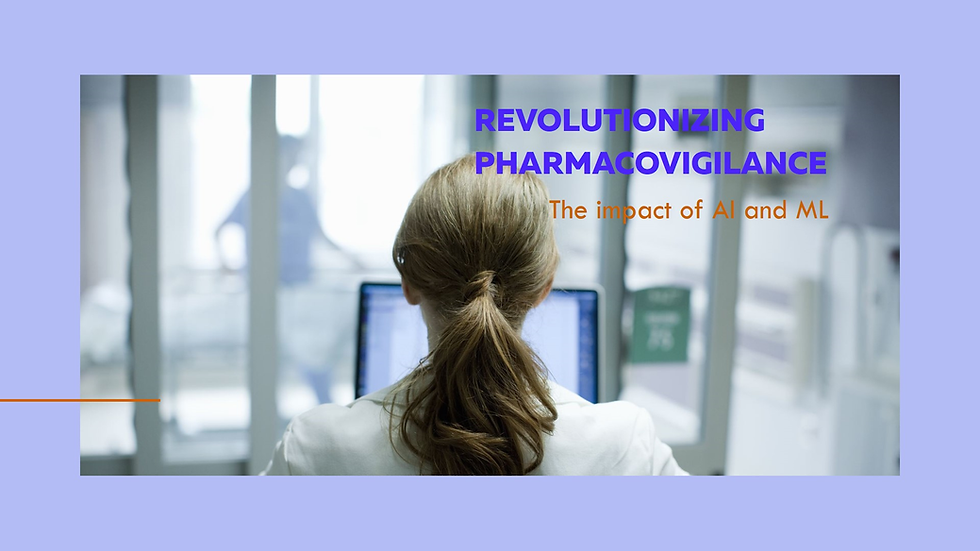
Pharmacovigilance (PV) is the science dedicated to the detection, assessment, understanding, and prevention of adverse effects related to pharmaceutical products. Traditionally, pharmacovigilance has relied on manual processes and static systems for monitoring drug safety. However, the integration of Artificial Intelligence (AI) and Machine Learning (ML) into pharmacovigilance systems is revolutionizing how adverse drug reactions (ADRs) are detected, analyzed, and managed. This blog explores how AI and ML are evolving pharmacovigilance systems, their impact on drug safety, and the challenges and opportunities associated with these technological advancements.
1. The Role of AI and ML in Pharmacovigilance
AI and ML are subsets of technology designed to enhance the ability of systems to learn from data and make predictions or decisions without explicit programming. In pharmacovigilance, AI and ML are being utilized to:
Automate Data Processing: AI and ML algorithms can process large volumes of data faster and more accurately than traditional methods. This automation helps streamline the collection, sorting, and analysis of adverse event reports.
Enhance Signal Detection: AI and ML techniques improve the ability to detect and analyze potential safety signals from vast amounts of data, including electronic health records, social media, and clinical trial results.
Predict and Prevent Adverse Events: Machine learning models can predict potential adverse events by analyzing patterns in historical data, enabling proactive risk management and preventive measures.
Improve Case Management: AI can assist in the management of individual adverse event cases, including data extraction, case narrative writing, and regulatory reporting.
2. Automating Data Processing
The sheer volume of data generated in pharmacovigilance, including reports from healthcare professionals, patients, and clinical trials, can overwhelm traditional systems. AI and ML offer significant improvements in automating data processing:
Natural Language Processing (NLP): NLP, a branch of AI, is used to extract relevant information from unstructured data sources such as free-text reports, social media posts, and medical literature. NLP algorithms can identify and categorize adverse events, extract patient demographics, and summarize case details, significantly reducing manual effort and improving accuracy.
Data Integration: AI systems can integrate data from various sources, including electronic health records (EHRs), patient registries, and clinical trial databases. This holistic view of data enhances the ability to identify trends and correlations that might indicate potential safety issues.
Case Triage and Prioritization: Machine learning algorithms can prioritize cases based on their severity and potential impact. By analyzing historical data, these algorithms can flag high-priority cases for immediate review, ensuring that critical safety concerns are addressed promptly.
3. Enhancing Signal Detection
Signal detection is a critical component of pharmacovigilance, involving the identification of new or previously unrecognized safety signals. AI and ML enhance this process through:
Advanced Analytics: Machine learning algorithms analyze large datasets to identify patterns and trends that may indicate potential safety signals. These algorithms can detect subtle correlations and interactions that might be missed by traditional methods.
Predictive Modeling: Predictive models use historical data to forecast potential adverse events and safety signals. By analyzing past incidents and patient outcomes, these models can provide early warnings of emerging safety issues.
