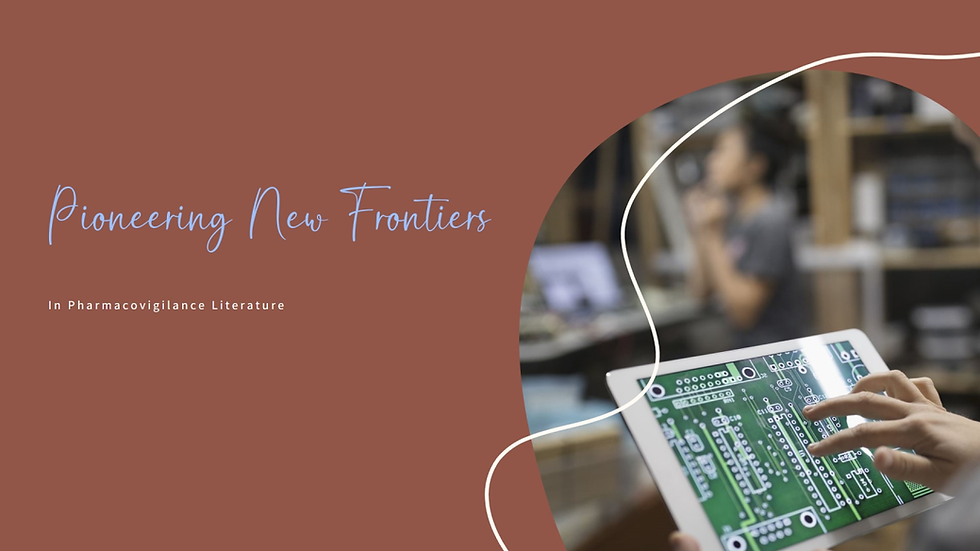
The landscape of pharmacovigilance literature is undergoing a transformative shift, propelled by the rapid advancements in artificial intelligence (AI) technology. As AI continues to revolutionize various industries, its impact on pharmacovigilance research and literature is becoming increasingly profound. This blog embarks on a journey through the realm of AI explorers, pioneering new frontiers in pharmacovigilance literature, and examines the implications of these advancements on drug safety monitoring, signal detection, and regulatory compliance.
The Rise of AI in Pharmacovigilance Literature:
Unleashing the Power of AI
AI technologies, including machine learning, natural language processing (NLP), and predictive analytics, have emerged as invaluable tools for analyzing vast amounts of pharmacovigilance data. By automating data extraction, analysis, and interpretation, AI accelerates the pace of pharmacovigilance research and enhances the efficiency and accuracy of literature reviews.
Revolutionizing Signal Detection
Signal detection, the process of identifying potential safety concerns associated with medicinal products, is a cornerstone of pharmacovigilance literature. AI-driven algorithms can analyze diverse sources of data, including scientific literature, electronic health records, and social media, to identify emerging safety signals and trends with unprecedented speed and precision.
Enhancing Regulatory Compliance
Regulatory authorities, such as the FDA and EMA, rely on pharmacovigilance literature to assess the safety profiles of drugs and make informed regulatory decisions. AI-powered literature reviews provide regulators with comprehensive insights into adverse drug reactions, drug interactions, and other safety-related issues, facilitating regulatory compliance and risk management.
Applications of AI in Pharmacovigilance Literature:
Automated Literature Screening
AI algorithms can streamline the process of literature screening by automatically identifying relevant articles and extracting pertinent information. Natural language processing techniques enable AI systems to analyze text, classify articles based on relevance to pharmacovigilance, and extract key data elements, such as adverse drug reactions and patient demographics.
Literature Synthesis and Analysis
AI-driven literature synthesis platforms aggregate and analyze data from diverse sources, enabling researchers to identify patterns, trends, and associations within pharmacovigilance literature. Predictive analytics algorithms can forecast future safety risks based on historical data, empowering healthcare providers and regulators to proactively manage drug safety concerns.
