AI Trailblazers: Redefining Literature Review Strategies for Safety
- Chaitali Gaikwad
- May 20, 2024
- 3 min read
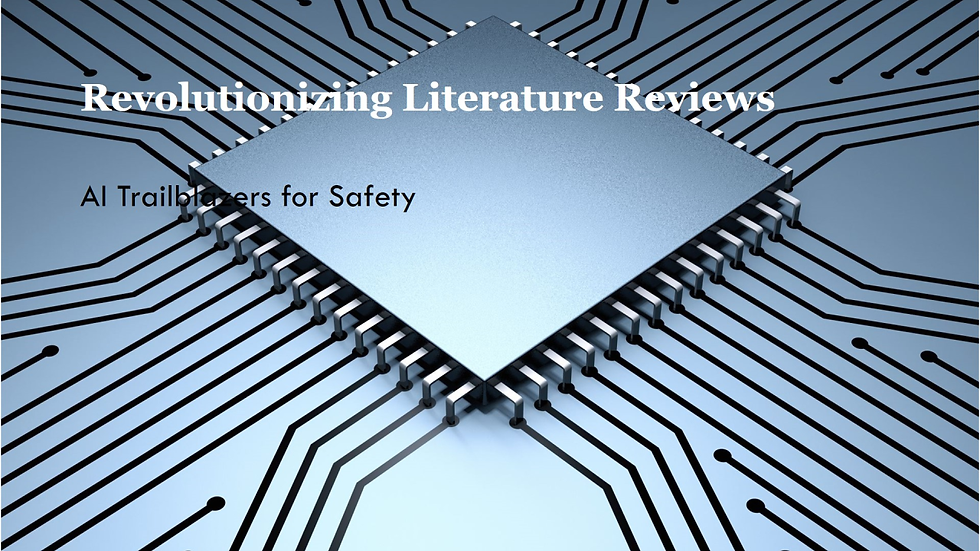
In the realm of drug safety and pharmacovigilance, staying abreast of the latest research findings and safety concerns is paramount. Literature review serves as a cornerstone in this process, but traditional methods can be time-consuming and resource-intensive. However, with the advent of artificial intelligence (AI), there's a new frontier in literature review strategies. In this blog post, we'll explore how AI trailblazers are redefining literature review strategies for safety, revolutionizing the landscape of drug safety monitoring and ensuring the continued safety of medications.
The Need for Innovation in Literature Review:
Before delving into the impact of AI on literature review, it's crucial to understand the challenges that traditional literature review methods pose. The sheer volume of scientific literature produced each year can be overwhelming, making it difficult for pharmacovigilance professionals to identify relevant studies, extract meaningful insights, and stay ahead of emerging safety concerns. Moreover, manual literature review processes are time-consuming and labor-intensive, often resulting in delays in safety signal detection and regulatory action.
AI-Powered Literature Review: A Paradigm Shift:
Artificial intelligence offers a promising solution to the challenges of traditional literature review methods. By leveraging machine learning algorithms, natural language processing (NLP) techniques, and advanced data analytics, AI systems can rapidly analyze vast repositories of scientific literature with unparalleled speed and accuracy. These AI-powered approaches have the potential to revolutionize literature review strategies for safety, enabling pharmacovigilance professionals to identify relevant studies, extract key insights, and detect safety signals more efficiently and effectively than ever before.
Automated Literature Screening:
One of the key applications of AI in literature review is automated screening of scientific literature. AI algorithms can be trained to analyze large databases of scholarly articles and identify studies that are relevant to specific safety queries. By using advanced text mining and classification techniques, AI systems can sift through vast amounts of literature, extracting key information from abstracts, titles, and full-text articles. This automated screening process not only saves time but also ensures that no potentially relevant information is overlooked, improving the efficiency and thoroughness of literature review efforts.
Natural Language Processing for Data Extraction:
Natural language processing (NLP) is another powerful tool in the AI arsenal for literature review. NLP algorithms can analyze the text of scientific articles to extract relevant data, such as adverse drug reactions, drug interactions, patient demographics, and study outcomes. By automatically extracting this information from the literature, NLP systems can help pharmacovigilance professionals quickly identify safety signals and trends, enabling more timely and informed decision-making. Additionally, NLP techniques can facilitate the synthesis and summarization of findings from multiple studies, providing a comprehensive overview of the evidence landscape.
Sentiment Analysis and Trend Detection:
In addition to extracting structured data from scientific literature, AI-powered systems can also perform sentiment analysis and trend detection. Sentiment analysis algorithms can assess the tone and sentiment of text, helping pharmacovigilance professionals gauge the overall perception of a drug or treatment modality within the scientific community. Trend detection algorithms can identify emerging topics, hot research areas, and shifting priorities, enabling researchers to stay ahead of the curve and identify new avenues for exploration in drug safety monitoring.
Leveraging Big Data and Real-World Evidence:
AI-powered literature review also benefits from access to big data and real-world evidence. By integrating data from diverse sources, including electronic health records, claims databases, social media, and patient forums, AI systems can provide a more comprehensive understanding of drug safety profiles and patient experiences. This holistic approach to data analysis enables pharmacovigilance professionals to identify safety signals earlier, detect rare adverse events, and assess the impact of interventions in real-world clinical settings.
Overcoming Challenges and Limitations:
While AI holds tremendous promise for redefining literature review strategies for safety, it's important to acknowledge and address potential challenges and limitations. AI algorithms are only as good as the data they're trained on, and biases or inaccuracies in training data can lead to erroneous results. Additionally, AI systems may struggle with understanding context or nuance in scientific literature, particularly when dealing with complex medical terminology or subtle linguistic cues. Furthermore, the black-box nature of some AI algorithms may raise concerns about transparency and interpretability, particularly in highly regulated domains such as pharmacovigilance.
Conclusion:
In conclusion, AI trailblazers are redefining literature review strategies for safety, ushering in a new era of efficiency, accuracy, and innovation in drug safety monitoring. By automating screening, extracting data, performing sentiment analysis, and leveraging big data and real-world evidence, AI-powered systems enable pharmacovigilance professionals to stay ahead of emerging safety concerns and ensure the continued safety of medications for patients worldwide. While challenges and limitations remain, ongoing research and innovation in the field of AI hold the promise of further advancements in literature review methods, ultimately benefiting patients, healthcare providers, and society as a whole. As we look to the future, AI-powered literature review will continue to blaze new trails in safety monitoring, driving scientific progress and innovation forward.
Comments