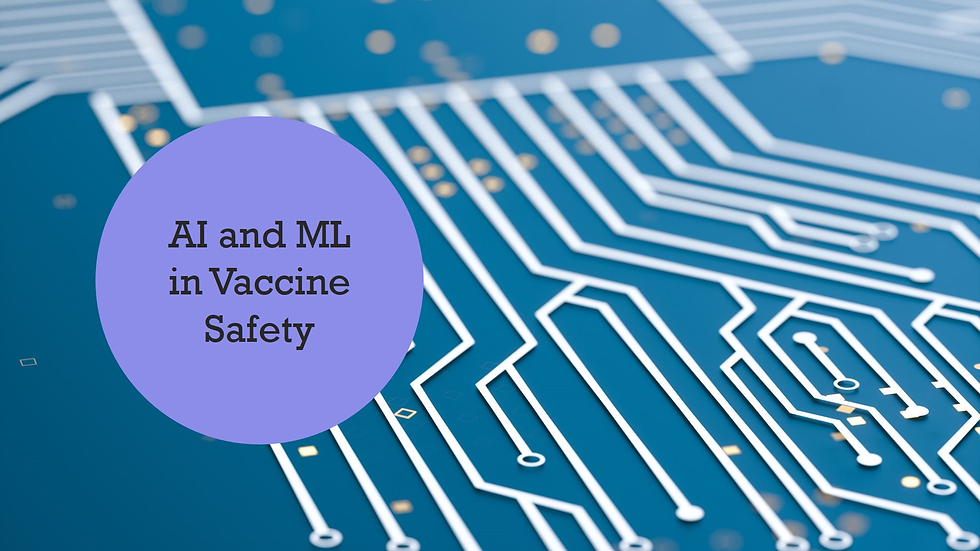
Ensuring vaccine safety is critical for public health, particularly during large-scale immunization programs. As vaccines reach millions of people worldwide, monitoring for potential adverse events becomes complex. Traditional pharmacovigilance practices, while essential, often struggle to process the sheer volume of safety data generated from clinical trials, post-marketing surveillance, electronic health records (EHRs), and spontaneous adverse event reports. This is where Artificial Intelligence (AI) and Machine Learning (ML) play a transformative role. These technologies automate processes, uncover hidden patterns, and improve the speed and accuracy of adverse event detection in vaccine safety monitoring.
This blog explores how AI and ML facilitate adverse event detection, offering deeper insights, timely interventions, and improved safety outcomes for vaccines.
1. The Importance of Adverse Event Detection in Vaccine Safety
Adverse events following immunization (AEFI) are any unfavorable medical occurrences that happen after a vaccine is administered. These can range from mild reactions (e.g., fever or soreness) to more severe events like allergic reactions. Detecting these events promptly ensures swift intervention, builds public trust, and ensures compliance with regulatory frameworks.
However, challenges in monitoring AEFIs include:
Large datasets: Millions of reports are generated from various sources, including healthcare systems and patient-reported data.
Delayed detection: Manually processing reports can result in delays, potentially endangering public health.
Complex patterns: Some adverse events may have rare occurrences or emerge only in specific populations or under certain conditions, making them hard to detect.
AI and ML offer solutions by automating data processing, recognizing patterns, and identifying safety signals in real-time.
2. How AI and ML Transform Adverse Event Detection
2.1. Automating Data Collection and Preprocessing
The first step in adverse event detection involves gathering data from multiple sources, such as:
Spontaneous reports from patients and healthcare professionals
EHRs and vaccine registries
Social media and online forums
Clinical trial outcomes
AI-powered systems can automatically collect and consolidate this data, ensuring comprehensive coverage. Additionally, AI tools preprocess the data by:
Cleaning duplicate entries to avoid redundant analysis
Handling missing values with imputation techniques
Standardizing formats to ensure consistency
This automation reduces manual workload and ensures that the data is ready for meaningful analysis.
2.2. Using Natural Language Processing (NLP) to Extract Relevant Information
Many adverse event reports are unstructured, containing free-text descriptions. Manually analyzing these reports is time-consuming and prone to errors. NLP algorithms enable AI systems to extract relevant information (e.g., symptoms, drug names, and timelines) from large volumes of unstructured text.
For instance, NLP can process:
Patient-reported symptoms on social media platforms
Physician notes from EHRs
Case narratives from individual case safety reports (ICSRs)
By extracting key data elements, NLP helps safety teams identify emerging safety issues faster.
2.3. Detecting Patterns and Safety Signals Using Machine Learning
Machine learning models are highly effective at identifying patterns that may not be obvious to human analysts. In vaccine safety monitoring, ML algorithms analyze vast datasets to uncover associations between vaccines and adverse events.
Supervised learning models:
These models are trained on historical data to predict the likelihood of an adverse event occurring.
Example: A logistic regression model may predict the probability of allergic reactions based on patient demographics and vaccine type.
