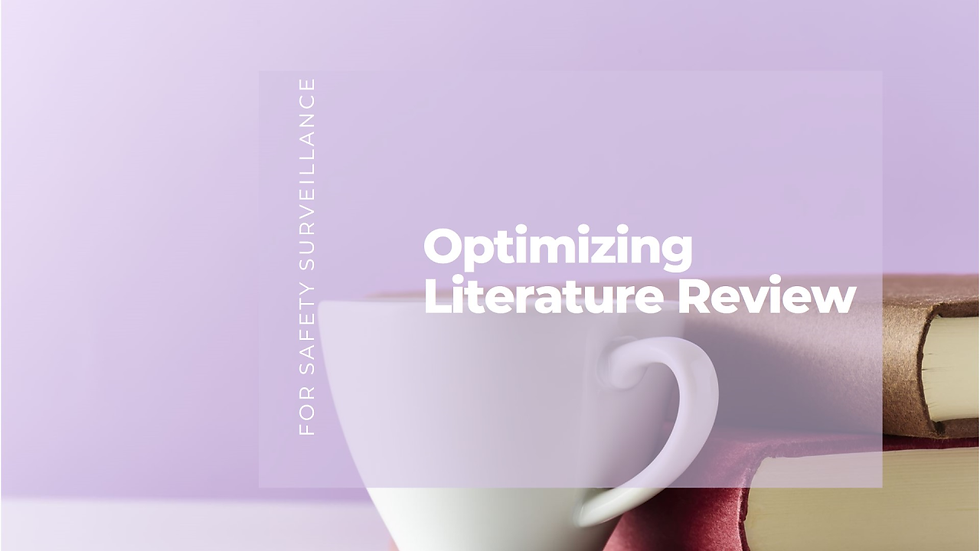
In the pharmaceutical and healthcare industries, the literature review process is a critical component of safety surveillance. Traditionally, this process involves meticulous and labor-intensive examination of scientific publications to identify potential safety signals and adverse drug reactions (ADRs). However, the advent of artificial intelligence (AI) is revolutionizing this field, making the literature review process more efficient, accurate, and comprehensive. This blog explores the transformative impact of AI on literature reviews for safety surveillance, detailing the technologies involved, their benefits, and the future of AI-driven pharmacovigilance.
The Importance of Literature Review in Safety Surveillance:
Safety surveillance, also known as pharmacovigilance, involves the continuous monitoring of the safety of pharmaceutical products throughout their lifecycle. A crucial part of this process is the literature review, which aims to:
Identify New Safety Signals: Detect emerging adverse effects that were not apparent during clinical trials.
Verify Known Risks: Monitor and confirm known risks associated with a drug.
Evaluate Risk-Benefit Profiles: Assess the overall safety profile of a drug to inform regulatory decisions and clinical practice.
The literature review process traditionally requires extensive manual effort, including:
Searching Databases: Scouring multiple databases for relevant scientific articles.
Screening Articles: Evaluating titles and abstracts to identify pertinent studies.
Data Extraction: Extracting relevant safety information from full-text articles.
Synthesis and Reporting: Compiling and synthesizing data into coherent reports for regulatory bodies and healthcare providers.
Given the ever-growing volume of scientific literature, this manual process is increasingly untenable. Enter AI, with its potential to transform the literature review landscape.
How AI is Revolutionizing Literature Reviews:
AI, particularly machine learning (ML) and natural language processing (NLP), offers powerful tools to automate and enhance the literature review process. Here’s how AI technologies are optimizing each stage of the review process:
Automated Database Searching
AI-driven systems can automate the search process across various scientific databases, including PubMed, and clinical trial registries. By using advanced algorithms, these systems can:
Expand Search Scope: AI can simultaneously search multiple databases, ensuring a comprehensive literature search.
Refine Search Queries: Machine learning models can refine and optimize search queries to reduce irrelevant results and increase the precision of retrieved articles.
Update Searches: AI can continuously monitor and update search results as new literature is published, ensuring up-to-date surveillance.
Efficient Screening and Selection
Screening articles for relevance is a labor-intensive step that AI can streamline significantly. AI algorithms, particularly those based on NLP, can:
Prioritize Articles: Machine learning models can rank articles based on relevance, allowing human reviewers to focus on the most pertinent studies first.
Automate Screening: AI can automatically screen titles and abstracts, filtering out irrelevant studies with high accuracy.
Reduce Bias: By standardizing the screening criteria, AI reduces the risk of human bias in the selection process.
Accurate Data Extraction
Extracting relevant data from full-text articles is another area where AI excels. NLP algorithms can:
Identify Key Information: NLP models can extract specific information such as drug names, dosages, patient demographics, and reported adverse events.
Summarize Findings: AI can generate concise summaries of safety data, facilitating quicker understanding and synthesis.
Handle Large Volumes: AI can process vast amounts of text quickly and accurately, far surpassing human capabilities.
Comprehensive Data Synthesis and Reporting
Once the data is extracted, AI can assist in synthesizing and reporting the findings:
Pattern Recognition: Machine learning models can identify patterns and trends in the data, highlighting potential safety signals.
Generate Reports: AI can automate the generation of comprehensive safety reports, ensuring consistency and reducing the time required for manual report writing.
