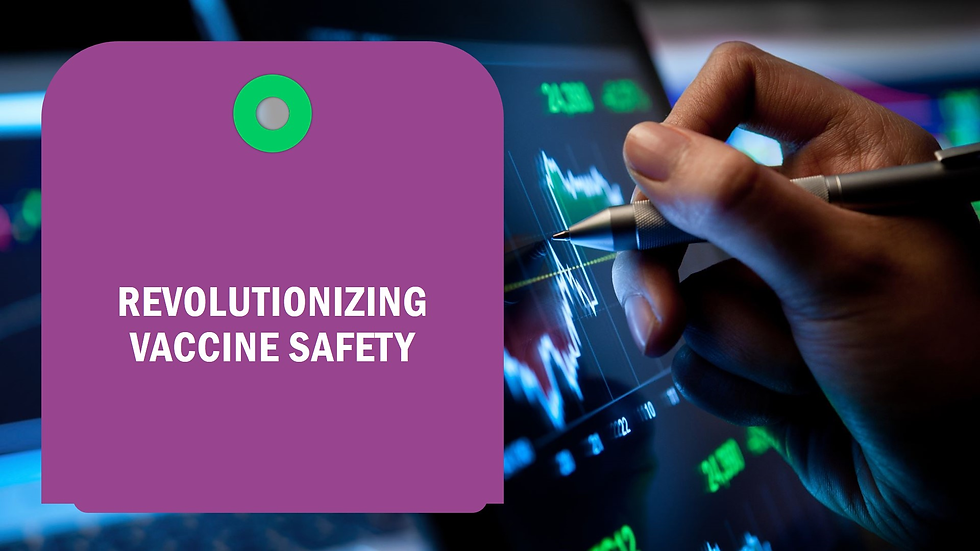
In the realm of healthcare, the safety of vaccines is paramount. With the rapid development and deployment of vaccines, especially in response to global health crises like the COVID-19 pandemic, ensuring data quality in vaccine safety systems has never been more critical. Artificial Intelligence (AI) and Machine Learning (ML) technologies have emerged as powerful tools to enhance the quality and reliability of data within these systems. This blog explores how AI and ML improve data quality in vaccine safety systems, focusing on their applications, benefits, challenges, and future directions.
Understanding Vaccine Safety Systems
Vaccine safety systems are designed to monitor the safety of vaccines throughout their lifecycle, from preclinical trials to post-marketing surveillance. These systems collect and analyze vast amounts of data, including:
Adverse Event Reporting: Monitoring any adverse events following vaccination, which may indicate safety concerns.
Clinical Trials Data: Assessing safety and efficacy during clinical trials.
Post-Marketing Surveillance: Evaluating vaccine safety in the general population once a vaccine is approved and distributed.
The primary goal of these systems is to ensure that the benefits of vaccination outweigh the risks, safeguarding public health. However, the large volume of data generated poses significant challenges in terms of data quality, integrity, and analysis.
The Role of AI and ML in Enhancing Data Quality
1. Automated Data Collection and Integration
One of the fundamental ways AI and ML improve data quality in vaccine safety systems is through automated data collection and integration.
Data Extraction: AI algorithms can automatically extract data from diverse sources, including electronic health records (EHRs), social media, and clinical trial reports. This reduces the risk of human error during data entry and ensures more comprehensive data collection.
Data Integration: ML techniques can integrate data from multiple sources, providing a unified view of vaccine safety. By using natural language processing (NLP), AI can analyze unstructured data from clinical notes, reports, and patient feedback, enhancing the breadth of data available for analysis.
2. Enhanced Data Accuracy and Consistency
AI and ML algorithms significantly improve the accuracy and consistency of data collected in vaccine safety systems.
Data Validation: AI can employ advanced validation techniques to identify inconsistencies, duplicates, and anomalies in the data. For example, ML models can analyze historical data to detect patterns and flag outliers, ensuring that only high-quality data is used for decision-making.
Predictive Analytics: Machine learning algorithms can predict potential adverse events based on historical data patterns. This proactive approach helps identify potential safety issues before they become widespread, ensuring that vaccine safety systems are always alert.
3. Real-Time Monitoring and Analysis
AI and ML enable real-time monitoring and analysis of vaccine safety data, which is crucial for timely decision-making.
Continuous Surveillance: AI systems can continuously monitor data streams from various sources, including social media and health agencies, to identify trends and potential safety signals in real time. This allows health authorities to respond promptly to emerging safety concerns.
Adaptive Learning: Machine learning models can adapt to new data and refine their predictions over time. As more data becomes available, these models improve their accuracy, leading to better decision-making in vaccine safety assessments.
