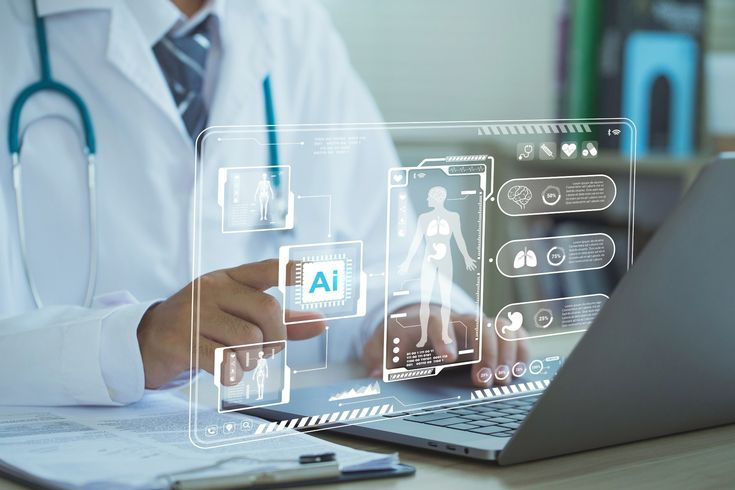
Pharmacovigilance (PV), the science of monitoring the safety of pharmaceutical products, has long depended on collecting and analyzing vast amounts of data. The sheer volume of data generated from clinical trials, post-market surveillance, spontaneous reporting systems, literature monitoring, and real-world evidence poses challenges to traditional methods of maintaining data quality. Inaccurate, incomplete, or delayed data can compromise patient safety and regulatory compliance. This is where artificial intelligence (AI) and machine learning (ML) have begun to revolutionize the pharmacovigilance landscape.
By leveraging AI and ML, organizations can significantly enhance data quality within pharmacovigilance databases. These advanced technologies can automate complex data collection, processing, and analysis tasks, reducing errors, improving consistency, and enabling real-time insights into drug safety. This blog will delve into the key ways AI and ML improve data quality in PV databases, examining their impact on data accuracy, timeliness, completeness, and consistency.
1. Automation of Data Entry and Collection
One of the primary areas where AI and ML impact data quality is in automating the data entry and collection process. Traditional manual data entry methods are prone to errors such as misreporting, duplication, or missing information. In pharmacovigilance, this can lead to delays in detecting safety signals or inaccurate conclusions about a drug’s risk profile.
AI-Powered Optical Character Recognition (OCR): AI-powered OCR tools can process and extract relevant information from structured and unstructured data sources, such as adverse event reports, medical literature, and patient records. This automation reduces the likelihood of human error, improving the quality of data entered into pharmacovigilance databases.
Natural Language Processing (NLP): NLP is a subset of AI that can interpret and understand human language. In pharmacovigilance, NLP can automate the extraction of relevant safety data from diverse sources, such as free-text case reports or social media posts, ensuring that key information is captured accurately.
Robotic Process Automation (RPA): RPA can be used to automate repetitive tasks, such as data extraction from regulatory submissions or adverse event reports. By reducing human involvement in data entry, RPA minimizes errors and ensures more consistent data quality.
2. Improving Data Accuracy
Data accuracy is crucial in pharmacovigilance. Inaccurate data can lead to false safety signals, delayed risk mitigation, or incorrect regulatory reporting, potentially compromising patient safety. AI and ML enhance data accuracy by identifying and correcting errors in real-time.
Machine Learning Algorithms for Error Detection: ML algorithms can be trained to detect anomalies and inconsistencies in pharmacovigilance data. For example, if there’s a mismatch between a patient’s reported adverse reaction and the drug’s known side effect profile, an ML algorithm can flag this inconsistency for further review.
Automated Signal Detection: AI-powered systems can analyze vast datasets to identify early warning signals for adverse drug reactions more efficiently than traditional manual methods. By continuously scanning pharmacovigilance databases, AI can detect subtle patterns and correlations in data that may otherwise go unnoticed, leading to more accurate and timely safety assessments.
Data Cleansing Tools: AI-based data cleansing tools can automatically clean and standardize data in pharmacovigilance databases, removing duplicate entries, correcting errors, and filling in missing data. These tools can ensure that the data used for analysis is accurate and reliable.
3. Enhancing Data Completeness
Incomplete data in pharmacovigilance can obscure the true safety profile of a drug and hinder effective decision-making. AI and ML are transforming the way data is collected and integrated, ensuring more comprehensive coverage and completeness.
