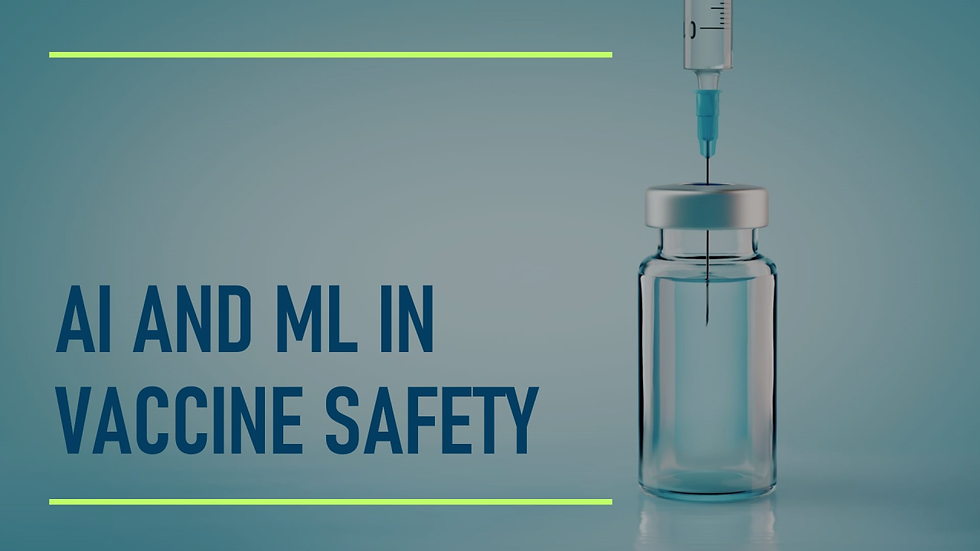
In the realm of public health, vaccine safety is paramount to ensure widespread immunization programs are effective and trustworthy. Advanced technologies like Artificial Intelligence (AI) and Machine Learning (ML) are transforming vaccine safety by revolutionizing safety profile analysis. This blog explores how AI and ML enhance safety profile analysis in vaccine safety, including their methodologies, benefits, challenges, real-world applications, and the future implications of these technologies.
Understanding Vaccine Safety Profile Analysis:
Vaccine safety profile analysis involves evaluating the safety and efficacy of vaccines through the systematic collection, monitoring, and analysis of data. This data includes adverse event reports, clinical trial results, post-marketing surveillance data, and electronic health records. The goal is to identify potential safety concerns, assess risks, and ensure the overall safety of vaccines before and after they are licensed for public use.
Role of AI and ML in Vaccine Safety:
AI and ML technologies play a transformative role in vaccine safety profile analysis by leveraging large datasets and advanced analytics techniques to:
1. Enhance Signal Detection
AI algorithms can sift through vast amounts of data to detect subtle patterns or correlations that may indicate potential safety signals. This proactive approach allows for early identification of adverse events, contributing to timely interventions and improved safety monitoring.
2. Improve Data Integration and Standardization
ML models can streamline the integration and standardization of heterogeneous data sources, such as adverse event reports from different healthcare systems or clinical trial data from various locations. This enhances data quality and consistency, facilitating more comprehensive safety analyses.
3. Facilitate Real-Time Monitoring
AI-powered systems enable real-time monitoring of vaccine safety by continuously analyzing incoming data from diverse sources. This capability is crucial for promptly identifying emerging safety concerns and ensuring rapid response measures.
4. Optimize Risk Prediction
ML algorithms can predict individual and population-level risks associated with vaccines based on demographic data, medical history, and previous adverse events. This personalized approach supports targeted interventions and enhances overall safety management.
Methodologies for AI and ML in Vaccine Safety:
The methodologies employed by AI and ML in vaccine safety profile analysis include:
- Natural Language Processing (NLP):
NLP techniques extract valuable insights from unstructured data sources such as patient reports and clinical notes. This helps in identifying and categorizing adverse events more accurately.
- Supervised Learning:
Supervised learning algorithms train on labeled datasets to classify adverse events, predict outcomes, and assess the likelihood of safety concerns associated with specific vaccines.