4. Improved Signal Detection
Signal detection is a critical aspect of vaccine safety systems, and AI and ML play a significant role in enhancing this process.
Data Mining: AI algorithms can analyze vast amounts of data to identify potential safety signals that may not be apparent through traditional analysis methods. By employing sophisticated data mining techniques, ML can uncover hidden patterns and correlations that warrant further investigation.
Enhanced Risk Assessment: Machine learning models can assess the risk associated with specific adverse events, providing insights into the likelihood of occurrence based on historical data. This helps prioritize safety investigations and allocate resources effectively.
5. Streamlined Reporting Processes
The reporting process in vaccine safety systems can be cumbersome and time-consuming. AI and ML can streamline this process, improving efficiency and accuracy.
Automated Reporting: AI can automate the generation of reports required by regulatory agencies, ensuring that all necessary information is included and formatted correctly. This reduces the administrative burden on healthcare professionals and enhances compliance with reporting requirements.
Natural Language Generation: Using natural language generation (NLG), AI can create human-readable summaries of complex data sets, making it easier for stakeholders to understand vaccine safety information quickly.
Benefits of Using AI and ML in Vaccine Safety Systems
The integration of AI and ML into vaccine safety systems brings several significant benefits:
1. Enhanced Data Quality
AI and ML improve the accuracy, consistency, and completeness of data collected in vaccine safety systems, leading to more reliable safety assessments.
2. Timely Decision-Making
Real-time monitoring and predictive analytics enable quicker responses to emerging safety concerns, ensuring that public health authorities can act promptly to mitigate risks.
3. Resource Optimization
By automating data collection, validation, and reporting processes, AI and ML free up valuable resources, allowing healthcare professionals to focus on critical tasks related to vaccine safety.
4. Improved Public Confidence
Transparent and reliable vaccine safety monitoring, bolstered by AI and ML, enhances public confidence in vaccination programs, which is crucial for achieving herd immunity and overall public health goals.
Challenges in Implementing AI and ML in Vaccine Safety Systems
While the potential benefits of AI and ML are significant, there are also challenges associated with their implementation in vaccine safety systems:
1. Data Privacy and Security
The collection and analysis of sensitive health data raise concerns about privacy and data security. Ensuring compliance with regulations such as the Health Insurance Portability and Accountability Act (HIPAA) is essential to protect patient information.
2. Data Quality and Availability
Despite advancements in AI and ML, the quality of data used for training models is crucial. Inconsistent or incomplete data can lead to biased results and undermine the effectiveness of AI applications.
3. Interoperability Issues
Integrating AI and ML technologies with existing vaccine safety systems can pose interoperability challenges. Ensuring that different systems can communicate and share data seamlessly is critical for optimizing workflows.
4. Resistance to Change
Implementing new technologies often faces resistance from healthcare professionals accustomed to traditional methods. Training and education are necessary to facilitate the transition to AI and ML-enhanced systems.
Future Directions
As AI and ML technologies continue to evolve, their application in vaccine safety systems is expected to expand further:
1. Advanced Predictive Modeling
Future developments in predictive modeling techniques will enhance the ability to forecast adverse events and safety signals. By leveraging more sophisticated algorithms, vaccine safety systems can improve risk assessments and decision-making processes.
2. Greater Use of Real-World Data
The integration of real-world data, including patient-reported outcomes and social media data, into vaccine safety systems will provide a more comprehensive view of vaccine safety. AI and ML can help analyze this data to identify trends and insights that may not be captured in clinical trials.
3. Collaborative Platforms
The establishment of collaborative platforms that facilitate data sharing among stakeholders, including regulatory agencies, healthcare providers, and pharmaceutical companies, will enhance vaccine safety monitoring. AI and ML can streamline data integration and analysis across these platforms.
4. Enhanced Transparency
As public demand for transparency in vaccine safety increases, AI and ML can help provide accessible and understandable information to the public. Utilizing NLG and data visualization techniques can make complex safety data more digestible for non-experts.
Conclusion
AI and ML have the potential to revolutionize data quality in vaccine safety systems, enhancing the accuracy, consistency, and timeliness of data collection and analysis. By automating processes, improving signal detection, and facilitating real-time monitoring, these technologies play a vital role in ensuring vaccine safety and public health.
While challenges remain in implementing these technologies, the benefits they offer in improving vaccine safety systems are significant. As the healthcare landscape continues to evolve, embracing AI and ML will be crucial for ensuring that vaccination programs remain safe and effective in protecting public health. Through continued innovation and collaboration, the future of vaccine safety monitoring looks promising, ultimately contributing to better health outcomes for populations worldwide.
コメント