How AI and ML Facilitate Case Prioritization in Medical Device Safety
- Chaitali Gaikwad
- Jun 8, 2024
- 4 min read
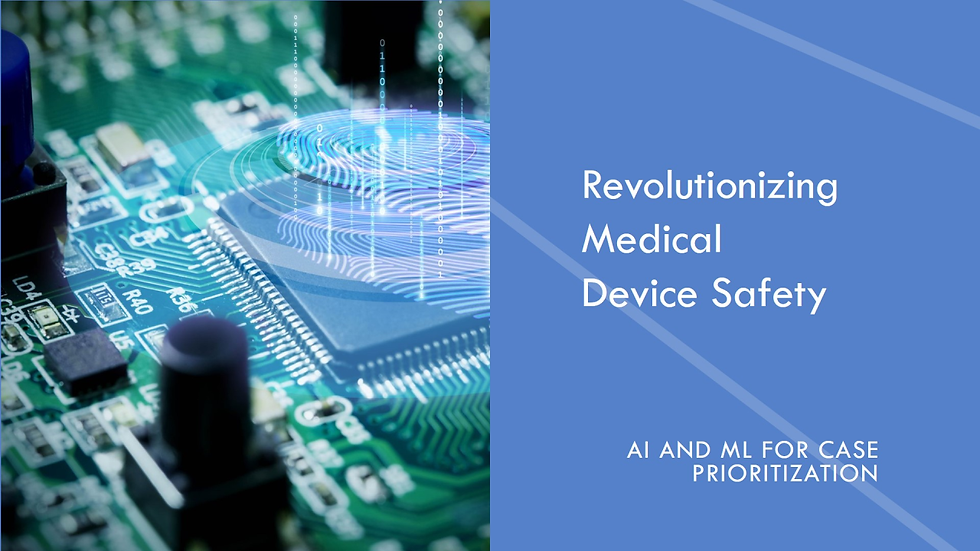
In the realm of healthcare, ensuring the safety and efficacy of medical devices is paramount. With advancements in technology, Artificial Intelligence (AI) and Machine Learning (ML) have emerged as game-changers, revolutionizing various aspects of healthcare, including medical device safety. One crucial area where AI and ML are making a significant impact is in the prioritization of cases related to medical device safety. In this blog, we will delve into how AI and ML facilitate case prioritization in medical device safety, thereby enhancing patient care and regulatory processes.
Understanding Case Prioritization:
Case prioritization involves the systematic assessment and ranking of reported adverse events or safety concerns associated with medical devices. These events can range from minor issues to serious complications, and prioritizing them effectively is vital for prompt intervention and regulatory action. Traditionally, case prioritization relied on manual review processes, which were time-consuming and prone to human error. However, the integration of AI and ML technologies has transformed this approach, enabling faster and more accurate prioritization.
Utilizing AI for Data Analysis:
One of the primary strengths of AI in case prioritization is its ability to analyze vast amounts of data efficiently. Medical device safety data often include diverse sources such as adverse event reports, clinical studies, patient records, and regulatory databases. AI algorithms can process these heterogeneous data sources and identify patterns, trends, and correlations that may indicate potential safety issues. By analyzing historical data, AI systems can also learn from past cases and improve their predictive capabilities over time.
Machine Learning for Predictive Modeling:
Machine Learning algorithms play a crucial role in predictive modeling for case prioritization. These algorithms can be trained using labeled datasets, where each case is categorized based on its severity or priority level. By analyzing features such as patient demographics, device characteristics, and clinical outcomes, ML models can learn to predict the likelihood of adverse events or safety concerns associated with specific medical devices. This predictive capability enables regulatory agencies and healthcare providers to proactively identify and address potential risks before they escalate.
Enhanced Signal Detection:
Signal detection is a key component of case prioritization, aimed at identifying potential safety signals or emerging trends that warrant further investigation. AI-powered signal detection systems leverage advanced algorithms to sift through large volumes of data and detect subtle patterns indicative of safety issues. These systems can analyze unstructured data from sources like social media, patient forums, and medical literature, providing valuable insights into real-world experiences with medical devices. By augmenting traditional signal detection methods with AI, regulators can identify safety concerns more rapidly and initiate appropriate actions to mitigate risks.
Automated Risk Stratification:
In addition to identifying safety signals, AI and ML enable automated risk stratification, which involves categorizing reported cases based on their potential impact and urgency. ML models can assign risk scores to individual cases by considering various factors such as the severity of adverse events, patient demographics, device usage patterns, and clinical context. This automated risk stratification allows regulatory agencies and healthcare providers to prioritize their resources effectively, focusing on cases with the highest likelihood of causing harm or requiring intervention.
Continuous Learning and Adaptation:
One of the inherent advantages of AI and ML is their ability to continuously learn and adapt in response to new data. As more safety-related information becomes available, AI systems can update their algorithms and refine their predictive models accordingly. This iterative process ensures that case prioritization remains dynamic and responsive to evolving trends and emerging threats. By leveraging real-time data feeds and feedback mechanisms, AI-powered systems can stay ahead of the curve and provide timely insights into medical device safety.
Integration with Regulatory Frameworks:
The integration of AI and ML technologies into existing regulatory frameworks is essential for maximizing their impact on medical device safety. Regulatory agencies such as the FDA (Food and Drug Administration) and EMA (European Medicines Agency) play a central role in overseeing the safety and effectiveness of medical devices. By incorporating AI-powered tools into their surveillance and monitoring systems, these agencies can enhance their capacity to identify, evaluate, and respond to safety concerns in a more proactive and efficient manner. Moreover, collaboration between regulators, industry stakeholders, and healthcare professionals is crucial for ensuring the successful implementation of AI-driven approaches to case prioritization.
Challenges and Considerations:
While AI and ML offer significant benefits for case prioritization in medical device safety, several challenges and considerations must be addressed. These include data quality issues, algorithm bias, interpretability of results, privacy concerns, and regulatory compliance. Ensuring transparency and accountability in AI-driven decision-making processes is essential to maintain trust and credibility among stakeholders. Moreover, ongoing validation and validation of AI models are necessary to verify their performance and reliability in real-world settings.
Conclusion:
In conclusion, AI and ML technologies are transforming the landscape of medical device safety by revolutionizing case prioritization processes. By leveraging the power of data analysis, predictive modeling, signal detection, and risk stratification, AI-powered systems enable regulators and healthcare providers to identify and respond to safety concerns more effectively. However, realizing the full potential of AI in medical device safety requires addressing various challenges and considerations, including data quality, algorithm bias, and regulatory compliance. Through collaboration and innovation, AI-driven approaches have the potential to enhance patient care, improve regulatory oversight, and ultimately save lives in the ever-evolving field of healthcare.
コメント