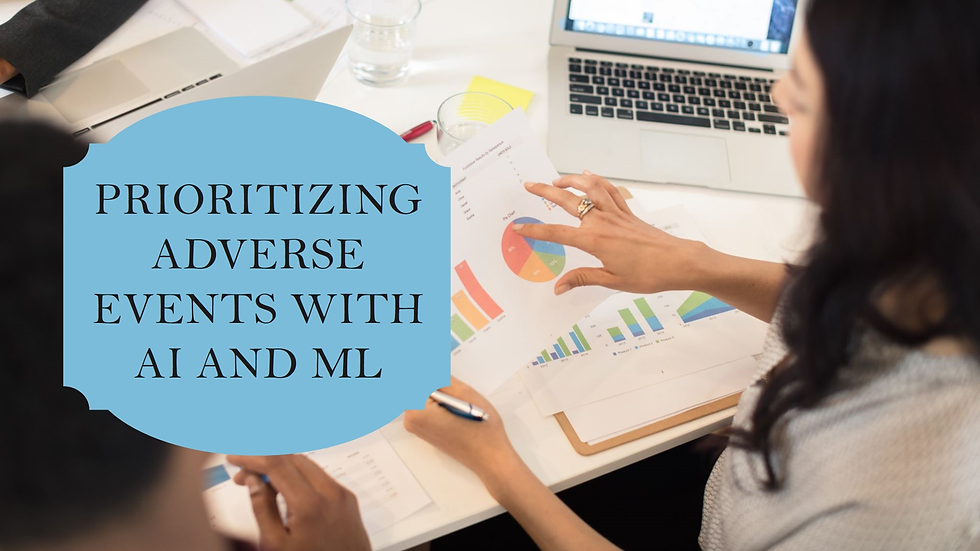
In today’s rapidly evolving landscape of healthcare and pharmaceuticals, the monitoring and management of adverse events (AEs) have become increasingly crucial. Adverse events refer to any undesired or harmful outcomes resulting from medical treatment or interventions, including medications, procedures, and therapies. The ability to prioritize these events effectively is vital for ensuring patient safety and improving therapeutic outcomes. Traditional methods of adverse event reporting and analysis have often been resource-intensive, time-consuming, and limited in their capacity to handle the vast amounts of data generated in clinical settings. This is where Artificial Intelligence (AI) and Machine Learning (ML) step in, transforming how adverse events are prioritized and managed.
Understanding Adverse Event Prioritization:
Adverse event prioritization involves identifying and ranking AEs based on their severity, frequency, and potential impact on patient safety. This process allows healthcare professionals and regulatory agencies to focus their attention and resources on the most critical issues, ultimately enhancing patient care and compliance with safety regulations.
Traditional approaches to AE prioritization often rely on manual reporting systems, which can be cumbersome and prone to errors. Additionally, these systems typically use standardized criteria to assess AEs, which may not account for the nuanced complexities of individual cases. As a result, many significant adverse events can go unreported or underreported, leading to inadequate responses from healthcare providers and regulatory agencies.
The Role of AI and ML in AE Prioritization:
1. Data Integration and Analysis
AI and ML algorithms can process vast amounts of data from various sources, including electronic health records (EHRs), clinical trial databases, social media, and patient-reported outcomes. By integrating and analyzing this diverse data, AI can identify patterns and trends that might be missed using traditional methods. This holistic approach to data analysis allows for more accurate AE detection and prioritization.
For instance, Natural Language Processing (NLP), a subfield of AI, can analyze unstructured data, such as clinical notes and patient feedback, to identify adverse events that may not have been formally reported. By converting this qualitative data into quantitative insights, healthcare providers can gain a clearer understanding of the prevalence and severity of specific AEs.
2. Predictive Analytics
Machine learning algorithms excel at predictive analytics, enabling healthcare organizations to anticipate potential adverse events before they occur. By training models on historical data, AI can identify risk factors associated with particular treatments, patient demographics, and other variables. This predictive capability allows clinicians to proactively address potential safety concerns, ultimately reducing the incidence of adverse events.
For example, if a specific medication has historically been associated with a higher risk of a particular AE in certain patient populations (e.g., elderly patients or those with pre-existing conditions), AI can flag these individuals for closer monitoring or suggest alternative therapies. This targeted approach to patient care enhances safety and improves outcomes.
3. Risk Stratification
AI and ML can enhance risk stratification by categorizing patients based on their likelihood of experiencing adverse events. By utilizing advanced algorithms, healthcare providers can identify high-risk patients who may benefit from closer monitoring or intervention. This tailored approach ensures that resources are allocated efficiently, focusing on those most in need of additional support.