Unsupervised learning models:
These models detect previously unknown patterns or clusters of adverse events.
Example: A clustering algorithm may identify rare but serious side effects that occur in a specific age group.
These insights help safety teams detect safety signals proactively and respond promptly.
2.4. Real-Time Monitoring with AI-Powered Dashboards
AI enables real-time monitoring by continuously analyzing new data as it becomes available. AI-powered dashboards allow pharmacovigilance teams to track vaccine safety trends and potential signals in real-time.
Features of AI-based dashboards:
Visualization tools: Graphs and heatmaps to identify adverse event trends
Alert systems: Notifications for new or emerging signals
Filtering capabilities: Drill-down views based on demographics, geography, or vaccine type
This real-time surveillance ensures faster detection and response to potential safety concerns.
2.5. Predictive Analytics for Proactive Safety Management
In addition to detecting existing safety signals, ML models can predict future risks, enabling proactive interventions. Predictive analytics involves using historical data to forecast potential adverse events and identify at-risk populations.
Examples of Predictive Models in Vaccine Safety:
Survival analysis models estimate the likelihood of adverse events over time after vaccination.
Decision trees and random forests predict patient groups that are more likely to experience severe side effects.
Predictive analytics allows healthcare providers to plan for contingencies and mitigate risks effectively.
3. Enhancing Signal Detection with Hybrid AI Models
Hybrid AI models combine multiple AI techniques to improve the accuracy of adverse event detection. For example:
NLP + ML models: NLP extracts relevant data from free-text reports, which ML algorithms then analyze for patterns.
Deep learning + supervised learning: Deep learning identifies complex patterns in large datasets, while supervised learning validates the results based on historical data.
These hybrid models enhance both the precision and recall of adverse event detection systems, minimizing the chances of false positives and false negatives.
4. Regulatory Compliance and AI-Driven Adverse Event Detection
AI and ML systems help organizations comply with regulatory requirements by ensuring:
Timely reporting: AI enables faster identification and submission of safety reports to regulatory bodies (e.g., FDA, EMA).
Data standardization: ML algorithms ensure that safety reports align with international standards, such as ICH E2B and MedDRA.
Audit readiness: Automated systems maintain accurate records, facilitating smooth regulatory audits.
Regulatory bodies are increasingly adopting AI tools for signal detection, making it essential for pharmaceutical companies to align their systems with these technologies.
5. Challenges and Solutions in Implementing AI and ML for Vaccine Safety
5.1. Data Privacy and Security Concerns
AI systems often process sensitive patient data, raising concerns about data privacy.
Solution: Implement data anonymization techniques and comply with regulations like GDPR and HIPAA to ensure privacy and security.
5.2. Algorithm Bias
AI algorithms may exhibit bias if trained on incomplete or unrepresentative data.
Solution: Regularly validate and retrain models with diverse datasets to reduce bias and improve fairness.
5.3. Integration with Existing Systems
Legacy systems may not be compatible with AI tools, posing integration challenges.
Solution: Use APIs and interoperability protocols (e.g., FHIR) to ensure seamless data exchange.
6. Case Study: AI-Enhanced Vaccine Safety Monitoring During COVID-19
During the COVID-19 pandemic, pharmacovigilance systems faced unprecedented challenges in monitoring the safety of new vaccines. Governments and pharmaceutical companies adopted AI-driven solutions to manage the surge in safety data.
Key actions included:
NLP-powered analysis of spontaneous adverse event reports to identify common side effects.
Real-time monitoring dashboards for tracking safety signals globally.
Predictive analytics to anticipate risks in specific populations (e.g., elderly or immunocompromised individuals).
These AI-enabled approaches ensured faster detection of potential safety concerns, building public trust in the vaccination process.
7. The Future of AI and ML in Vaccine Safety
The future of vaccine safety monitoring will increasingly rely on AI and ML technologies. Emerging trends include:
Integration with wearable devices: AI can analyze data from wearables to monitor patient health in real-time.
Federated learning models: These allow AI systems to learn from distributed data without compromising privacy.
Advanced predictive analytics: Future models will provide even more accurate forecasts for safety risks.
As AI technology evolves, vaccine safety monitoring will become more efficient, ensuring better health outcomes.
8. Conclusion
AI and ML are revolutionizing vaccine safety by automating data collection, enhancing signal detection, and enabling real-time monitoring. These technologies improve the speed and accuracy of adverse event detection, ensuring faster interventions and greater public trust. However, challenges such as data privacy, algorithm bias, and system integration must be addressed to fully realize the potential of AI in pharmacovigilance.
By adopting AI-driven solutions, pharmaceutical companies and regulatory agencies can create safer vaccination programs, protect public health, and maintain compliance with evolving regulatory standards. As the landscape of vaccine safety continues to grow, AI and ML will remain at the forefront of adverse event detection, ensuring that vaccines remain both safe and effective.
Comentarios