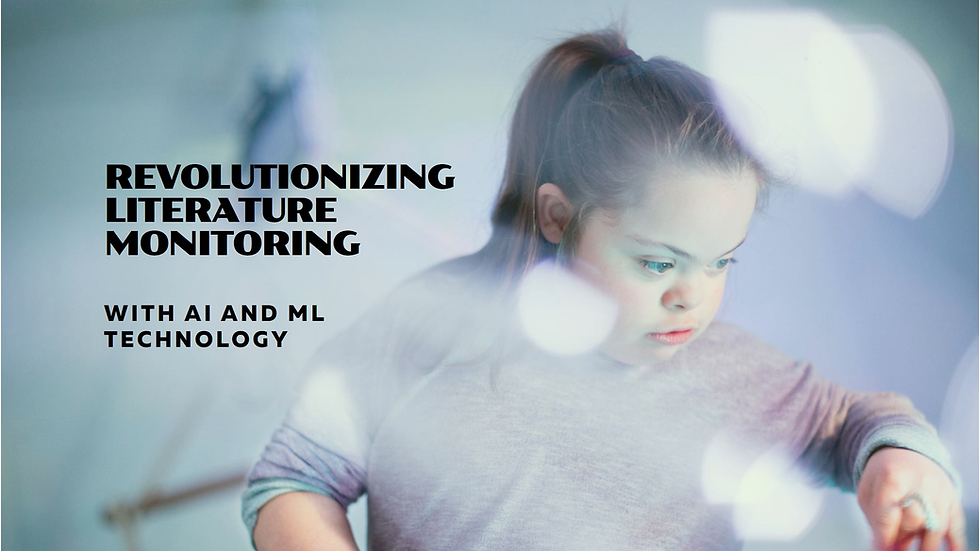
In the realm of research and development, particularly within fields like pharmaceuticals, healthcare, and academia, literature monitoring is a crucial task. It involves keeping track of the latest studies, findings, and trends to stay informed and make evidence-based decisions. However, manually sifting through vast amounts of literature can be time-consuming and prone to error. This is where Artificial Intelligence (AI) and Machine Learning (ML) come into play, offering transformative solutions to enhance literature monitoring efficiency. In this blog, we’ll explore how AI and ML are revolutionizing literature monitoring, discussing their benefits, applications, and future potential.
Understanding Literature Monitoring:
Literature monitoring involves systematically reviewing and analyzing new publications and research to stay updated with the latest developments in a field. This process typically includes:
Searching for Relevant Literature: Identifying and retrieving articles, papers, and reports related to specific research interests or questions.
Screening and Filtering: Evaluating the relevance and quality of the retrieved literature to select the most pertinent studies.
Data Extraction: Extracting key information from selected studies, such as results, methodologies, and conclusions.
Synthesis and Analysis: Synthesizing findings from multiple studies to identify trends, gaps, and insights.
Reporting and Documentation: Documenting the findings and updates for future reference or decision-making.
Given the exponential growth in research publications, manual literature monitoring is becoming increasingly impractical. AI and ML offer innovative approaches to streamline and enhance this process.
The Role of AI and ML in Literature Monitoring:
Automated Literature Search
AI and ML can significantly enhance the efficiency of literature searches by automating and optimizing the retrieval of relevant documents.
Natural Language Processing (NLP): NLP algorithms enable AI systems to understand and process search queries written in natural language. This capability allows for more accurate and contextually relevant search results. For example, NLP can interpret complex queries and identify relevant studies even if they use different terminology or phrasing.
Semantic Search: Unlike traditional keyword-based searches, semantic search uses AI to understand the meaning and context of search terms. This approach helps in retrieving relevant literature that might not be captured through simple keyword matching.
Content-Based Filtering: ML algorithms can analyze the content of research articles and filter out irrelevant studies based on their similarity to the research query. This content-based filtering improves the precision of search results.
Efficient Screening and Filtering
Screening and filtering literature is a critical step in monitoring, and AI and ML can make this process more efficient.
Machine Learning Classification: ML models can be trained to classify articles based on relevance, quality, and other criteria. These models can quickly review abstracts and full texts, flagging potentially relevant studies for further examination.
Automated Abstract Screening: AI tools can automatically screen abstracts and titles to identify studies that meet predefined inclusion and exclusion criteria. This automation reduces the manual effort required and speeds up the screening process.
Duplicate Detection: AI can identify duplicate studies and eliminate redundancy, ensuring that each article is unique and contributes new insights to the review. This process helps in maintaining the quality and accuracy of the literature monitoring.
Data Extraction and Analysis
Extracting and analyzing data from research articles is a time-consuming task that can be enhanced with AI and ML.
Automated Data Extraction: AI tools can automatically extract key data points, such as study results, methodologies, and sample sizes, from research articles. This automation reduces manual effort and minimizes errors in data extraction.
Text Mining and Data Extraction: AI-powered text mining techniques can identify and extract relevant information from large volumes of text. This approach helps in uncovering patterns, trends, and key findings across multiple studies.
Sentiment and Trend Analysis: AI can perform sentiment analysis on research findings to gauge the overall tone and implications of the studies. Additionally, trend analysis can identify emerging research areas and shifts in focus over time.
Enhanced Synthesis and Reporting
Synthesizing findings and generating reports are critical aspects of literature monitoring. AI and ML can enhance these processes by:
Automated Summarization: AI can generate concise summaries of individual studies and the overall review. These summaries help in quickly grasping the main findings and conclusions without reading every article in detail.
Data Visualization: AI can create visualizations, such as charts, graphs, and network diagrams, to represent complex data and relationships. These visualizations help in understanding trends, gaps, and correlations across studies.
