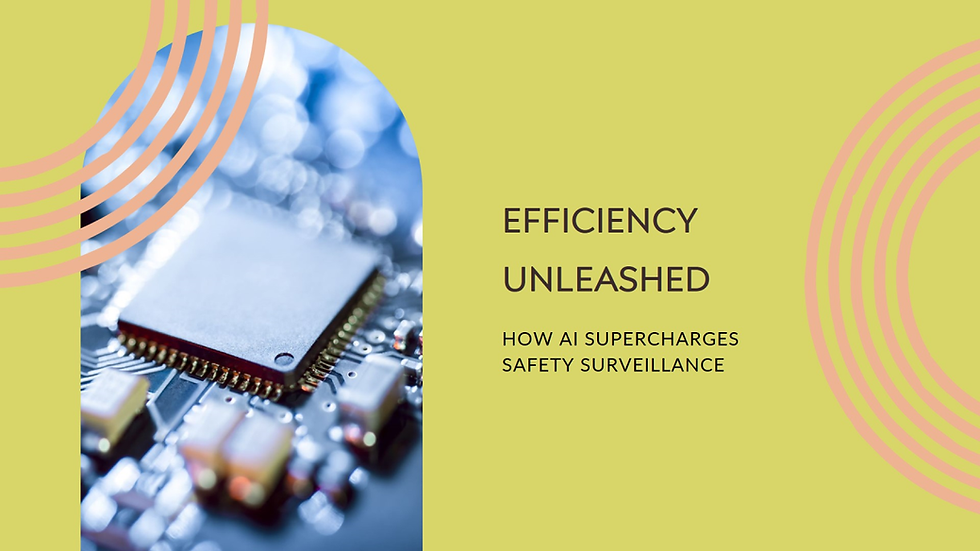
In the realm of pharmacovigilance, the rapid and accurate detection of adverse drug reactions (ADRs) is paramount. With the growing volume of healthcare data, traditional methods of safety surveillance struggle to keep pace. Enter artificial intelligence (AI), a transformative force revolutionizing the pharmacovigilance landscape. This blog explores how AI enhances safety surveillance, empowering stakeholders to detect, assess, and mitigate drug-related risks with unprecedented efficiency and precision.
The Evolution of Safety Surveillance:
Traditional Methods
Historically, safety surveillance relied on manual review of adverse event reports, clinical trial data, and medical literature. While effective, these methods were time-consuming, resource-intensive, and prone to human error. Moreover, they often struggled to detect rare or unexpected ADRs hidden within vast datasets.
The AI Revolution
Artificial intelligence, particularly machine learning (ML) and natural language processing (NLP), offers a game-changing solution to the limitations of traditional safety surveillance methods. By automating data analysis, AI accelerates the identification of safety signals, enhances data quality, and enables real-time monitoring of drug safety.
How AI Supercharges Safety Surveillance:
Rapid Signal Detection
AI algorithms can analyze large volumes of structured and unstructured data with unparalleled speed and accuracy. By scanning electronic health records, social media posts, and other sources, AI swiftly identifies potential safety signals, allowing stakeholders to take proactive measures to protect patient health.
Enhanced Data Analysis
ML algorithms excel at identifying patterns and trends within complex datasets. By analyzing disparate sources of safety data, including electronic medical records, claims databases, and wearable devices, AI uncovers hidden correlations and provides valuable insights into drug safety profiles.
Real-Time Monitoring
One of AI's most significant advantages is its ability to monitor drug safety in real-time. By continuously analyzing incoming data streams, AI can detect emerging safety concerns as they occur, enabling rapid response and intervention. This real-time monitoring capability is essential for safeguarding patient health in an increasingly dynamic healthcare landscape.
Applications of AI in Safety Surveillance:
Pharmacovigilance Systems
Pharmaceutical companies and regulatory agencies are increasingly incorporating AI into their pharmacovigilance systems. AI-powered tools automate adverse event reporting, signal detection, and risk assessment, streamlining the entire safety surveillance process and improving efficiency.
Social Media Monitoring
AI algorithms can analyze social media posts, patient forums, and online healthcare communities to identify potential safety concerns related to medications. By monitoring real-time conversations, AI provides valuable insights into patient experiences and perceptions of drug safety.
Wearable Devices
The proliferation of wearable devices, such as fitness trackers and smartwatches, offers new opportunities for AI-powered safety surveillance. By analyzing data collected from these devices, AI can detect changes in patient health metrics and identify potential ADRs in real-time.
Case Studies: AI in Action:
FDA's Sentinel Initiative
The U.S. Food and Drug Administration (FDA) operates the Sentinel Initiative, a national electronic system for monitoring the safety of FDA-regulated medical products. AI algorithms analyze electronic health records and insurance claims data to detect safety signals and inform regulatory decisions.
IBM Watson for Drug Safety
IBM Watson, a leading AI platform, offers a suite of tools for pharmacovigilance and safety surveillance. Watson's NLP capabilities enable it to analyze unstructured data from medical literature, clinical trial reports, and adverse event databases, empowering stakeholders to make informed decisions about drug safety.
AstraZeneca's AI-Driven Safety Surveillance
AstraZeneca, a global biopharmaceutical company, has implemented AI-driven tools for safety surveillance. By leveraging machine learning algorithms, AstraZeneca analyzes real-world data to identify potential safety signals and inform drug development and regulatory decisions.
Challenges and Considerations:
Data Quality and Bias
AI algorithms are only as good as the data they're trained on. Ensuring the quality and representativeness of training data is essential to avoid bias and inaccuracies in AI-powered safety surveillance systems.
Regulatory Compliance
Regulatory agencies must establish guidelines and standards for the use of AI in safety surveillance. Ensuring transparency, accountability, and compliance with regulatory requirements is essential to foster trust and confidence in AI-powered pharmacovigilance systems.
Ethical Considerations
AI raises important ethical considerations, particularly regarding patient privacy, consent, and autonomy. Safeguarding patient rights and ensuring ethical use of AI algorithms are paramount to maintaining public trust in pharmacovigilance practices.
The Future of AI in Safety Surveillance:
Integration with Real-World Data
The integration of AI with real-world data sources, including electronic health records, claims databases, and wearable devices, will further enhance the accuracy and effectiveness of safety surveillance efforts.
Personalized Safety Monitoring
AI has the potential to enable personalized safety monitoring, where safety assessments are tailored to individual patient characteristics and medical histories. This personalized approach will enhance the precision of safety surveillance and improve patient outcomes.
Collaboration and Knowledge Sharing
Collaboration between stakeholders, including pharmaceutical companies, regulatory agencies, healthcare providers, and technology vendors, will be essential for advancing the use of AI in safety surveillance. Knowledge sharing, data exchange, and best practice sharing will accelerate progress and drive innovation in pharmacovigilance.
Conclusion:
Artificial intelligence is revolutionizing safety surveillance, unlocking new possibilities for detecting, assessing, and mitigating drug-related risks. By harnessing the power of AI algorithms, stakeholders can enhance the efficiency, accuracy, and timeliness of safety surveillance efforts, ultimately improving patient outcomes and advancing public health.
As AI continues to evolve and integrate with pharmacovigilance systems, the future of safety surveillance looks promising. By embracing AI-powered technologies, stakeholders can stay ahead of emerging safety concerns, respond proactively to patient needs, and ensure the safe and effective use of medications for all.
Comments