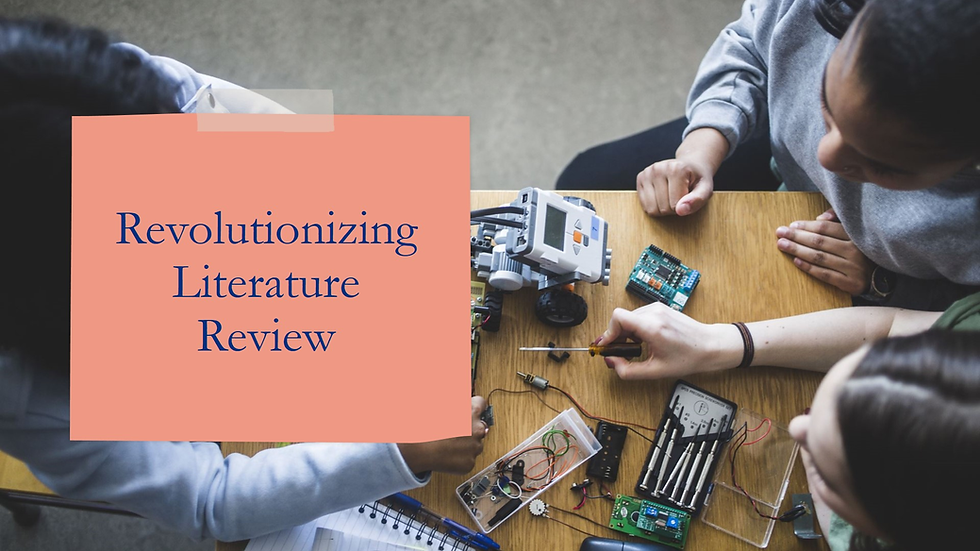
Pharmacovigilance, the science of monitoring the effects of drugs after they have been licensed for use, is critical for ensuring patient safety and efficacy. An essential component of pharmacovigilance is the literature review, which involves evaluating existing studies, reports, and publications to identify potential safety signals and adverse drug reactions (ADRs). Traditional methods of literature review are often labor-intensive and time-consuming, requiring significant human effort to sift through vast amounts of data. However, advancements in Artificial Intelligence (AI) and Machine Learning (ML) are transforming how literature reviews are conducted, offering new tools and methodologies that enhance efficiency, accuracy, and insight.
The Challenges of Traditional Literature Review:
Conducting a literature review in pharmacovigilance traditionally involves several challenges:
Volume of Data: The sheer volume of scientific literature is overwhelming. Thousands of new research articles, case reports, and reviews are published every year, making it difficult for human reviewers to keep up with the latest developments.
Relevance and Quality: Identifying relevant and high-quality studies from a vast array of sources requires expertise and can be prone to human error. Distinguishing between credible and non-credible sources adds another layer of complexity.
Data Extraction and Analysis: Extracting pertinent data from literature, such as study design, outcomes, and statistical significance, involves meticulous manual work. Analyzing and synthesizing this data to identify patterns and trends further complicates the process.
Bias and Subjectivity: Human reviewers are susceptible to biases and subjective judgments, which can affect the consistency and reliability of the review process.
AI and ML Are Transforming Literature Reviews:
AI and ML technologies are addressing these challenges by automating and enhancing various aspects of the literature review process. Here’s how:
Automated Data Extraction and Organization
AI-powered tools can automate the extraction of relevant data from scientific articles. Natural Language Processing (NLP), a subfield of AI, enables these tools to read and interpret text, identify key information, and categorize data efficiently. For example, AI algorithms can automatically extract data on drug names, adverse effects, study methodologies, and patient demographics from research articles.
Enhanced Search and Retrieval
Traditional search methods often rely on keyword-based queries, which can miss relevant studies or yield too many irrelevant results. AI-driven search engines and recommendation systems use advanced algorithms to understand the context and semantics of queries, improving search accuracy. Machine learning models can analyze historical search patterns and user interactions to suggest the most relevant articles and sources.
Bias Detection and Mitigation
ML algorithms can help identify and mitigate biases in literature reviews. For example, machine learning models can analyze publication trends and detect potential biases in study results, such as selective reporting or publication bias. This helps ensure a more balanced and objective review of the literature.
Predictive Analytics and Signal Detection
Predictive analytics, powered by machine learning, can identify potential safety signals and trends before they become apparent through traditional methods. By analyzing large datasets and identifying patterns, ML models can predict adverse drug reactions and other safety issues. This proactive approach allows for early intervention and better risk management.
Real-Time Updates and Monitoring
AI systems can continuously monitor and update literature databases, providing real-time access to new research and findings. This ensures that pharmacovigilance professionals have the most current information at their disposal, reducing the time lag between study publication and review.
Integration with Other Data Sources
AI and ML can integrate literature review findings with other data sources, such as electronic health records (EHRs), clinical trial databases, and social media platforms. This holistic approach provides a more comprehensive understanding of drug safety and efficacy, as well as emerging trends and potential safety signals.
Improved Data Visualization
Data visualization tools powered by AI and ML can present complex data in more accessible and interpretable formats. Interactive dashboards and visualizations help pharmacovigilance professionals quickly grasp key insights, trends, and correlations from the literature.
Case Studies and Applications:
IBM Watson for Drug Discovery
IBM Watson for Drug Discovery is a prime example of how AI is being used in pharmacovigilance. Watson uses NLP and machine learning to analyze vast amounts of scientific literature, clinical trial data, and patient records to identify potential drug candidates and safety concerns. This AI-powered platform has been instrumental in accelerating drug discovery and improving the efficiency of literature reviews.
Elsevier’s PharmaPendium
Elsevier’s PharmaPendium utilizes AI to streamline the literature review process for pharmacovigilance. The platform employs machine learning algorithms to analyze drug labels, clinical trial data, and adverse event reports, providing actionable insights and enhancing the accuracy of safety assessments.
FDA’s Sentinel Initiative
The FDA’s Sentinel Initiative leverages AI and machine learning to monitor and analyze drug safety data from various sources, including literature. By integrating literature review findings with electronic health records and other data sources, the initiative improves the detection and management of drug-related safety issues.
Future Directions and Considerations:
As AI and ML technologies continue to evolve, their impact on literature reviews in pharmacovigilance will likely grow. Future developments may include:
Advanced NLP Techniques: Continued advancements in NLP will improve the ability of AI systems to understand and interpret complex scientific language, further enhancing data extraction and analysis.
Greater Integration with Clinical Data: Increased integration of literature review findings with real-world clinical data will provide a more comprehensive view of drug safety and efficacy.
Ethical and Regulatory Considerations: As AI and ML play a larger role in pharmacovigilance, addressing ethical and regulatory considerations will be crucial. Ensuring transparency, accountability, and compliance with regulatory standards will be essential for maintaining trust and integrity in the review process.
Collaboration and Knowledge Sharing: Collaborative efforts between AI developers, pharmacovigilance professionals, and regulatory agencies will be vital for maximizing the benefits of AI and ML in literature reviews. Sharing knowledge and best practices will help drive innovation and improve outcomes.
Conclusion:
AI and ML are revolutionizing the literature review process in pharmacovigilance by addressing the limitations of traditional methods and providing new tools for data extraction, analysis, and signal detection. These technologies enhance efficiency, accuracy, and insight, enabling pharmacovigilance professionals to better safeguard patient health and manage drug safety. As AI and ML continue to advance, their role in literature reviews will become increasingly critical, shaping the future of pharmacovigilance and contributing to more effective and timely drug safety monitoring.
Comments