How AI and ML Improve Data Quality in Vaccine Safety Systems
- Chaitali Gaikwad
- Oct 28, 2024
- 5 min read
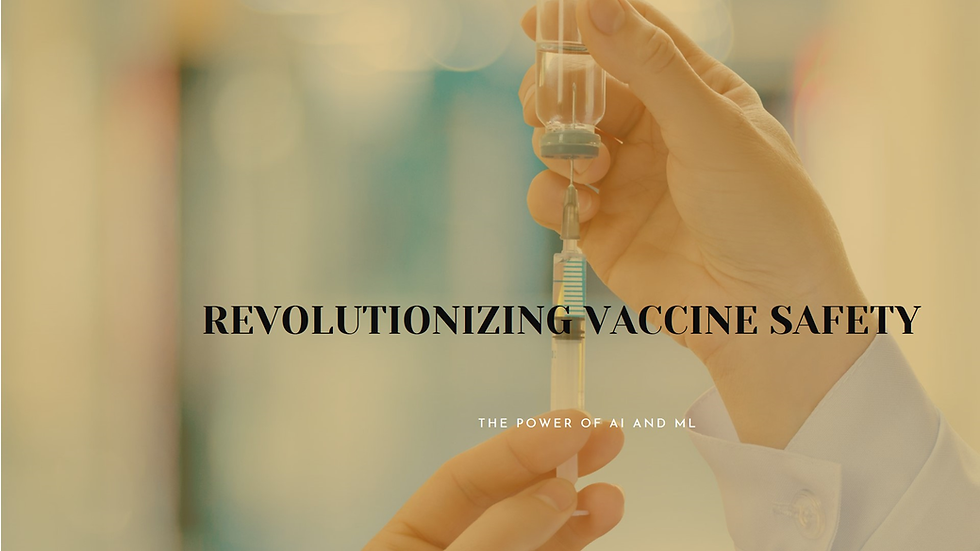
In an era of rapid technological advancement, artificial intelligence (AI) and machine learning (ML) are transforming the landscape of vaccine safety systems. These technologies enhance data quality by automating processes, improving accuracy, and enabling real-time analysis. As vaccines become more widely distributed globally, ensuring the safety and efficacy of these medical interventions has never been more critical. AI and ML provide tools that help public health officials and healthcare organizations monitor adverse events more effectively, thus maintaining trust in vaccination programs.
This blog will explore how AI and ML improve data quality in vaccine safety systems, discussing their applications, benefits, challenges, and future trends.
The Importance of Data Quality in Vaccine Safety
Data quality is vital for vaccine safety systems for several reasons:
Informed Decision-Making: Accurate data allows health authorities to make informed decisions regarding vaccine safety and efficacy. Poor data quality can lead to misinterpretations and ineffective public health responses.
Regulatory Compliance: Regulatory agencies, such as the FDA and EMA, require high-quality data to approve and monitor vaccines. Incomplete or inaccurate data can hinder regulatory processes.
Public Trust: The public's trust in vaccination programs hinges on transparent and accurate reporting of vaccine safety data. High data quality helps foster this trust.
Early Detection of Safety Signals: High-quality data enables the timely detection of potential safety signals associated with vaccines, allowing for prompt action to mitigate risks.
Applications of AI and ML in Vaccine Safety Systems
1. Data Collection and Integration
AI and ML facilitate the collection and integration of diverse data sources, including:
Electronic Health Records (EHRs): AI algorithms can extract relevant information from unstructured data in EHRs, such as clinical notes and laboratory results, improving the completeness and richness of data available for vaccine safety monitoring.
Social Media and Online Platforms: Natural language processing (NLP), a subset of AI, can analyze social media posts, forums, and other online content to identify adverse events that may not be reported through traditional channels.
Public Health Databases: AI can aggregate data from various public health databases, enhancing the breadth of information used for safety monitoring.
2. Data Cleaning and Validation
Ensuring the accuracy of data is crucial in vaccine safety monitoring. AI and ML algorithms can automatically clean and validate data by identifying and correcting errors. Techniques include:
Anomaly Detection: ML algorithms can identify outliers or anomalies in datasets, signaling potential errors that require further investigation.
Automated Data Standardization: AI can standardize data formats, making it easier to analyze and compare data from different sources.
Duplicate Record Identification: AI can recognize duplicate records within databases, ensuring that each adverse event is recorded only once, thus improving data integrity.
3. Signal Detection and Risk Assessment
AI and ML enhance the process of signal detection, which is essential for identifying potential safety concerns associated with vaccines:
Statistical Modeling: ML algorithms can analyze large datasets to detect unusual patterns or signals that might indicate a relationship between a vaccine and an adverse event. For example, Bayesian models can estimate the probability of a causal relationship between the vaccine and reported events.
