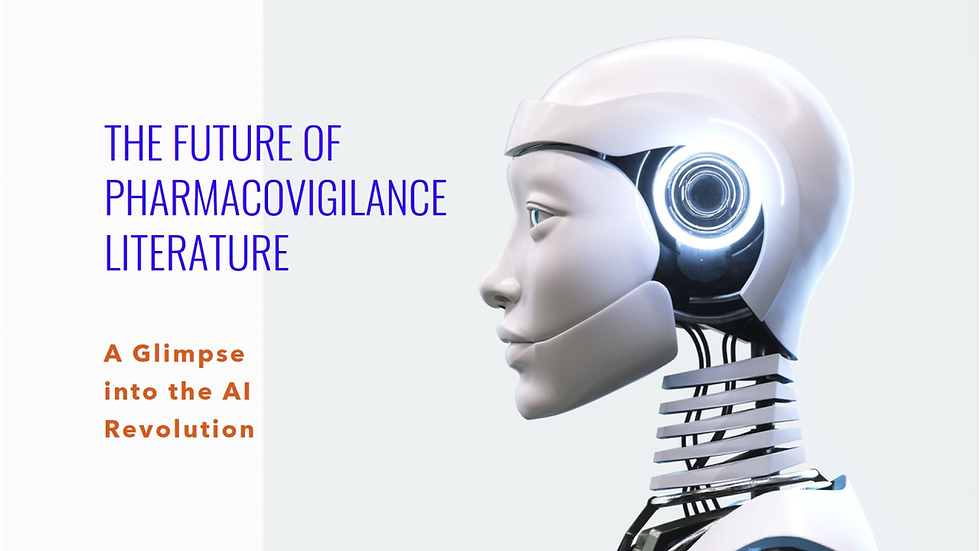
In recent years, the field of pharmacovigilance has witnessed a remarkable transformation propelled by advances in artificial intelligence (AI) and data analytics. AI algorithms are increasingly being deployed to streamline pharmacovigilance processes, enhance signal detection, and improve the efficiency and accuracy of adverse event monitoring. As we peer into the future of pharmacovigilance literature, it becomes evident that AI will play a pivotal role in shaping the landscape of drug safety surveillance and revolutionizing the way we detect, assess, and manage adverse drug reactions (ADRs). This blog explores the burgeoning intersection of AI and pharmacovigilance literature, offering insights into the transformative potential of AI-driven approaches in ensuring medication safety and improving patient outcomes.
AI-powered signal detection represents a paradigm shift in pharmacovigilance, offering the promise of more proactive and predictive approaches to identifying potential safety concerns. Traditional signal detection methods rely on manual review of pharmacovigilance databases, spontaneous reporting systems, and scientific literature, which can be time-consuming and prone to human error. AI algorithms, on the other hand, can analyze vast amounts of structured and unstructured data with speed and precision, uncovering hidden patterns and associations that may indicate previously unrecognized ADRs. By leveraging machine learning techniques such as natural language processing (NLP) and deep learning, AI systems can sift through diverse data sources to identify emerging safety signals in real time, enabling healthcare providers and regulatory agencies to take swift action to mitigate potential risks.
Furthermore, AI-driven pharmacovigilance literature holds the promise of personalized medicine, tailoring drug safety assessments to individual patient characteristics and medical histories. By integrating electronic health records (EHRs), genomics data, and other patient-specific information, AI algorithms can identify subpopulations at heightened risk of experiencing adverse drug reactions, allowing healthcare providers to personalize treatment regimens and optimize medication safety. This personalized approach not only enhances patient care but also contributes to the ongoing shift toward precision medicine, where treatments are tailored to the unique needs and characteristics of each patient.
Another area where AI is poised to revolutionize pharmacovigilance literature is in the realm of drug repurposing and pharmacovigilance. AI algorithms can analyze large-scale drug databases, molecular datasets, and clinical trial data to identify novel therapeutic indications for existing drugs, as well as potential drug-drug interactions and adverse event profiles. By repurposing existing medications for new indications, AI-driven pharmacovigilance has the potential to accelerate the drug discovery and development process, reduce costs, and bring new treatments to market more quickly. Moreover, AI can help identify drug combinations that offer synergistic therapeutic effects while minimizing the risk of adverse reactions, paving the way for more effective and personalized treatment strategies.
Furthermore, AI-powered literature reviews and systematic analyses have the potential to revolutionize evidence synthesis in pharmacovigilance. Traditional literature reviews are often time-consuming and subject to publication bias, limiting the comprehensiveness and reliability of the evidence base. AI algorithms can automatically extract, synthesize, and analyze information from vast repositories of scientific literature, identifying relevant studies, extracting key findings, and summarizing evidence on drug safety and efficacy. By automating the literature review process, AI-driven approaches can provide more comprehensive and up-to-date evidence for pharmacovigilance decision-making, enabling healthcare providers and regulatory agencies to make more informed and timely decisions about medication use.
However, the integration of AI into pharmacovigilance literature also presents certain challenges and considerations. Ethical and regulatory considerations surrounding data privacy, transparency, and accountability must be carefully addressed to ensure the responsible and ethical use of AI in drug safety surveillance. Moreover, the potential for algorithmic bias and unintended consequences of AI-driven decision-making necessitate ongoing monitoring and validation of AI systems to ensure their reliability and fairness. Additionally, the need for interdisciplinary collaboration between data scientists, pharmacovigilance experts, healthcare providers, and regulatory agencies is paramount to harnessing the full potential of AI in pharmacovigilance literature and translating AI-driven insights into actionable strategies for improving patient safety and public health.
Conclusion:
In conclusion, the future of pharmacovigilance literature is undeniably intertwined with the transformative power of AI. As AI algorithms continue to evolve and mature, they hold the promise of revolutionizing drug safety surveillance, enabling more proactive, personalized, and evidence-based approaches to pharmacovigilance. By embracing AI-driven innovation and fostering interdisciplinary collaboration, we can harness the full potential of AI to advance the science of pharmacovigilance, enhance medication safety, and improve patient outcomes in the years to come. As we embark on this journey into the AI revolution, let us remain vigilant in our commitment to leveraging technology for the greater good of public health and medication safety.
Comments