Predictive Data Completion: ML models can predict missing information based on patterns and historical data, ensuring that data gaps are filled intelligently. For instance, if an adverse event report is missing certain demographic information, AI algorithms can infer the most likely values based on other similar cases, ensuring that the report remains usable for analysis.
Integration of Diverse Data Sources: AI and ML can efficiently integrate data from multiple sources, such as electronic health records (EHRs), wearable devices, social media, and real-world evidence databases. This integration creates a more complete picture of drug safety by capturing data that might not be available through traditional reporting mechanisms.
Automating Literature Monitoring: In pharmacovigilance, monitoring published literature for adverse drug reactions is critical. AI-powered tools can automate literature searches, continuously scanning new studies and publications for relevant safety information. This automation ensures that all pertinent data is captured and incorporated into pharmacovigilance databases, improving completeness.
4. Ensuring Data Consistency
Consistency is another important aspect of data quality. Inconsistent data across different sources or systems can result in conflicting safety assessments, delayed regulatory reporting, or difficulty detecting safety signals. AI and ML technologies help standardize data formats and ensure consistency in pharmacovigilance databases.
Standardizing Terminology: AI tools can standardize terminology across different datasets by mapping terms to standardized dictionaries, such as the Medical Dictionary for Regulatory Activities (MedDRA). For instance, different reports may use different terms for the same adverse event, but AI can harmonize this information to ensure consistency in data analysis.
Reconciliation of Disparate Data: ML algorithms can reconcile discrepancies between different data sources. For example, if adverse event data from a clinical trial database conflicts with data from a spontaneous reporting system, ML can analyze and harmonize the data, ensuring that the final dataset used for decision-making is consistent.
Real-Time Data Monitoring: AI and ML can provide real-time monitoring of pharmacovigilance databases, continuously scanning for inconsistencies or discrepancies. This capability ensures that any issues are flagged and addressed immediately, preventing downstream problems in data analysis or reporting.
5. Facilitating Real-Time Data Analysis
The ability to analyze pharmacovigilance data in real-time is critical for detecting emerging safety signals and ensuring timely responses to potential risks. AI and ML enable organizations to process and analyze vast amounts of data quickly and efficiently.
AI-Powered Analytics Platforms: AI-based analytics platforms can process data in real-time, identifying trends, patterns, and anomalies that might signal an adverse drug reaction. This rapid analysis allows pharmacovigilance teams to act more swiftly in assessing and mitigating risks.
Machine Learning Models for Predictive Analytics: Predictive analytics models built on machine learning can forecast potential safety issues before they become significant problems. For example, ML algorithms can analyze historical adverse event data and predict the likelihood of future events, helping pharmacovigilance teams prioritize safety assessments.
Automated Reporting: AI can automate the generation of regulatory reports, ensuring that the data submitted to regulatory agencies is accurate, up-to-date, and compliant with reporting requirements. This automation reduces the risk of errors in regulatory submissions and improves the timeliness of safety reporting.
6. Addressing Data Privacy and Security Concerns
As AI and ML improve data quality in pharmacovigilance databases, it's essential to address potential concerns regarding data privacy and security. Pharmacovigilance systems handle sensitive patient information, and the use of AI introduces new challenges in maintaining data confidentiality.
AI-Driven Data Anonymization: AI can anonymize patient data to protect privacy while preserving the integrity of the information needed for safety analysis. Advanced algorithms can remove or encrypt personally identifiable information (PII) without compromising the utility of the data for pharmacovigilance purposes.
Security Monitoring: AI-powered security systems can monitor pharmacovigilance databases for suspicious activities, unauthorized access, or data breaches. By ensuring the security of data, AI helps maintain the integrity and confidentiality of pharmacovigilance databases.
Conclusion:
AI and ML are transforming pharmacovigilance by enhancing the quality of data used to monitor drug safety. These technologies improve data accuracy, completeness, consistency, and timeliness, enabling more reliable safety assessments and faster detection of potential risks. Through automation, real-time data analysis, and advanced error detection, AI and ML streamline pharmacovigilance processes, ensuring that pharmaceutical companies and healthcare organizations can maintain compliance and protect patient safety.
As the volume of pharmacovigilance data continues to grow, the adoption of AI and ML will become increasingly critical. By improving data quality in pharmacovigilance databases, these technologies not only enhance regulatory reporting and safety monitoring but also contribute to a more robust, efficient, and proactive approach to safeguarding public health.
Commenti