Knowledge Graphs: AI can construct knowledge graphs to map relationships between different research topics, authors, and studies.
These graphs provide a comprehensive view of the research landscape and facilitate the identification of key trends and connections.
Real-Time Updates and Alerts
Staying updated with the latest research is crucial for effective literature monitoring. AI can provide real-time updates and alerts to keep researchers informed.
Automated Alerts: AI systems can monitor new publications and generate alerts based on predefined criteria. This feature ensures that researchers are notified of relevant studies as soon as they are published.
Customizable Notification Systems: AI can tailor notifications based on individual preferences and research interests, ensuring that alerts are relevant and actionable.
Improving Review Quality
AI and ML contribute to the quality and rigor of literature reviews by addressing potential biases and ensuring comprehensive coverage.
Bias Detection: AI tools can identify potential biases in the selection and analysis of studies, such as publication bias or methodological flaws. This detection ensures a more objective and comprehensive review.
Quality Assessment: AI can assess the quality of studies based on predefined criteria, such as study design, sample size, and methodological rigor. This assessment helps in including only high-quality studies in the review.
Gap Identification: AI can analyze the literature to identify research gaps and areas where further investigation is needed. This insight helps researchers focus on underexplored topics and contribute to advancing knowledge.
Enhancing Collaboration
Collaboration is an essential aspect of literature monitoring, and AI can facilitate this by:
Collaborative Platforms: AI-powered collaborative platforms enable researchers to share and review literature in real time. These platforms support annotations, discussions, and joint analysis, improving the efficiency of the monitoring process.
Knowledge Sharing: AI tools can aggregate and share insights from literature monitoring across different research groups and institutions, promoting knowledge sharing and collaboration.
Examples of AI and ML Tools for Literature Monitoring:
Several AI and ML tools are designed to enhance literature monitoring efficiency:
Semantic Scholar: This AI-powered search engine uses NLP and machine learning to provide relevant search results, citation graphs, and key paper recommendations.
Scraper and Zotero: Zotero, along with web scraping tools, can automate the collection and organization of literature, integrating AI for better management and retrieval.
Rayyan: Rayyan uses AI to assist in the systematic review process, including screening and selection of studies. It offers collaborative features and automated classification.
EndNote: EndNote integrates AI-driven features for organizing and annotating literature, as well as generating bibliographies.
IBM Watson Discovery: This AI platform offers advanced text mining and data analysis capabilities, helping researchers uncover insights from large volumes of text.
Benefits of AI and ML in Literature Monitoring:
Increased Efficiency: AI and ML automate time-consuming tasks, such as literature search, screening, and data extraction, significantly reducing the manual effort required and speeding up the process.
Enhanced Accuracy: AI algorithms can improve the accuracy of literature monitoring by minimizing human errors and biases in data retrieval and analysis.
Comprehensive Coverage: AI tools can analyze vast amounts of literature and identify relevant studies that might be missed through traditional methods, ensuring comprehensive coverage of the research landscape.
Real-Time Updates: AI provides real-time updates and alerts, keeping researchers informed of the latest developments and publications in their field.
Improved Data Analysis: AI and ML enable advanced data analysis, including trend identification, sentiment analysis, and knowledge graph construction, providing deeper insights into the research landscape.
Enhanced Collaboration: AI-powered collaborative platforms facilitate real-time sharing and discussion of literature, promoting effective teamwork and knowledge sharing.
Challenges and Considerations:
While AI and ML offer significant advantages, there are challenges and considerations to keep in mind:
Data Quality: The effectiveness of AI and ML depends on the quality and completeness of the input data. Ensuring high-quality data and well-defined criteria is essential for accurate results.
Algorithm Bias: AI algorithms can inherit biases from training data or design. Researchers should be aware of potential biases and validate AI results to ensure fairness and accuracy.
Integration with Existing Processes: Integrating AI tools with existing literature monitoring processes and workflows may require adjustments and training. Ensuring smooth integration is crucial for maximizing AI benefits.
Ethical Considerations: The use of AI in literature monitoring raises ethical considerations, such as data privacy and intellectual property. Researchers should adhere to ethical guidelines and practices when using AI tools.
User Training: Effective use of AI tools requires training and familiarization with the technology. Ensuring that researchers are adequately trained is essential for successful implementation.
The Future of AI and ML in Literature Monitoring:
As AI and ML technology continues to advance, their role in literature monitoring will expand and evolve. Future developments may include:
Enhanced NLP Capabilities: Improved NLP algorithms will enable more accurate interpretation of complex research queries and nuanced content.
Greater Integration: AI tools will become more seamlessly integrated with research databases, reference management systems, and collaborative platforms.
Advanced Predictive Analytics: AI may offer predictive analytics to forecast research trends and identify emerging topics before they become prominent.
Improved Personalization: AI will provide more personalized recommendations and insights based on individual research preferences and objectives.
Augmented Reality Integration: AI and ML may integrate with augmented reality (AR) technologies to create immersive literature monitoring experiences and visualizations.
Conclusion:
AI and ML are transforming literature monitoring by enhancing efficiency, accuracy, and depth of analysis. By automating time-consuming tasks, providing real-time updates, and offering advanced data analysis capabilities, AI and ML are revolutionizing how researchers track and analyze the latest developments in their fields.
As these technologies continue to evolve, their integration into literature monitoring will become increasingly sophisticated, offering new tools and techniques to support researchers in navigating the vast and growing body of scientific knowledge. Embracing AI and ML in literature monitoring not only streamlines the research process but also contributes to the advancement of knowledge and innovation across diverse fields.
Comments