Predictive Analytics: By analyzing historical data, AI can forecast potential adverse events and risks associated with specific vaccines, enabling proactive risk management.
Causality Assessment: AI can assist in determining the causality of adverse events by evaluating various factors, such as timing, severity, and biological plausibility.
4. Real-Time Monitoring and Reporting
AI and ML enable real-time monitoring of vaccine safety data, allowing for swift responses to emerging safety concerns:
Automated Alerts: Machine learning algorithms can generate alerts when adverse event reporting exceeds expected thresholds, prompting further investigation.
Dashboard Visualization: AI-powered dashboards can provide stakeholders with real-time insights into vaccine safety data, enabling quick decision-making.
Continuous Learning: ML algorithms can continuously learn from new data, improving their accuracy and effectiveness over time.
Benefits of AI and ML in Improving Data Quality
1. Enhanced Accuracy
AI and ML technologies significantly improve the accuracy of data collected and analyzed in vaccine safety systems. By automating data cleaning, validation, and signal detection, these technologies minimize human error and provide more reliable results.
2. Increased Efficiency
Automating data collection, validation, and analysis processes allows public health organizations to operate more efficiently. AI and ML enable healthcare professionals to focus on higher-level tasks, such as interpreting data and making decisions, rather than spending time on manual data management.
3. Comprehensive Data Analysis
AI and ML can analyze vast amounts of data from diverse sources, providing a comprehensive view of vaccine safety. This capability helps identify rare adverse events that may not be apparent through traditional monitoring methods.
4. Proactive Risk Management
AI and ML facilitate proactive risk management by identifying potential safety signals early. This early detection allows public health authorities to respond swiftly to emerging concerns, thus safeguarding public health.
5. Cost-Effectiveness
By streamlining data management processes and improving efficiency, AI and ML can reduce the costs associated with vaccine safety monitoring. Lower operational costs enable organizations to allocate resources more effectively, ultimately improving vaccine safety efforts.
Challenges in Implementing AI and ML in Vaccine Safety Systems
While the benefits of AI and ML in improving data quality are significant, several challenges must be addressed:
1. Data Privacy and Security
Collecting and analyzing sensitive health data raises concerns about privacy and security. Organizations must implement robust data protection measures to ensure compliance with regulations such as HIPAA and GDPR.
2. Data Quality and Availability
AI and ML rely on high-quality, comprehensive datasets to function effectively. Incomplete or biased data can lead to inaccurate predictions and conclusions. Ensuring data quality and availability is crucial for the success of AI and ML applications.
3. Integration with Existing Systems
Integrating AI and ML technologies with existing vaccine safety monitoring systems can be complex and resource-intensive. Organizations may face challenges in aligning new technologies with legacy systems and workflows.
4. Skill Gaps
The implementation of AI and ML technologies requires skilled personnel who can develop, maintain, and analyze these systems. Organizations may struggle to find qualified professionals with the necessary expertise.
5. Ethical Considerations
The use of AI and ML in public health raises ethical questions regarding decision-making, accountability, and transparency. Organizations must ensure that their AI-driven processes adhere to ethical standards and foster public trust.
Future Trends in AI and ML for Vaccine Safety
1. Improved Natural Language Processing (NLP)
As NLP technologies advance, they will become more effective at analyzing unstructured data from a variety of sources, enhancing the ability to detect adverse events from social media, forums, and other online platforms.
2. Enhanced Collaboration and Data Sharing
Future AI and ML systems will likely emphasize collaboration among public health organizations, regulatory agencies, and researchers, facilitating data sharing and improving the comprehensiveness of vaccine safety monitoring.
3. Greater Use of Wearable Technology
The increasing prevalence of wearable technology will provide real-time health data that can be integrated into vaccine safety systems, enabling continuous monitoring of vaccine recipients.
4. Advancements in Predictive Analytics
Future AI-driven predictive analytics will be more sophisticated, enabling earlier detection of potential adverse events and informing public health strategies to mitigate risks.
5. Emphasis on Explainability
As AI systems become more integrated into decision-making processes, there will be a growing focus on explainability and transparency, ensuring stakeholders understand how AI-driven insights are generated.
Conclusion
AI and ML are revolutionizing the way vaccine safety systems operate, significantly improving data quality and enabling more effective monitoring of adverse events. By enhancing data collection, cleaning, validation, signal detection, and real-time analysis, these technologies play a critical role in ensuring the safety and efficacy of vaccines.
Despite the challenges associated with implementing AI and ML in vaccine safety systems, the benefits they offer are undeniable. As technology continues to evolve, public health organizations must embrace these innovations to enhance vaccine safety monitoring efforts and maintain public trust in vaccination programs. Ultimately, leveraging AI and ML will help safeguard public health, ensuring that vaccination remains a cornerstone of disease prevention and control.
コメント