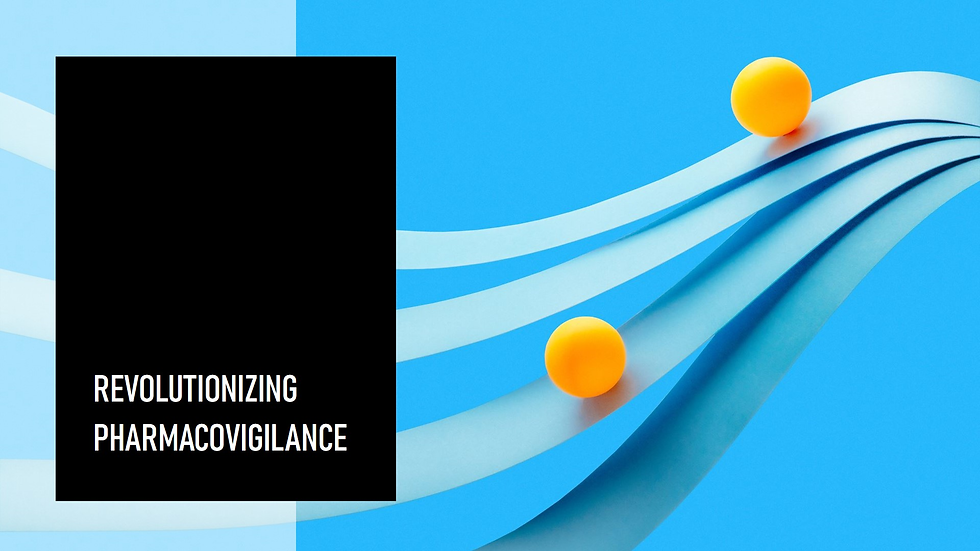
In the ever-evolving field of pharmacovigilance, the safety and efficacy of drugs are of paramount importance. As new medications enter the market, the need for robust monitoring systems to identify and assess adverse drug reactions (ADRs) becomes increasingly critical. One of the significant challenges in pharmacovigilance is the integration of data from various sources, such as clinical trials, electronic health records, and spontaneous reporting systems. Artificial Intelligence (AI) and Machine Learning (ML) are revolutionizing data integration in pharmacovigilance, enabling better analysis, enhanced signal detection, and improved decision-making processes. This blog explores how AI and ML facilitate data integration in pharmacovigilance, their benefits, challenges, and future potential.
Understanding Pharmacovigilance:
Pharmacovigilance is the science and activities related to the detection, assessment, understanding, and prevention of adverse effects or any other drug-related problems. It aims to improve patient safety and ensure that the benefits of medications outweigh their risks. The pharmacovigilance process involves several steps, including:
Data Collection: Gathering information about ADRs from various sources, including healthcare providers, patients, clinical studies, and electronic health records.
Data Analysis: Evaluating the collected data to identify patterns, trends, and potential safety signals.
Signal Detection: Identifying potential safety concerns that require further investigation, such as unusual rates of specific ADRs.
Risk Assessment: Assessing the likelihood that a drug may cause an adverse event and determining the overall benefit-risk profile.
Regulatory Action: Reporting findings to regulatory authorities and implementing necessary changes to drug labeling, usage recommendations, or withdrawal from the market.
The Role of Data Integration in Pharmacovigilance:
Data integration is a critical component of pharmacovigilance, as it allows for a comprehensive view of a drug's safety profile. By combining data from multiple sources, pharmacovigilance teams can:
Identify trends and correlations that may not be apparent when analyzing isolated datasets.
Improve the accuracy and reliability of safety assessments.
Enhance the detection of rare adverse events that may only emerge when analyzing large populations.
Facilitate timely decision-making regarding risk management strategies.
However, integrating data from various sources presents several challenges, including:
Data Silos: Data may be stored in different systems or formats, making it difficult to aggregate and analyze.
Data Quality Issues: Inconsistent data entry, missing information, and variations in reporting practices can affect the accuracy of integrated datasets.
Complexity of Data Types: Pharmacovigilance data can include structured data (e.g., numeric values) and unstructured data (e.g., free-text reports), complicating integration efforts.
How AI and ML Facilitate Data Integration:
AI and ML technologies offer powerful solutions to address the challenges of data integration in pharmacovigilance. Here’s how they facilitate this process:
1. Automated Data Extraction and Standardization
AI algorithms can automatically extract relevant information from diverse data sources, including clinical trial reports, electronic health records, and spontaneous ADR reports. Natural Language Processing (NLP), a subfield of AI, enables machines to understand and interpret human language, allowing for the extraction of valuable insights from unstructured text data.
Example: NLP can be used to identify ADRs mentioned in free-text clinical notes, transforming them into structured data that can be easily integrated into databases.
Once the data is extracted, AI can also help standardize the information, ensuring that it adheres to specific formats and terminologies. This standardization is crucial for effective integration and analysis.
2. Data Cleaning and Validation
Data quality is critical in pharmacovigilance, and AI-powered tools can help improve the accuracy and reliability of integrated datasets. Machine learning algorithms can analyze incoming data to identify and correct errors, inconsistencies, and missing values.
