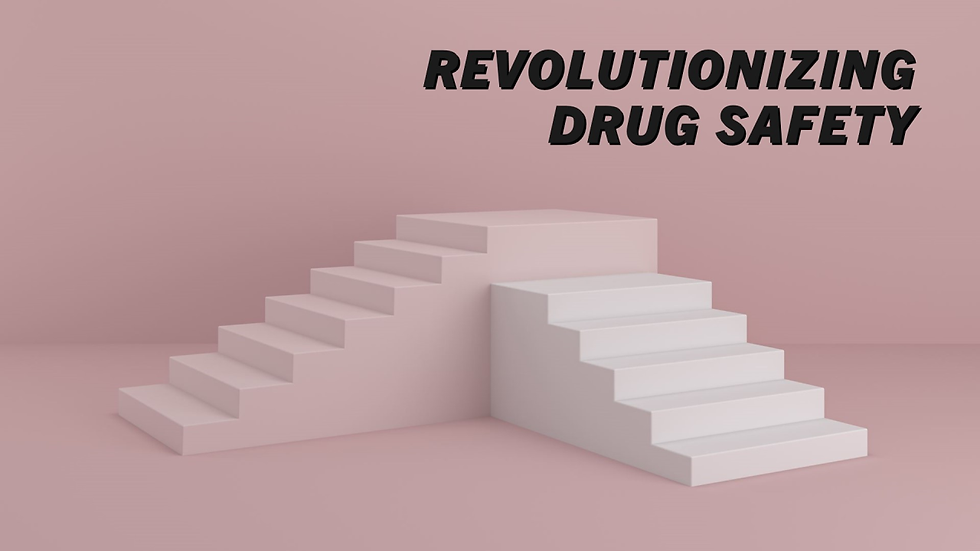
In the realm of pharmacovigilance, the timely detection and analysis of adverse events (AEs) associated with medications are crucial for ensuring patient safety and regulatory compliance. Traditional methods of AE detection often rely on manual review and reporting, which can be time-consuming and prone to errors. However, advancements in artificial intelligence (AI) and machine learning (ML) have revolutionized the field, offering innovative solutions for more accurate and efficient AE detection. This blog explores how AI and ML technologies are transforming adverse event detection in drug safety, enhancing patient care and public health outcomes.
Understanding Adverse Event Detection:
Adverse events refer to any untoward medical occurrence associated with the use of a pharmaceutical product in a patient. These events can range from mild reactions like nausea or headaches to severe complications such as organ failure or death. Detecting and analyzing AEs is critical for identifying potential safety concerns, assessing the risks and benefits of medications, and informing regulatory decisions.
Challenges with Traditional Methods:
Traditional methods of AE detection typically rely on spontaneous reporting systems, where healthcare professionals and patients voluntarily report suspected adverse reactions to regulatory authorities or pharmaceutical companies. While these systems have been valuable for identifying safety signals, they have several limitations:
Underreporting: Many adverse events go unreported due to lack of awareness, time constraints, or uncertainty about causality.
Bias: Reporting biases, such as overrepresentation of severe events or recent media attention, can skew the data.
Data Volume: The sheer volume of reported events can overwhelm manual review processes, leading to delays in signal detection.
Data Quality: Incomplete or inaccurate data entries can compromise the reliability of the reported information.
The Role of AI and ML in Adverse Event Detection:
AI and ML technologies offer innovative solutions to overcome the limitations of traditional AE detection methods. These technologies leverage algorithms and computational techniques to analyze large volumes of data, identify patterns, and make predictions without explicit programming instructions. In the context of drug safety, AI and ML can enhance adverse event detection in several ways:
1. Automated Data Processing
AI algorithms can automatically process vast amounts of structured and unstructured data from diverse sources, including electronic health records, social media, medical literature, and wearable devices. By extracting relevant information and standardizing data formats, AI
streamlines the data collection process and prepares it for analysis.
2. Natural Language Processing (NLP)
NLP algorithms enable computers to understand and interpret human language, allowing for the analysis of unstructured text data such as clinical notes, patient narratives, and social media posts. NLP can identify mentions of adverse events, extract key information, and categorize events based on severity, onset, duration, and other relevant parameters.
3. Pattern Recognition
ML algorithms excel at identifying patterns and correlations within complex datasets. By analyzing historical AE data, ML models can identify common risk factors, temporal trends, and associations between drugs and adverse events. This enables early detection of safety signals and prediction of potential risks before they escalate.
4. Signal Detection
AI-powered signal detection algorithms can flag potential safety signals by analyzing deviations from expected event frequencies, clustering of events in specific patient populations, or temporal associations between drug exposure and adverse outcomes. These algorithms can prioritize signals based on statistical significance, clinical relevance, and regulatory requirements.
5. Pharmacovigilance Triage
AI-driven triage systems can automatically categorize reported adverse events based on their severity, urgency, and potential impact on patient safety. By prioritizing events for further review, triage systems optimize the allocation of human resources and ensure timely follow-up on high-risk events.
Case Studies and Success Stories:
Numerous case studies and real-world applications demonstrate the effectiveness of AI and ML in adverse event detection:
FDA Sentinel System: The FDA's Sentinel System utilizes AI and ML algorithms to monitor real-world data from electronic health records and insurance claims databases for adverse drug reactions. The system has successfully identified safety signals for various medications, leading to label updates and regulatory actions.
Social Media Monitoring: Pharmaceutical companies and regulatory agencies use AI-powered social media monitoring tools to track mentions of adverse events on platforms like Twitter, Facebook, and patient forums. These tools enable rapid identification of emerging safety concerns and facilitate proactive risk management strategies.
Electronic Health Records Analysis: Hospitals and healthcare systems leverage AI algorithms to analyze electronic health records for adverse event signals. By integrating clinical data with medication usage and laboratory results, these systems enhance post-market surveillance and support evidence-based decision-making.
Challenges and Considerations:
While AI and ML offer tremendous potential for improving adverse event detection in drug safety, several challenges and considerations must be addressed:
Data Quality: Ensuring the accuracy, completeness, and consistency of input data is essential for the reliability of AI models.
Interpretability: Making AI models interpretable and transparent is critical for understanding their decision-making processes and gaining trust from stakeholders.
Regulatory Compliance: AI-driven adverse event detection systems must comply with regulatory requirements, including data privacy laws and reporting standards.
Bias and Fairness: Mitigating bias in AI algorithms and ensuring fairness in adverse event detection across diverse patient populations is an ongoing challenge.
Future Directions and Opportunities:
The future of AI and ML in adverse event detection is promising, with several opportunities for further innovation:
Personalized Medicine: AI algorithms can facilitate the identification of patient-specific risk factors for adverse events, enabling personalized interventions and treatment strategies.
Predictive Analytics: ML models can predict adverse events based on patient characteristics, genetic markers, and other factors, allowing for preemptive risk mitigation measures.
Real-Time Surveillance: AI-powered surveillance systems can provide real-time monitoring of adverse events, enabling rapid response to emerging safety signals and public health threats.
Conclusion:
AI and ML technologies have revolutionized adverse event detection in drug safety, offering unprecedented capabilities for analyzing complex datasets, identifying safety signals, and improving patient care outcomes. By harnessing the power of AI, pharmaceutical companies, regulatory agencies, and healthcare providers can enhance pharmacovigilance practices, mitigate risks, and ensure the continued safety and efficacy of medications. As technology continues to advance, AI-driven adverse event detection will play an increasingly integral role in safeguarding public health and advancing the field of drug safety.
Comments