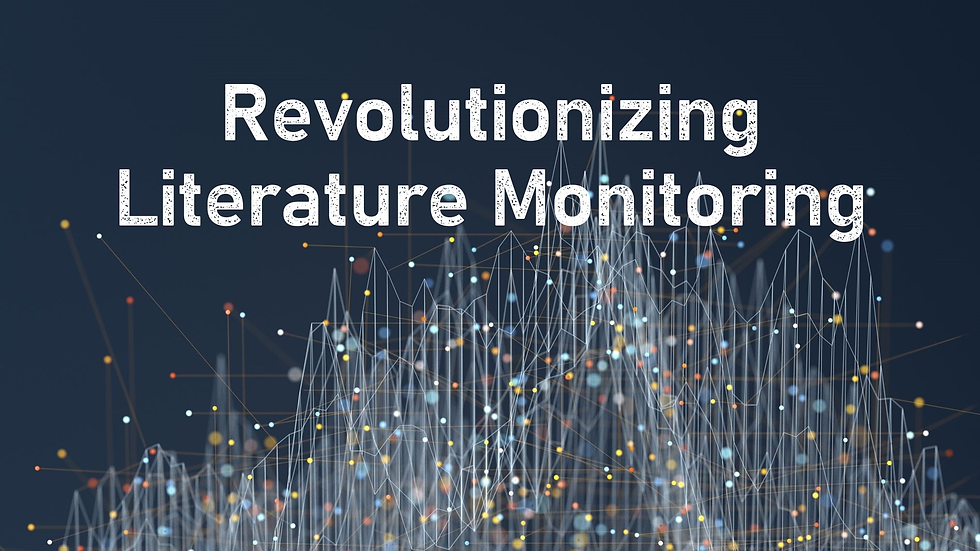
In the modern research landscape, staying up-to-date with the latest scientific literature has become a daunting task. The sheer volume of publications across various fields, especially in domains such as healthcare, pharmacovigilance, and medical device safety, makes it nearly impossible for researchers to manually monitor and analyze every relevant piece of literature. Literature monitoring, a critical aspect of research and regulatory processes, ensures that up-to-date knowledge is applied to decision-making, risk assessment, and compliance. However, without proper tools, literature monitoring can be both time-consuming and prone to errors.
Enter artificial intelligence (AI) and machine learning (ML). These technologies are transforming the way literature monitoring is conducted, offering automated solutions that not only enhance efficiency but also improve the quality of insights drawn from massive datasets. AI and ML can sift through thousands of documents in a fraction of the time it would take a human, while minimizing the risk of missing key studies or relevant updates. This blog will explore how AI and ML can significantly enhance literature monitoring efficiency, particularly in research, healthcare, and regulatory environments.
1. Understanding the Complexity of Literature Monitoring
Literature monitoring involves regularly scanning, retrieving, and analyzing a wide range of academic and scientific publications, clinical studies, patents, regulatory documents, and other forms of relevant data. It is particularly important in highly regulated fields such as pharmacovigilance, where accurate monitoring of adverse drug events and other safety concerns is critical for patient safety and compliance.
Traditional literature monitoring is often a manual process, involving the following steps:
Search and Retrieval: Searching databases, journals, and other repositories for relevant articles using keywords or other search criteria.
Screening and Selection: Reviewing the search results to filter out irrelevant studies and focus on those that meet specific inclusion criteria.
Data Extraction: Extracting key information, such as study outcomes, methodologies, and conclusions, for analysis.
Analysis and Reporting: Synthesizing the extracted data and generating reports or summaries for regulatory submissions or internal review.
The sheer volume of information published on a daily basis, coupled with the manual nature of these tasks, makes literature monitoring a highly labor-intensive process. Moreover, human error, bias, and inconsistencies in searching, screening, and analyzing data can lead to incomplete or inaccurate reviews.
2. AI and ML: A Game-Changer for Literature Monitoring
Artificial intelligence and machine learning technologies are revolutionizing the literature monitoring process by automating repetitive tasks and enabling more comprehensive and efficient analysis of large volumes of data. Here’s how these technologies can optimize the various stages of literature monitoring.
2.1. Automated Search and Retrieval
One of the most time-consuming tasks in literature monitoring is searching for relevant studies across multiple databases and sources. Traditional keyword-based searches often return irrelevant or incomplete results, requiring manual effort to sift through.
AI and ML-powered search engines, on the other hand, can perform more sophisticated searches by understanding the context behind search queries, using Natural Language Processing (NLP). NLP algorithms can interpret complex scientific terminology and concepts, delivering more accurate search results. Additionally, AI systems can continuously scan the latest publications and automatically retrieve relevant studies in real-time.
For example, in pharmacovigilance, AI-powered search engines can automatically identify new publications related to drug safety or adverse drug reactions, providing real-time alerts to researchers and regulatory bodies. This real-time monitoring ensures that no critical information is missed.
2.2. Improved Screening and Selection
Screening search results for relevance is another labor-intensive aspect of literature monitoring. In the manual process, researchers often have to read abstracts or even full texts to determine whether a study is relevant to their work. This is not only time-consuming but also increases the likelihood of missing important studies or introducing bias into the review process.