Real-Time Monitoring: AI systems enable real-time monitoring of adverse events by continuously analyzing data from various sources, including social media and patient feedback. This allows for quicker detection and response to potential safety concerns.
4. Predicting and Preventing Adverse Events
One of the most promising applications of AI and ML in pharmacovigilance is the ability to predict and prevent adverse events:
Risk Prediction Models: Machine learning models can predict the likelihood of adverse events based on patient demographics, medical history, and treatment regimens. These models help identify patients who may be at higher risk of experiencing adverse reactions, allowing for targeted monitoring and preventive measures.
Personalized Medicine: AI-driven insights can support personalized medicine by tailoring drug therapies to individual patient profiles. By analyzing genetic, clinical, and lifestyle data, AI systems can recommend personalized treatment plans that minimize the risk of adverse events.
Drug Interaction Analysis: AI and ML algorithms can analyze potential drug interactions by assessing data from clinical trials, EHRs, and literature. Identifying risky interactions in advance can help prevent adverse reactions and improve patient safety.
5. Improving Case Management
AI and ML also offer significant benefits in case management, making the process of handling adverse event reports more efficient:
Automated Data Extraction: AI systems can automatically extract relevant information from adverse event reports, including patient demographics, drug details, and event descriptions. This reduces the manual effort required for data entry and improves the consistency and accuracy of case data.
Narrative Generation: Machine learning algorithms can assist in generating case narratives, summarizing the details of adverse events in a structured and coherent manner. This supports regulatory reporting requirements and enhances the clarity of safety information.
Regulatory Compliance: AI systems can ensure compliance with regulatory requirements by automatically generating and submitting reports to regulatory authorities. These systems can also track submission deadlines and ensure that all required information is included in the reports.
6. Challenges and Opportunities
While AI and ML offer numerous benefits for pharmacovigilance, they also present challenges that need to be addressed:
Data Quality and Availability: The effectiveness of AI and ML algorithms depends on the quality and completeness of the data used for training and analysis. Inaccurate or incomplete data can lead to biased or incorrect predictions. Ensuring high-quality data sources is crucial for the success of AI-driven pharmacovigilance systems.
Regulatory and Ethical Considerations: The use of AI and ML in pharmacovigilance must comply with regulatory requirements and ethical standards. Ensuring transparency, fairness, and accountability in the development and deployment of AI systems is essential to maintain trust and ensure that patient safety is prioritized.
Integration with Existing Systems: Integrating AI and ML technologies with existing pharmacovigilance systems can be complex and resource-intensive. Organizations need to ensure that new technologies are compatible with their current infrastructure and workflows.
Data Security and Privacy: The use of AI and ML involves handling large volumes of sensitive patient data. Ensuring data security and privacy is critical to protect patient information and comply with regulations such as GDPR and HIPAA.
Despite these challenges, the opportunities offered by AI and ML are significant. The ability to process and analyze large volumes of data, detect emerging safety signals, and predict adverse events has the potential to greatly enhance pharmacovigilance practices and improve patient safety.
7. Future Directions in AI and ML for Pharmacovigilance
The future of pharmacovigilance will likely be shaped by ongoing advancements in AI and ML technologies:
Continued Innovation: Ongoing research and development in AI and ML will lead to more sophisticated algorithms and tools for pharmacovigilance. Innovations such as deep learning and reinforcement learning have the potential to further enhance the accuracy and efficiency of safety monitoring.
Integration with Other Technologies: The integration of AI and ML with other technologies, such as blockchain and IoT, could offer new solutions for data security, traceability, and real-time monitoring in pharmacovigilance.
Personalized Approaches: As AI and ML technologies continue to evolve, they will support more personalized approaches to pharmacovigilance. Tailoring safety monitoring and risk management strategies to individual patient profiles will become increasingly feasible, improving the overall effectiveness of drug safety practices.
Global Collaboration: Collaboration between pharmaceutical companies, regulatory authorities, and technology providers will be essential for maximizing the benefits of AI and ML in pharmacovigilance. Sharing data, insights, and best practices will help advance the field and address common challenges.
Conclusion:
AI and ML are transforming pharmacovigilance systems, offering new opportunities to enhance drug safety and improve patient care. By automating data processing, enhancing signal detection, predicting and preventing adverse events, and improving case management, these technologies are revolutionizing how adverse drug reactions are monitored and managed.
While challenges remain, such as ensuring data quality, addressing regulatory and ethical considerations, and integrating new technologies with existing systems, the potential benefits of AI and ML are substantial. As the field of pharmacovigilance continues to evolve, ongoing innovation and collaboration will be key to harnessing the full potential of these technologies and ensuring the highest standards of drug safety.
Comments