- Unsupervised Learning:
Unsupervised learning techniques, such as clustering and anomaly detection, identify patterns and outliers in vaccine safety data without predefined labels. This aids in discovering unexpected safety signals.
- Deep Learning:
Deep learning models, including Convolutional Neural Networks (CNNs) and Recurrent Neural Networks (RNNs), process complex data structures and sequences, improving the accuracy of safety profile analysis.
Benefits of AI and ML in Vaccine Safety:
Implementing AI and ML in vaccine safety profile analysis offers several benefits:
- Enhanced Efficiency:
Automating data analysis tasks reduces manual effort and speeds up the detection of safety signals, enabling quicker responses and interventions.
- Improved Accuracy:
AI and ML algorithms can analyze large volumes of data with high accuracy, minimizing human error and ensuring robust safety assessments.
- Real-Time Insights:
Continuous monitoring and real-time analytics provide immediate insights into vaccine safety, supporting timely decision-making and regulatory actions.
- Personalized Medicine:
ML-driven risk prediction models enable personalized safety assessments, tailoring interventions based on individual patient characteristics and medical histories.
- Cost-Effectiveness:
By optimizing resources and prioritizing safety efforts, AI and ML contribute to cost-effective vaccine safety management strategies.
Challenges and Considerations:
Despite their benefits, AI and ML in vaccine safety profile analysis present challenges:
- Data Quality:
Ensuring the accuracy, completeness, and reliability of data inputs is crucial for generating meaningful insights and avoiding biased outcomes.
- Interpretability:
Complex AI and ML models may produce results that are difficult to interpret or explain, posing challenges for regulatory decision-making and public trust.
- Ethical and Legal Issues:
Navigating issues related to data privacy, patient confidentiality, and regulatory compliance requires robust governance frameworks and adherence to ethical standards.
- Integration with Existing Systems:
Integrating AI and ML technologies into existing vaccine safety infrastructure may require updates to data management systems, IT capabilities, and workforce training.
Real-World Applications:
Several real-world applications demonstrate the effectiveness of AI and ML in vaccine safety profile analysis:
- Vaccine Adverse Event Reporting System (VAERS):
VAERS utilizes AI algorithms to analyze adverse event reports and detect potential safety signals associated with vaccines in the United States.
- European Medicines Agency (EMA) Edra Vigilance:
Edra Vigilance employs ML techniques to monitor and evaluate the safety of vaccines and medicines across Europe, enhancing regulatory decision-making.
- Global Vaccine Safety Initiative (GVSI):
The WHO-led GVSI leverages AI-driven analytics to support vaccine safety monitoring and surveillance in low- and middle-income countries, improving global health outcomes.
Future Implications:
The future of AI and ML in vaccine safety profile analysis holds promising developments:
- Advancements in Predictive Analytics:
Further development of predictive models will enable more accurate forecasting of vaccine safety outcomes and personalized risk assessments.
- Integration with Precision Medicine:
Combining AI with genomic and biometric data will enhance precision medicine approaches to vaccine safety, optimizing efficacy and safety profiles.
- Enhanced Regulatory Approaches:
Regulatory bodies will continue to integrate AI and ML technologies into safety assessment frameworks, enhancing transparency, efficiency, and global harmonization.
- Ethical AI Frameworks:
Developing ethical guidelines and frameworks for AI and ML applications in vaccine safety will promote responsible use and mitigate potential risks.
Conclusion:
AI and ML are pivotal in transforming vaccine safety profile analysis by enhancing signal detection, improving data integration, facilitating real-time monitoring, and optimizing risk prediction. Despite challenges, their benefits in efficiency, accuracy, real-time insights, personalized medicine, and cost-effectiveness underscore their indispensable role in public health. As we navigate future advancements and regulatory landscapes, leveraging AI and ML technologies responsibly will be crucial in safeguarding vaccine safety and promoting global health security. By fostering collaboration, innovation, and ethical standards, stakeholders can harness the full potential of AI and ML to ensure safe and effective immunization programs worldwide.
Comments