Machine learning models can be trained to automatically screen search results based on pre-defined inclusion and exclusion criteria. These models learn from past selections made by researchers, enabling them to improve over time and more accurately identify relevant studies. For example, if researchers are interested in clinical trials that test a specific drug in a particular population, ML algorithms can be trained to identify such studies based on parameters such as population demographics, drug dosage, and study outcomes.
By automating the screening process, AI and ML reduce human bias and error, ensuring that relevant studies are not overlooked. Moreover, automated screening enables researchers to process larger datasets more efficiently, allowing for more comprehensive literature monitoring.
2.3. Advanced Data Extraction and Text Mining
After screening and selecting relevant studies, the next step is to extract key information from those studies for further analysis. This process often involves manually identifying important data points, such as study methodologies, sample sizes, outcomes, and conclusions. AI and ML can automate this task through advanced data extraction and text mining capabilities.
NLP algorithms can automatically extract relevant information from research papers, summarizing key findings, and categorizing data points. For instance, AI-powered systems can read and extract data from clinical trial reports, identifying trends in efficacy, safety outcomes, and adverse reactions. This reduces the time and effort required for manual data extraction and ensures that the data is structured in a consistent and easily analyzable format.
Moreover, some AI tools can even generate visual representations of the extracted data, making it easier for researchers to identify trends and draw conclusions.
2.4. Streamlined Reporting and Compliance
In highly regulated fields such as pharmacovigilance, accurate literature monitoring is essential for meeting regulatory requirements and ensuring patient safety. AI and ML technologies can help streamline the reporting process by automatically generating reports based on extracted data and ensuring that all relevant studies are included.
For instance, AI-powered tools can automatically compile reports on adverse drug reactions or other safety concerns, including all relevant publications, clinical studies, and regulatory guidelines. These tools can also ensure that the reports are formatted according to regulatory standards, saving time and reducing the risk of non-compliance.
By automating the reporting process, AI and ML enable organizations to stay compliant with regulatory requirements while minimizing the time and effort required for manual reporting.
3. Benefits of AI and ML in Literature Monitoring
AI and ML offer numerous benefits when it comes to enhancing the efficiency of literature monitoring. Some of the most significant advantages include:
3.1. Time Efficiency
AI and ML-powered tools can process vast amounts of data in a fraction of the time it would take a human. Tasks such as searching, screening, and data extraction, which traditionally take weeks or months, can be completed in hours or days with AI. This allows researchers to stay up-to-date with the latest literature without being overwhelmed by the sheer volume of publications.
3.2. Increased Accuracy
AI and ML algorithms can analyze data with a high degree of accuracy, reducing the risk of human error and ensuring that no critical studies are missed. Moreover, these technologies can eliminate bias from the screening and selection process, ensuring a more objective review of the literature.
3.3. Scalability
AI and ML systems can scale to handle large datasets, making them particularly useful in fields such as pharmacovigilance and medical device safety, where thousands of publications must be monitored. As the volume of published research continues to grow, AI and ML will become increasingly essential for managing the scale of literature monitoring tasks.
3.4. Real-Time Updates
AI-powered tools can continuously scan for new publications and alert researchers in real-time when relevant studies are published. This ensures that literature reviews are always current and that critical information is not missed due to delays in manual monitoring.
3.5. Cost Savings
By automating repetitive tasks and reducing the time required for literature monitoring, AI and ML can significantly lower operational costs for organizations. Researchers can focus on higher-level analysis and interpretation, while AI handles the more labor-intensive aspects of monitoring.
4. Applications of AI and ML in Literature Monitoring
The use of AI and ML in literature monitoring is already transforming various industries, particularly in areas such as healthcare, drug safety, and regulatory compliance. Below are a few examples of how these technologies are being applied in specific fields:
4.1. Pharmacovigilance
In pharmacovigilance, AI and ML are used to monitor literature for reports of adverse drug reactions (ADRs) and other safety concerns. By automatically identifying relevant studies and extracting key data points, AI helps pharmacovigilance teams stay compliant with regulatory requirements and ensures that no critical safety information is missed.
4.2. Medical Device Safety
AI and ML tools are also being used in medical device safety monitoring to track new studies, clinical trials, and reports related to the safety and efficacy of medical devices. These technologies help ensure that safety concerns are identified early and that the relevant data is included in regulatory submissions.
4.3. Public Health Research
In public health research, AI and ML are being used to monitor literature for emerging trends, such as the spread of infectious diseases or the efficacy of public health interventions. By automating the monitoring process, public health organizations can respond more quickly to new findings and implement evidence-based interventions.
5. The Future of AI and ML in Literature Monitoring
As AI and ML technologies continue to advance, their role in literature monitoring will become even more prominent. Future developments may include even more sophisticated NLP algorithms capable of understanding complex scientific concepts and generating highly accurate summaries of research findings.
Moreover, the integration of AI and ML with other technologies, such as blockchain and big data analytics, could further enhance the transparency and efficiency of literature monitoring processes.
Conclusion
AI and ML are transforming literature monitoring by automating time-consuming tasks, improving accuracy, and enabling researchers to handle larger volumes of data with ease. These technologies are particularly valuable in regulated industries such as pharmacovigilance, where accurate and timely monitoring is critical for compliance and patient safety.
By embracing AI and ML, organizations can optimize their literature monitoring workflows, stay ahead of the latest
Comments