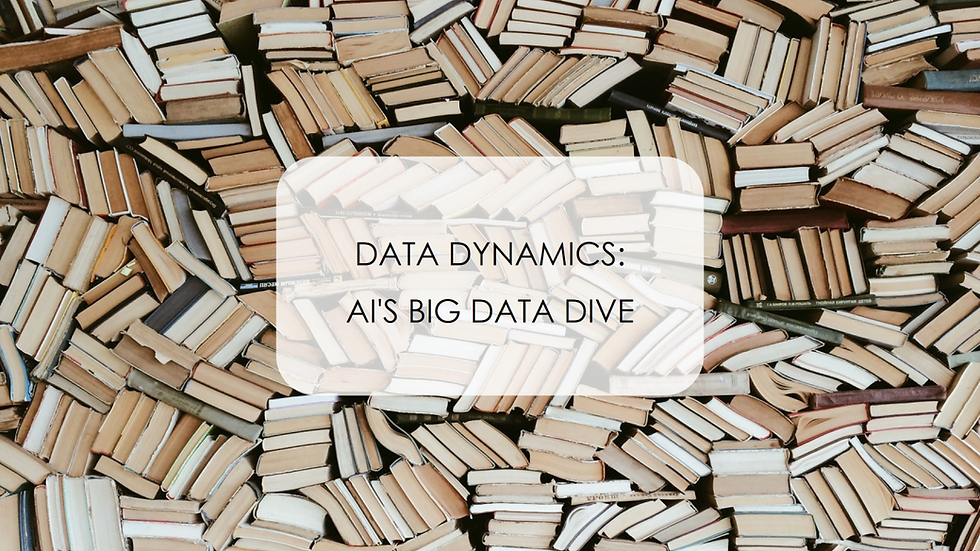
The realm of pharmacovigilance, dedicated to monitoring the safety of pharmaceuticals, is undergoing a transformation. The advent of big data and artificial intelligence (AI) is revolutionizing how drug safety information is collected, analyzed, and acted upon. This blog delves into the dynamic interplay between AI and big data in pharmacovigilance literature, highlighting the transformative impact on drug safety, the methodologies employed, the challenges faced, and the future directions of this burgeoning field.
The Role of Big Data in Pharmacovigilance:
Understanding Big Data
Big data refers to the vast volume, velocity, variety, and veracity of data generated from diverse sources, including electronic health records (EHRs), social media, clinical trials, adverse event reports, and scientific literature. In pharmacovigilance, big data encompasses all information pertinent to drug safety, offering a comprehensive view of a drug’s risk-benefit profile.
Importance of Big Data in Drug Safety
The integration of big data in pharmacovigilance offers several key advantages:
Comprehensive Insights: By aggregating data from multiple sources, big data provides a holistic understanding of drug safety.
Timely Signal Detection: Real-time data processing enables the early detection of adverse drug reactions (ADRs) and safety signals.
Enhanced Risk Assessment: Large datasets allow for more robust statistical analyses, improving the accuracy of risk assessments.
The Intersection of AI and Big Data in Pharmacovigilance:
AI's Role in Analyzing Big Data
Artificial intelligence, encompassing machine learning (ML), natural language processing (NLP), and deep learning, plays a pivotal role in managing and analyzing big data in pharmacovigilance. AI techniques can automatically process and interpret vast amounts of data, identifying patterns and anomalies that may indicate potential safety concerns.
Machine Learning
Machine learning algorithms, trained on historical pharmacovigilance data, can predict ADRs and identify safety signals with high accuracy. Techniques such as supervised learning, unsupervised learning, and reinforcement learning are employed to develop models that continuously improve as they are exposed to more data.
Applications in Pharmacovigilance:
Signal Detection: ML models can analyze EHRs, spontaneous reporting systems (SRS), and other data sources to identify new safety signals.
Risk Prediction: Predictive models assess the likelihood of ADRs based on patient characteristics and drug usage patterns.
Natural Language Processing
Natural language processing enables the extraction and analysis of unstructured data from scientific literature, social media, and other textual sources. NLP techniques can parse and interpret complex medical terminology, converting unstructured text into structured data for analysis.
Applications in Pharmacovigilance:
Literature Mining: NLP algorithms scan scientific publications and clinical trial reports to identify mentions of ADRs and other safety-related information.
Social Media Monitoring: By analyzing posts and comments on social media platforms, NLP tools can detect emerging safety concerns and patient experiences in real-time.
Deep Learning
Deep learning, a subset of machine learning, utilizes neural networks to process complex datasets. Deep learning models excel in recognizing intricate patterns and relationships within big data, making them particularly valuable for pharmacovigilance.
Applications in Pharmacovigilance:
Image and Signal Analysis: Deep learning algorithms can analyze medical images and signals for indications of ADRs.
Complex Data Integration: These models can integrate data from multiple sources, providing a comprehensive analysis of drug safety.
Methodologies and Tools for AI-Driven Pharmacovigilance:
Data Collection and Integration
Effective pharmacovigilance relies on the integration of diverse data sources. AI tools facilitate the aggregation and harmonization of data from EHRs, clinical trials, spontaneous reporting systems, and social media.