Real-Time Signal Monitoring
AI-powered signal monitoring systems continuously monitor pharmacovigilance literature in real time, alerting healthcare providers and regulators to emerging safety signals and trends. By analyzing online forums, social media platforms, and healthcare databases, these systems provide early warnings of potential adverse drug reactions, enabling timely intervention and risk mitigation.
Challenges and Considerations:
Data Quality and Standardization
Ensuring the quality and standardization of data is essential for the reliability and accuracy of AI-driven pharmacovigilance literature reviews. Variations in data formats, terminology, and reporting practices can affect the performance of AI algorithms and lead to inconsistencies in signal detection and analysis.
Solutions:
Data Harmonization: Implementing standardized data models and terminologies, such as Medical Dictionary for Regulatory Activities (MedDRA) and Systematized Nomenclature of Medicine-Clinical Terms (SNOMED CT), promotes consistency and interoperability in pharmacovigilance literature.
Quality Assurance Measures: Incorporating quality assurance protocols, such as data validation checks and peer review processes, ensures the integrity and reliability of AI-generated literature reviews.
Regulatory Acceptance and Validation:
Achieving regulatory acceptance of AI-driven pharmacovigilance literature reviews requires rigorous validation and verification of AI algorithms and methodologies. Regulators must evaluate the performance, accuracy, and reliability of AI systems to ensure compliance with regulatory standards and guidelines.
Solutions:
Validation Studies: Conducting comprehensive validation studies, including benchmarking against gold standard datasets and comparison with human experts, demonstrates the reliability and effectiveness of AI algorithms in pharmacovigilance literature reviews.
Transparency and Explain ability: Enhancing the transparency and explain ability of AI-driven processes, such as providing clear documentation of algorithms and decision-making criteria, facilitates regulatory review and acceptance.
Ethical and Legal Considerations:
Ethical and legal considerations, including patient privacy, data security, and algorithmic bias, are paramount in AI-driven pharmacovigilance literature reviews. Safeguarding patient rights and ensuring fair and equitable use of data are essential principles guiding the development and deployment of AI systems.
Solutions:
Privacy Protection Measures: Implementing robust data anonymization and encryption techniques, along with strict access controls and data governance policies, safeguards patient privacy and confidentiality.
Bias Detection and Mitigation: Employing bias detection algorithms and fairness metrics helps identify and mitigate potential biases in AI models, ensuring equitable outcomes and reducing the risk of algorithmic discrimination.
Future Directions and Opportunities:
Collaboration and Knowledge Sharing
Collaboration among stakeholders, including researchers, healthcare providers, regulators, and technology vendors, is essential for advancing AI-driven pharmacovigilance literature reviews. Knowledge sharing initiatives, collaborative research projects, and public-private partnerships foster innovation and best practices in drug safety monitoring.
Integration with Real-World Data
The integration of AI-driven pharmacovigilance literature reviews with real-world data sources, such as electronic health records, patient registries, and wearable devices, offers new opportunities for enhancing drug safety monitoring and signal detection. By leveraging diverse data sources, AI systems provide a more comprehensive understanding of drug safety profiles and enable personalized risk assessment and management strategies.
Continuous Improvement and Adaptation
Continuous improvement and adaptation are key principles guiding the evolution of AI-driven pharmacovigilance literature reviews. As technology advances and new data sources emerge, AI algorithms must evolve to address emerging challenges and opportunities in drug safety monitoring.
Conclusion:
AI-driven pharmacovigilance literature reviews are at the forefront of innovation, transforming the landscape of drug safety monitoring and signal detection. By leveraging the power of AI technologies, researchers, healthcare providers, and regulators can unlock new insights, accelerate decision-making, and enhance patient safety in the ever-evolving field of pharmacovigilance literature. As AI explorers continue to pioneer new frontiers, the future of drug safety monitoring promises to be more efficient, effective, and patient-centered than ever before.
Comments