Example: AI can detect duplicate entries, flag anomalies, and fill in missing information based on patterns in the data, ensuring that the integrated dataset is as accurate as possible.
3. Integration of Structured and Unstructured Data
Pharmacovigilance involves both structured data (e.g., numerical values, categorical data) and unstructured data (e.g., free-text narratives). AI and ML technologies can bridge the gap between these data types, enabling seamless integration.
Example: Machine learning models can analyze unstructured clinical narratives to extract relevant ADR information and convert it into structured data formats for integration into safety databases.
4. Enhanced Signal Detection and Analysis
AI and ML algorithms excel at identifying patterns and correlations within large datasets. By applying these technologies to integrated pharmacovigilance data, organizations can enhance signal detection and improve the identification of potential safety concerns.
Example: Machine learning algorithms can analyze vast amounts of data to detect unusual patterns in ADR reporting, helping pharmacovigilance teams identify safety signals that may require further investigation.
5. Predictive Analytics
Predictive analytics powered by AI and ML can provide insights into potential safety risks before they become significant issues. By analyzing historical data and identifying risk factors, these technologies can help organizations proactively manage drug safety.
Example: Machine learning models can predict the likelihood of specific adverse events based on patient demographics, drug characteristics, and previous ADR reports, allowing for more targeted monitoring and intervention strategies.
6. Real-Time Monitoring and Reporting
AI and ML can enable real-time monitoring of pharmacovigilance data, allowing organizations to respond quickly to emerging safety concerns. Automated reporting systems can provide up-to-date information on ADR trends, facilitating timely decision-making.
Example: AI algorithms can continuously monitor incoming data from various sources, alerting pharmacovigilance teams to potential safety signals as they arise.
Benefits of AI and ML in Data Integration:
The integration of AI and ML in pharmacovigilance offers several key benefits:
Improved Efficiency: Automation of data extraction, cleaning, and standardization processes reduces the time and effort required for data integration, allowing pharmacovigilance teams to focus on analysis and decision-making.
Enhanced Data Quality: AI-powered tools can improve the accuracy and consistency of integrated datasets, leading to more reliable safety assessments.
Timely Signal Detection: Real-time monitoring and predictive analytics enable organizations to identify safety signals promptly, allowing for quicker interventions and risk management strategies.
Comprehensive Safety Profiles: By integrating diverse data sources and types, AI and ML provide a more holistic view of a drug's safety profile, enhancing the ability to detect rare adverse events.
Cost-Effectiveness: By streamlining data integration processes, organizations can reduce operational costs associated with pharmacovigilance activities.
Challenges and Considerations:
While AI and ML offer significant advantages in data integration for pharmacovigilance, there are challenges and considerations to keep in mind:
Data Privacy and Security: Handling sensitive patient data requires robust security measures to ensure compliance with data protection regulations, such as GDPR and HIPAA.
Bias in Algorithms: Machine learning algorithms can be susceptible to biases present in training data, potentially leading to skewed results. Ensuring that AI models are trained on diverse and representative datasets is essential.
Interpretability: The "black box" nature of some AI algorithms can make it challenging for pharmacovigilance professionals to understand how decisions are made, necessitating the development of more interpretable models.
Integration with Existing Systems: Implementing AI and ML solutions may require significant changes to existing data management systems, which can be resource-intensive.
Continuous Learning: AI and ML models need to be continuously updated and retrained with new data to maintain accuracy and relevance over time.
Future Potential of AI and ML in Pharmacovigilance:
The future of pharmacovigilance is poised to be significantly shaped by advancements in AI and ML technologies. As these technologies continue to evolve, their potential applications in pharmacovigilance are expected to expand:
Personalized Medicine: AI and ML can contribute to personalized medicine by analyzing patient-specific data to predict individual responses to medications, allowing for more tailored treatment plans and monitoring strategies.
Blockchain for Data Integrity: The integration of blockchain technology with AI and ML can enhance data integrity and traceability in pharmacovigilance, ensuring that safety data is secure and tamper-proof.
Patient Engagement: AI-powered tools can facilitate patient engagement in pharmacovigilance by enabling easier reporting of adverse events and providing educational resources about drug safety.
Global Collaboration: AI and ML can facilitate collaboration between regulatory agencies, pharmaceutical companies, and healthcare providers on a global scale, enhancing the collective understanding of drug safety.
Advanced Predictive Modeling: The development of more sophisticated predictive models can further enhance risk assessment and management strategies in pharmacovigilance.
Conclusion:
AI and ML technologies are revolutionizing data integration in pharmacovigilance, enabling organizations to enhance their monitoring and assessment of drug safety. By automating data extraction, cleaning, and analysis, these technologies streamline the integration of diverse data sources, improving the accuracy and reliability of safety assessments. The ability to detect safety signals in real-time and predict potential risks empowers pharmacovigilance teams to respond swiftly to emerging concerns.
While challenges remain, the benefits of AI and ML in pharmacovigilance are significant, offering improved efficiency, data quality, and timely decision-making. As these technologies continue to evolve, their applications in pharmacovigilance are expected to expand, ultimately contributing to safer and more effective medications for patients. Embracing AI and ML in pharmacovigilance is not just a technological advancement; it is a crucial step toward ensuring the health and safety of populations around the world.
Comments