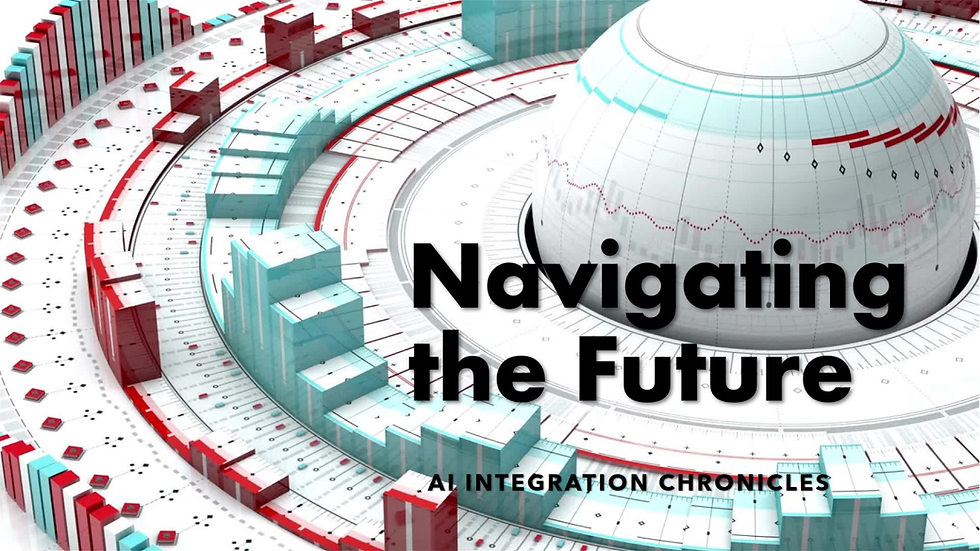
In the ever-evolving landscape of healthcare, pharmacovigilance stands as a crucial component ensuring the safety of medications post-market approval. With the constant influx of new drugs and the imperative to monitor their effects on patients, the pharmacovigilance process has traditionally been labor-intensive and time-consuming. However, the advent of Artificial Intelligence (AI) has promised to revolutionize this field, offering novel solutions to streamline and enhance pharmacovigilance reviews. In this blog, we embark on a journey through the integration of AI in pharmacovigilance, exploring its benefits, challenges, and the future it holds.
Understanding Pharmacovigilance:
Before delving into the integration of AI, let's grasp the essence of pharmacovigilance. It encompasses the detection, assessment, understanding, and prevention of adverse effects or any other drug-related problems. Pharmacovigilance aims to ensure that medications in circulation are safe for consumption, identifying and mitigating potential risks promptly.
The Promise of AI in Pharmacovigilance:
AI brings a plethora of opportunities to enhance pharmacovigilance processes:
Efficiency: One of the most significant advantages of AI is its ability to process vast amounts of data rapidly. AI algorithms can sift through mountains of patient data, medical records, and adverse event reports to identify patterns and signals that might indicate previously unknown adverse effects.
Early Detection: By continuously monitoring real-world data, AI systems can detect potential safety concerns earlier than traditional methods. This early detection enables timely intervention, preventing harm to patients and minimizing risks associated with medications.
Signal Detection: AI algorithms excel at identifying subtle signals and associations within complex datasets. By analyzing structured and unstructured data sources, including social media, electronic health records, and biomedical literature, AI can uncover potential safety issues that might have been overlooked using conventional methods.
Risk Prediction: Machine learning models can predict the likelihood of adverse events based on various patient factors, medication history, and other relevant variables. These predictive analytics enable healthcare providers to proactively manage risks and tailor treatment plans to individual patients.
Automation: AI-powered tools can automate repetitive tasks, such as data entry, case triaging, and literature review, freeing up pharmacovigilance professionals to focus on more complex tasks requiring human expertise.
Challenges and Considerations:
Despite its immense potential, the integration of AI in pharmacovigilance is not without challenges:
Data Quality: The effectiveness of AI algorithms relies heavily on the quality and completeness of the data they are trained on. Ensuring the integrity and accuracy of pharmacovigilance data is paramount to the success of AI-driven initiatives.
Regulatory Compliance: Regulatory agencies impose stringent requirements for pharmacovigilance activities to ensure patient safety. Integrating AI into existing pharmacovigilance processes necessitates compliance with regulatory standards and guidelines, which may vary across jurisdictions.
Interpretability: The black-box nature of some AI algorithms poses challenges in interpreting their decisions and recommendations. Establishing trust and transparency in AI-driven pharmacovigilance systems requires methods for explaining and validating their outputs.
Ethical Considerations: AI applications in healthcare raise ethical concerns regarding patient privacy, consent, and bias mitigation. Safeguarding patient rights and ensuring equitable access to healthcare are essential considerations in the development and deployment of AI-driven pharmacovigilance solutions.
The Future Landscape:
Looking ahead, the future of pharmacovigilance is intertwined with AI inextricably. As AI technologies continue to advance, we can anticipate several developments in this domain:
Personalized Pharmacovigilance: AI algorithms will enable the customization of pharmacovigilance strategies based on individual patient characteristics, medical histories, and genetic profiles, ensuring tailored monitoring and intervention approaches.
Predictive Analytics: AI-driven predictive models will become more sophisticated, accurately forecasting adverse events and identifying patients at heightened risk, allowing proactive intervention and risk mitigation.
Real-Time Surveillance: AI-powered surveillance systems will provide real-time monitoring of drug safety signals, facilitating rapid response to emerging safety concerns and enabling agile decision-making by healthcare stakeholders.
Collaborative Ecosystems: AI will foster collaboration among healthcare stakeholders, including pharmaceutical companies, regulatory agencies, healthcare providers, and patients, facilitating data sharing and collective efforts to enhance drug safety.
Conclusion:
The integration of AI holds immense promise for the future of pharmacovigilance, offering opportunities to enhance efficiency, accuracy, and timeliness in monitoring medication safety. By harnessing the power of AI-driven analytics, healthcare stakeholders can navigate the complexities of pharmacovigilance more effectively, ensuring the continued safety and well-being of patients worldwide. However, realizing the full potential of AI in pharmacovigilance requires addressing various challenges, including data quality, regulatory compliance, interpretability, and ethical considerations. As we embark on this journey towards a future where AI and pharmacovigilance converge, collaboration, innovation, and a steadfast commitment to patient safety will pave the way forward.
Learn more about Crypta and discover how your organization can transition to a Literature Review Software that supports Local Literature Review. Ready to see it in action? Request a demo today.
Comments