Key Tools:
Data Lakes: Centralized repositories that store structured and unstructured data, allowing for efficient data access and analysis.
Interoperability Standards: Protocols such as HL7 and FHIR ensure consistent data formats, enabling seamless data integration.
Data Preprocessing
Before analysis, big data must undergo preprocessing to ensure quality and consistency. AI techniques automate the cleaning, normalization, and transformation of raw data, preparing it for subsequent analysis.
Preprocessing Steps:
Data Cleaning: Identifying and correcting errors and inconsistencies in the data.
Data Normalization: Standardizing data formats and units to ensure comparability.
Feature Engineering: Creating new features or variables that enhance the predictive power of AI models.
Signal Detection and Analysis
AI-driven signal detection involves identifying patterns and anomalies in pharmacovigilance data that may indicate potential safety concerns. Advanced statistical methods and machine learning algorithms are used to detect and assess these signals.
Key Techniques:
Disproportionality Analysis: Statistical methods, such as the Bayesian Confidence Propagation Neural Network (BCPNN), identify signals by comparing the observed and expected frequencies of ADRs.
Pattern Recognition: ML algorithms detect patterns in the data that suggest new or emerging safety issues.
Visualization and Reporting
Effective communication of pharmacovigilance findings is crucial for regulatory decision-making and public health. AI tools enhance data visualization and reporting, making complex data accessible and understandable.
Key Tools:
Dashboards: Interactive dashboards provide real-time visualizations of pharmacovigilance data, facilitating quick and informed decision-making.
Automated Reporting: AI systems generate automated reports, summarizing key findings and insights for regulatory authorities and stakeholders.
Challenges in AI-Driven Pharmacovigilance:
Data Quality and Standardization
The effectiveness of AI in pharmacovigilance depends on the quality and consistency of the data. Variability in data sources, formats, and reporting practices can hinder the performance of AI models.
Solutions:
Data Governance: Implementing robust data governance frameworks to ensure data quality and consistency.
Standardization Initiatives: Supporting initiatives to standardize pharmacovigilance data formats and terminologies.
Algorithm Transparency and Interpretability:
AI algorithms, particularly deep learning models, can be complex and opaque, making it difficult to understand how decisions are made. Ensuring transparency and interpretability is crucial for regulatory compliance and stakeholder trust.
Solutions:
Explainable AI (XAI): Developing techniques that provide insights into how AI models make decisions, enhancing transparency.
Model Documentation: Comprehensive documentation of AI models, including their development, validation, and performance metrics.
Ethical and Privacy Concerns:
The use of AI in pharmacovigilance raises ethical and privacy concerns, particularly regarding the handling of sensitive patient data.
Solutions:
Data Anonymization: Implementing techniques to anonymize patient data, protecting privacy while maintaining data utility.
Ethical Guidelines: Establishing ethical guidelines for the use of AI in pharmacovigilance, ensuring responsible and fair practices.
Regulatory Acceptance
Gaining regulatory acceptance for AI-driven pharmacovigilance methods can be challenging, given the stringent requirements for drug safety monitoring.
Solutions:
Regulatory Collaboration: Engaging with regulatory authorities to develop guidelines and standards for AI in pharmacovigilance.
Validation Studies: Conducting rigorous validation studies to demonstrate the reliability and accuracy of AI methods.
Future Directions in AI-Driven Pharmacovigilance:
Integration of Real-World Data
The integration of real-world data (RWD) from sources such as EHRs, patient registries, and wearable devices offers new opportunities for enhancing pharmacovigilance. AI can analyze RWD to provide a more comprehensive understanding of drug safety in real-world settings.
Advancements in AI and ML
Ongoing advancements in AI and machine learning will continue to enhance the capabilities of pharmacovigilance systems. Innovations such as transfer learning and federated learning hold promise for improving the accuracy and adaptability of AI models.
Global Collaboration
Global collaboration among regulatory authorities, pharmaceutical companies, healthcare providers, and technology vendors will drive the development and adoption of AI-driven pharmacovigilance methods. Knowledge sharing and collaborative research projects will promote best practices and innovation.
Patient-Centered Approaches
Empowering patients to participate in pharmacovigilance through digital health technologies and patient-reported outcomes will enhance the accuracy and relevance of safety data. Patient-centered approaches ensure that the perspectives and experiences of patients are integrated into safety assessments.
Conclusion:
AI and big data are transforming pharmacovigilance literature, offering unprecedented opportunities to enhance drug safety. By leveraging advanced technologies such as machine learning, natural language processing, and deep learning, pharmacovigilance professionals can analyze vast amounts of data with greater efficiency and accuracy. While challenges remain, ongoing advancements and collaborative efforts will continue to drive innovation and excellence in pharmacovigilance, ultimately ensuring better patient safety and public health outcomes. As the field continues to evolve, the dynamic interplay between AI and big data will shape the future of drug safety monitoring.
Comments