How AI and ML Improve Data Quality in Drug Safety Systems
- Chaitali Gaikwad
- Oct 22, 2024
- 6 min read
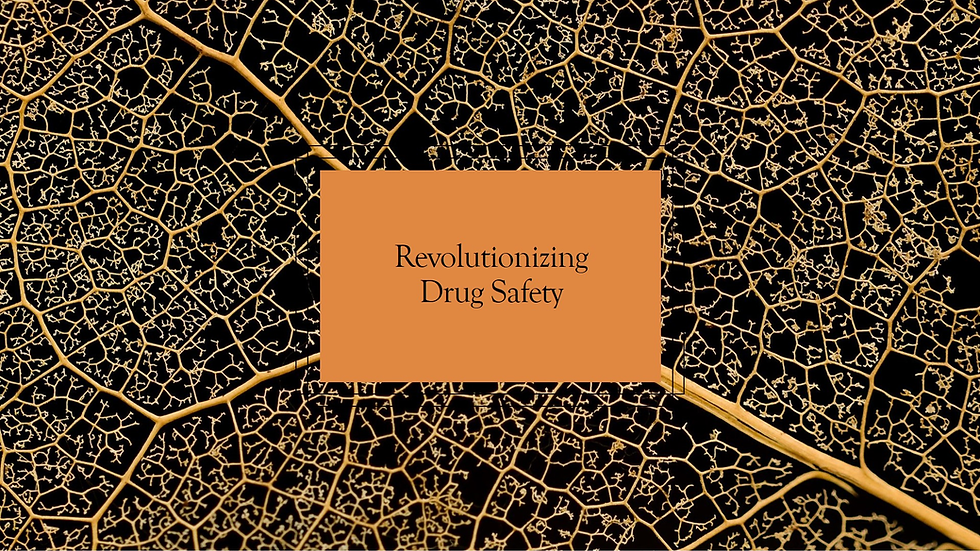
In the ever-evolving landscape of healthcare and pharmaceuticals, ensuring data quality in drug safety systems is paramount. As regulatory scrutiny increases and the complexity of drug development expands, the demand for reliable and high-quality data is more critical than ever. Artificial Intelligence (AI) and Machine Learning (ML) have emerged as transformative technologies in this realm, offering innovative solutions to enhance data quality in drug safety systems. This blog explores how AI and ML improve data quality, the methodologies involved, and the implications for the future of pharmacovigilance.
The Importance of Data Quality in Drug Safety
Data quality is crucial in drug safety for several reasons:
Patient Safety: Accurate data is essential for identifying adverse drug reactions (ADRs) and ensuring that medications are safe for public use. Poor data quality can lead to misinterpretations of safety signals, potentially endangering patients.
Regulatory Compliance: Regulatory agencies, such as the FDA and EMA, require pharmaceutical companies to maintain high standards of data integrity. Failing to comply with these standards can result in penalties, delayed approvals, and harm to a company's reputation.
Signal Detection: Effective signal detection relies on the integrity of data collected during clinical trials and post-marketing surveillance. High-quality data allows for timely identification of safety concerns, enabling swift regulatory actions.
Operational Efficiency: Improving data quality reduces the need for rework, minimizes errors, and streamlines processes within drug safety systems, ultimately leading to cost savings and faster decision-making.
How AI and ML Enhance Data Quality
AI and ML offer a range of capabilities that can significantly enhance data quality in drug safety systems:
1. Data Validation and Cleaning
AI algorithms can automate the data validation process by identifying anomalies, inconsistencies, and errors in large datasets. Traditional methods often rely on manual checks, which can be time-consuming and prone to human error. Machine learning models can learn from historical data patterns and flag data points that deviate from the norm, ensuring that only high-quality data is included in safety assessments.
For example, an ML model can analyze adverse event reports and identify outliers, such as unusually high or low values in the data. By using natural language processing (NLP) techniques, these models can also extract relevant information from unstructured data sources, such as clinical notes or social media posts, thereby enriching the dataset for analysis.
2. Enhanced Signal Detection
AI and ML can improve signal detection capabilities by analyzing vast amounts of data from multiple sources, including electronic health records (EHRs), clinical trial databases, and real-world evidence. Traditional signal detection methods often rely on predefined criteria, which may miss emerging safety signals.
With machine learning, models can adapt to new data and identify patterns that may indicate potential safety concerns. For instance, a supervised learning model can be trained to recognize the relationships between various factors, such as patient demographics, medication usage, and reported adverse events, enabling more nuanced signal detection.
3. Predictive Analytics
Predictive analytics, powered by AI and ML, allows drug safety professionals to anticipate potential safety issues before they manifest. By analyzing historical data, these technologies can identify risk factors associated with specific drugs or patient populations, enabling proactive risk management strategies.
For example, machine learning models can analyze data from previous clinical trials and post-marketing reports to predict which patients may be at a higher risk for specific adverse reactions. This information can inform labeling decisions and guide healthcare providers in making safer prescribing choices.