For instance, patients undergoing specific treatments or surgeries can be stratified based on their medical history, genetic predispositions, and other factors. AI can help identify patients at a higher risk of complications, allowing clinicians to implement preventative measures tailored to individual patients.
4. Continuous Monitoring and Real-Time Alerts
AI systems can facilitate continuous monitoring of patients by analyzing data in real time. This capability allows healthcare providers to receive immediate alerts when potential adverse events occur, enabling them to respond quickly and effectively. Real-time monitoring systems can track vital signs, lab results, and other clinical indicators, identifying deviations from expected outcomes that may signal an adverse event.
For example, an AI system might analyze a patient's vital signs during surgery and alert the surgical team if it detects signs of a potential AE, such as changes in heart rate or blood pressure. This immediate response can be critical in preventing severe complications and improving patient outcomes.
5. Improved Reporting and Documentation
AI and ML can streamline the reporting and documentation of adverse events, making the process more efficient and accurate. Traditional AE reporting systems often require significant manual input, leading to delays and errors in data collection. AI can automate many aspects of this process, reducing the burden on healthcare providers and ensuring that adverse events are documented consistently.
For instance, AI-driven systems can automatically extract relevant data from EHRs and populate reporting forms, reducing the time and effort required by clinicians. This automation can improve the accuracy of AE reports, leading to better data for analysis and decision-making.
Case Studies Demonstrating AI and ML in AE Prioritization:
Several organizations have begun to harness the power of AI and ML for adverse event prioritization, demonstrating the effectiveness of these technologies in improving patient safety.
Case Study 1: FDA’s Sentinel Initiative
The U.S. Food and Drug Administration (FDA) has implemented the Sentinel Initiative, a national electronic system designed to monitor the safety of FDA-regulated medical products. By leveraging large databases of healthcare data, including claims data and EHRs, Sentinel utilizes machine learning algorithms to identify potential safety signals associated with medications and vaccines. This proactive approach allows the FDA to prioritize investigations into adverse events, improving patient safety and regulatory compliance.
Case Study 2: IBM Watson for Health
IBM Watson for Health employs AI and machine learning technologies to analyze vast amounts of healthcare data, including clinical trials, research studies, and EHRs. By identifying patterns and correlations, Watson can assist healthcare providers in prioritizing adverse events based on their severity and frequency. For example, Watson has been utilized to analyze AE data related to oncology treatments, helping clinicians identify potential complications and adjust treatment plans accordingly.
Case Study 3: The ALERT Project
The ALERT project, a collaborative initiative between several healthcare institutions, uses AI and machine learning to improve AE reporting and prioritization in pediatric patients. By analyzing data from EHRs, the project aims to identify adverse events in real-time and prioritize interventions for high-risk patients. Initial results have shown a significant reduction in adverse events among participating hospitals, highlighting the potential of AI in enhancing patient safety.
Challenges and Considerations:
While AI and ML hold great promise in facilitating adverse event prioritization, there are several challenges and considerations that must be addressed:
Data Privacy and Security
The use of AI and ML in healthcare raises concerns regarding patient privacy and data security. Organizations must ensure that they comply with regulations such as the Health Insurance Portability and Accountability Act (HIPAA) while utilizing these technologies. Protecting sensitive patient information is paramount to maintaining trust and ensuring compliance.
Data Quality and Bias
The effectiveness of AI and ML algorithms relies heavily on the quality of the data used for training. Poor-quality data can lead to biased outcomes, which may disproportionately affect certain patient populations. Ensuring that training datasets are representative and comprehensive is crucial for minimizing bias and maximizing the accuracy of predictions.
Integration into Clinical Workflows
For AI and ML technologies to be effective in adverse event prioritization, they must be seamlessly integrated into existing clinical workflows. Healthcare providers need user-friendly systems that enhance their decision-making processes without adding complexity or burden.
Conclusion:
AI and ML technologies are revolutionizing the way adverse events are prioritized in healthcare and pharmaceuticals. By leveraging advanced data analytics, predictive modeling, and real-time monitoring, these technologies enable healthcare professionals to identify, assess, and respond to adverse events more effectively. As the industry continues to evolve, it is essential for organizations to address the challenges and considerations associated with AI implementation, ensuring that patient safety remains at the forefront of healthcare delivery.
By embracing AI and ML, the healthcare sector can enhance its ability to manage adverse events, ultimately leading to better patient outcomes, improved therapeutic interventions, and increased regulatory compliance. As we move into the future, the integration of these technologies will play a critical role in shaping the landscape of patient safety and healthcare quality.
Comments