Enhance Visualization: AI tools can create sophisticated visualizations of safety data, aiding in the interpretation and communication of findings.
Benefits of AI-Driven Literature Reviews:
The integration of AI into the literature review process for safety surveillance offers several significant benefits:
Improved Efficiency
AI dramatically reduces the time required to conduct literature reviews. Automated search, screening, and data extraction processes allow for rapid identification and analysis of relevant studies, freeing up valuable time for human experts to focus on interpretation and decision-making.
Enhanced Accuracy
AI systems, particularly those using NLP, have demonstrated high accuracy in identifying relevant studies and extracting critical data. This reduces the risk of human error and ensures more reliable safety assessments.
Comprehensive Coverage
AI's ability to simultaneously search multiple databases and continuously update search results ensures comprehensive coverage of the scientific literature. This is crucial for maintaining up-to-date safety profiles of pharmaceutical products.
Cost Savings
By automating labor-intensive tasks, AI reduces the overall cost of literature reviews. This makes safety surveillance more affordable and accessible, particularly for smaller organizations with limited resources.
Scalability
AI-driven literature review processes are highly scalable. As the volume of scientific literature continues to grow, AI can easily scale to handle the increased workload, ensuring that no relevant information is overlooked.
Case Study: AI in Action
To illustrate the impact of AI on literature reviews for safety surveillance, consider the following case study of a pharmaceutical company, PharmaTech, implementing AI-driven solutions.
Background
PharmaTech, a global pharmaceutical company, was facing challenges in maintaining up-to-date safety profiles for its extensive product portfolio. The traditional manual literature review process was time-consuming and prone to delays, risking regulatory non-compliance.
Implementation Strategy
Pharma Tech decided to integrate AI into its literature review process, focusing on three key areas:
Automated Search and Screening: Pharma Tech implemented an AI-driven search and screening platform capable of simultaneously searching multiple databases and automatically screening titles and abstracts for relevance.
NLP for Data Extraction: The company adopted NLP tools to automate the extraction of safety data from full-text articles, focusing on key information such as adverse event reports, patient demographics, and drug interactions.
Machine Learning for Pattern Recognition: PharmaTech utilized machine learning models to analyze extracted data, identifying potential safety signals and generating comprehensive safety reports.
Outcomes
The implementation of AI-driven literature review processes yielded several positive outcomes for Pharma Tech:
Reduced Review Time: The time required to complete literature reviews was reduced by over 50%, allowing for more timely updates to safety profiles.
Increased Accuracy: The AI systems demonstrated high accuracy in identifying relevant studies and extracting critical safety data, reducing the risk of human error.
Cost Savings: The automation of labor-intensive tasks led to significant cost savings, enabling PharmaTech to allocate resources more efficiently.
Enhanced Regulatory Compliance: By maintaining up-to-date safety profiles, Pharma Tech improved its compliance with regulatory requirements, avoiding potential penalties and product recalls.
The Future of AI-Driven Literature Reviews:
As AI technology continues to advance, its role in optimizing literature reviews for safety surveillance is set to expand further. Here are some future trends to watch:
Integration with Real-World Data
The integration of AI-driven literature reviews with real-world data (RWD) sources, such as electronic health records (EHRs) and patient registries, will provide a more comprehensive view of drug safety. Combining insights from scientific literature and RWD will enhance the detection of safety signals and inform more robust risk-benefit assessments.
Advances in NLP and ML
Continued advancements in NLP and ML will further improve the accuracy and efficiency of AI-driven literature reviews. Emerging technologies, such as deep learning and transformer models, hold promise for even more sophisticated data extraction and analysis capabilities.
Personalized Medicine
AI-driven literature reviews will play a crucial role in the era of personalized medicine. By analyzing vast amounts of data, AI can help identify patient-specific safety profiles, informing more personalized and effective treatment strategies.
Collaborative Platforms
Future AI-driven literature review systems will likely feature collaborative platforms that facilitate information sharing and collaboration among researchers, clinicians, and regulatory authorities. These platforms will enhance transparency and ensure that safety data is accessible to all relevant stakeholders.
Ethical Considerations and Regulatory Oversight
As AI becomes more integrated into literature reviews and safety surveillance, ethical considerations and regulatory oversight will be paramount. Ensuring the transparency, accountability, and fairness of AI algorithms will be crucial for maintaining public trust and achieving reliable safety assessments.
Conclusion:
AI is poised to revolutionize the literature review process for safety surveillance, offering significant benefits in terms of efficiency, accuracy, and comprehensiveness. By automating labor-intensive tasks and enhancing data analysis capabilities, AI-driven systems can transform pharmacovigilance practices, ultimately leading to safer pharmaceutical products and better patient outcomes.
The case of PharmaTech illustrates the practical impact of AI integration, demonstrating how AI can streamline processes, reduce costs, and improve regulatory compliance. As AI technology continues to evolve, its role in optimizing literature reviews for safety surveillance will only expand, paving the way for more robust and effective pharmacovigilance practices.
Organizations that embrace AI-driven solutions will be well-positioned to navigate the complexities of modern safety surveillance, ensuring that they can meet regulatory requirements, protect patient safety, and advance the field of pharmacovigilance. The future of AI in literature reviews is bright, and its precision play will be a game-changer for the industry.
Comments