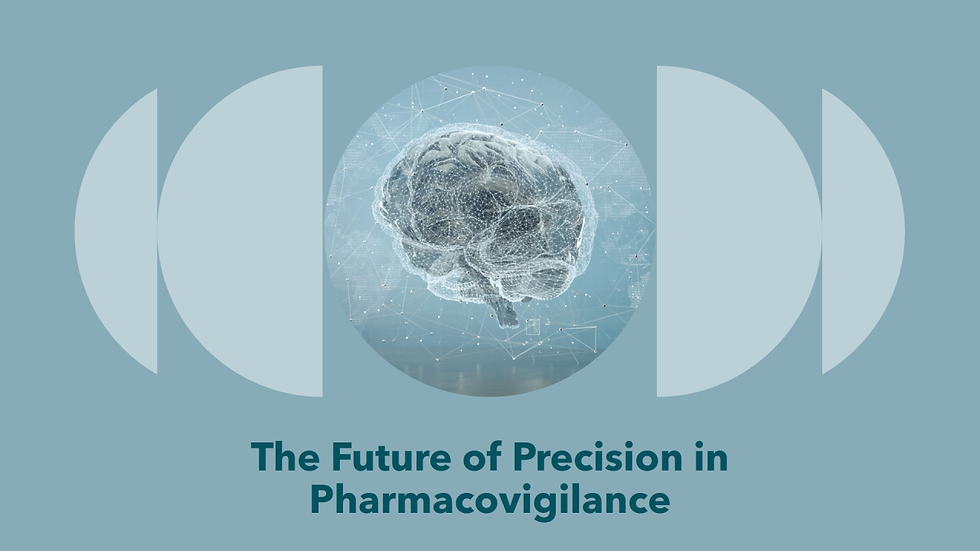
In the dynamic realm of pharmacovigilance, where the safety of medications is paramount, harnessing the power of artificial intelligence (AI) is poised to revolutionize the landscape of literature review and analysis. As the volume of biomedical literature continues to grow exponentially, traditional methods of literature review struggle to keep pace.
However, AI offers a promising solution, enabling researchers to sift through vast amounts of data with unprecedented speed and precision. This blog explores the potential of AI in pharmacovigilance literature, unveiling its transformative impact on safety surveillance, signal detection, and evidence synthesis.
The Challenge of Literature Review in Pharmacovigilance:
The proliferation of biomedical literature, including scientific articles, clinical trials, case reports, and regulatory documents, poses a significant challenge for pharmacovigilance professionals. The sheer volume and diversity of sources make it increasingly difficult to identify relevant information and extract meaningful insights efficiently.
Traditional literature review methods rely heavily on manual processes, such as keyword searches and manual screening of articles, which are time-consuming, labor-intensive, and prone to bias. Moreover, human reviewers may overlook relevant studies or struggle to synthesize findings from disparate sources, leading to gaps in knowledge and potentially overlooking important safety signals.
The Promise of AI in Pharmacovigilance Literature:
AI-powered text mining algorithms, such as natural language processing (NLP) and machine learning, offer sophisticated tools for automating literature review processes. By analyzing text data from diverse sources, these algorithms can identify relevant articles, extract key information, and uncover hidden patterns and associations, enabling researchers to gain insights at scale and with precision.
AI-driven literature review platforms have the potential to facilitate real-time signal detection by continuously monitoring and analyzing newly published studies and reports. By alerting pharmacovigilance professionals to emerging safety signals and trends, these platforms enable proactive risk management and timely intervention, enhancing patient safety and regulatory compliance.
Applications of AI in Pharmacovigilance Literature:
AI algorithms can streamline the screening and prioritization of literature by automatically identifying articles relevant to specific pharmacovigilance topics or products of interest. By triaging articles based on relevance and importance, these algorithms optimize the allocation of resources and ensure that researchers focus their attention on the most pertinent studies.
AI-driven literature review platforms facilitate evidence synthesis and knowledge discovery by systematically analyzing and summarizing findings from multiple studies. By aggregating data from diverse sources, these platforms enable researchers to identify patterns, trends, and associations that may not be apparent through manual review alone, fostering a deeper understanding of drug safety profiles and risk factors.
Challenges and Considerations:
The accuracy and reliability of AI-driven literature review platforms depend on the quality of input data and the robustness of algorithms. Biases in training data or algorithmic decision-making may introduce errors or inaccuracies, potentially compromising the validity of findings. Rigorous validation and quality assurance processes are essential to mitigate these risks and ensure the integrity of AI-driven analyses.
Interpretability and Transparency
AI algorithms often operate as "black boxes," making it challenging to understand the rationale behind their decisions. Ensuring interpretability and transparency in AI-driven literature review processes is crucial for building trust and confidence among stakeholders, including regulatory agencies, healthcare professionals, and patients. Efforts to enhance explain ability and accountability in AI models are necessary to address these concerns.
Future Directions and Opportunities:
The future of AI in pharmacovigilance literature lies in personalized approaches that tailor safety surveillance and risk assessment to individual patient characteristics and needs. By integrating patient-specific data, such as electronic health records and genetic information, AI-driven platforms can enhance the precision and relevance of safety analyses, enabling personalized risk management strategies and treatment recommendations.
Collaboration among stakeholders is essential for advancing the field of AI in pharmacovigilance literature. By sharing data, insights, and best practices, researchers, pharmaceutical companies, regulatory agencies, and technology providers can collectively drive innovation and accelerate the development and adoption of AI-driven solutions. Open collaboration platforms and data-sharing initiatives can facilitate knowledge exchange and collaboration across the pharmacovigilance ecosystem.
Conclusion:
The future of pharmacovigilance literature lies at the intersection of AI and precision medicine, where advanced technologies enable researchers to unlock insights and discoveries with unprecedented speed and accuracy. By harnessing the power of AI-driven text mining and data analytics, pharmacovigilance professionals can overcome the challenges of information overload, enhance signal detection, and advance patient safety. As AI continues to evolve and mature, its transformative impact on pharmacovigilance literature promises to reshape the landscape of drug safety surveillance and evidence synthesis, ushering in a new era of precision and efficiency.
Comments