4. Streamlined Data Integration
Drug safety systems often involve data from multiple sources, such as clinical trials, spontaneous reporting systems, and literature reviews. Integrating these diverse data streams can be challenging, particularly when dealing with varying formats and standards. AI and ML can facilitate this integration by automating data harmonization and ensuring consistent data formats.
Natural language processing techniques can be employed to extract relevant information from unstructured data sources, such as free-text reports or literature articles. By converting this unstructured data into a structured format, organizations can create a unified dataset for analysis, ultimately improving data quality and reducing discrepancies.
5. Real-Time Monitoring and Reporting
AI and ML enable real-time monitoring of adverse events, enhancing the ability to respond quickly to emerging safety concerns. Traditional pharmacovigilance methods often involve retrospective analysis of data, which can delay the identification of potential risks. In contrast, AI-driven systems can continuously analyze incoming data and generate alerts for any concerning trends or anomalies.
For example, a machine learning model can be trained to monitor social media for patient-reported outcomes and adverse events related to specific drugs. By analyzing this data in real-time, pharmacovigilance teams can identify potential safety signals faster and take appropriate actions to protect patients.
Methodologies for Implementing AI and ML in Drug Safety
To effectively leverage AI and ML in improving data quality within drug safety systems, organizations should consider the following methodologies:
1. Define Clear Objectives
Before implementing AI and ML solutions, it’s essential to establish clear objectives. Identify the specific data quality challenges your organization faces and determine how AI and ML can address those issues. Whether it's improving signal detection, enhancing data validation, or streamlining reporting processes, having well-defined goals will guide your implementation strategy.
2. Invest in Quality Data
The effectiveness of AI and ML models heavily relies on the quality of the data used for training and validation. Invest in robust data collection and management practices to ensure that the datasets used are accurate, complete, and representative of the population being studied. This may involve regular data cleaning and validation efforts to maintain data integrity.
3. Collaborate with Data Scientists
Building effective AI and ML models requires expertise in data science and machine learning techniques. Collaborate with data scientists to develop tailored algorithms that address your specific data quality challenges. These experts can help design and implement models that are not only accurate but also interpretable, ensuring that stakeholders can understand the rationale behind the predictions.
4. Continuous Learning and Improvement
AI and ML models should not be static; they must evolve as new data becomes available. Implement a continuous learning framework where models are regularly updated and retrained with new data. This ensures that the models remain relevant and effective in addressing emerging safety concerns.
5. Monitor and Evaluate Outcomes
After implementing AI and ML solutions, continuously monitor and evaluate their performance in improving data quality. Establish key performance indicators (KPIs) to measure the effectiveness of the models in enhancing data validation, signal detection, and overall pharmacovigilance processes. Use this feedback to make iterative improvements and optimize the models further.
Challenges and Considerations
While AI and ML offer significant benefits for improving data quality in drug safety systems, there are several challenges to consider:
Data Privacy and Security: The use of sensitive health data raises privacy and security concerns. Organizations must ensure compliance with regulations, such as HIPAA and GDPR, to protect patient information.
Bias and Fairness: AI and ML models can inadvertently perpetuate biases present in the training data. It’s crucial to assess the models for fairness and ensure that they do not disadvantage specific patient populations.
Interpretability: Many AI and ML models, particularly deep learning algorithms, can be challenging to interpret. Ensuring that the decision-making processes of these models are transparent and understandable to stakeholders is essential for gaining trust and acceptance.
Integration with Existing Systems: Implementing AI and ML solutions may require integrating them with existing drug safety systems. This can be complex and may require significant resources and time to achieve a seamless integration.
Future Implications
The integration of AI and ML into drug safety systems is likely to continue evolving, with several future implications:
Personalized Medicine: As AI and ML become more sophisticated, they will enable the development of personalized medicine approaches, where drug safety monitoring is tailored to individual patient characteristics, improving patient outcomes.
Enhanced Regulatory Frameworks: Regulatory agencies are increasingly recognizing the potential of AI and ML in pharmacovigilance. Future regulatory frameworks may incorporate guidelines for the use of these technologies in ensuring data quality and patient safety.
Collaborative Approaches: The collaboration between pharmaceutical companies, regulatory agencies, and technology providers will be vital in leveraging AI and ML effectively. Sharing data and insights will foster a more comprehensive understanding of drug safety.
Conclusion:
AI and ML are revolutionizing the way data quality is managed in drug safety systems. By automating data validation, enhancing signal detection, and enabling real-time monitoring, these technologies significantly improve the reliability and integrity of safety data. However, organizations must navigate challenges related to data privacy, bias, and interpretability while continuously evaluating and refining their AI and ML models.
As the field of pharmacovigilance evolves, embracing AI and ML will not only enhance data quality but also ultimately contribute to better patient safety and regulatory compliance. The future of drug safety lies in harnessing the power of these transformative technologies, paving the way for more effective and efficient pharmacovigilance practices.
Call to Action
If you’re involved in drug safety or pharmacovigilance, consider exploring how AI and ML can enhance your data quality initiatives. Invest in training and collaboration, and stay informed about the latest developments in technology to ensure that your organization remains at the forefront of ensuring patient safety.
Comments