How AI and ML Improve Literature Review Accuracy
- Chaitali Gaikwad
- Sep 12, 2024
- 5 min read
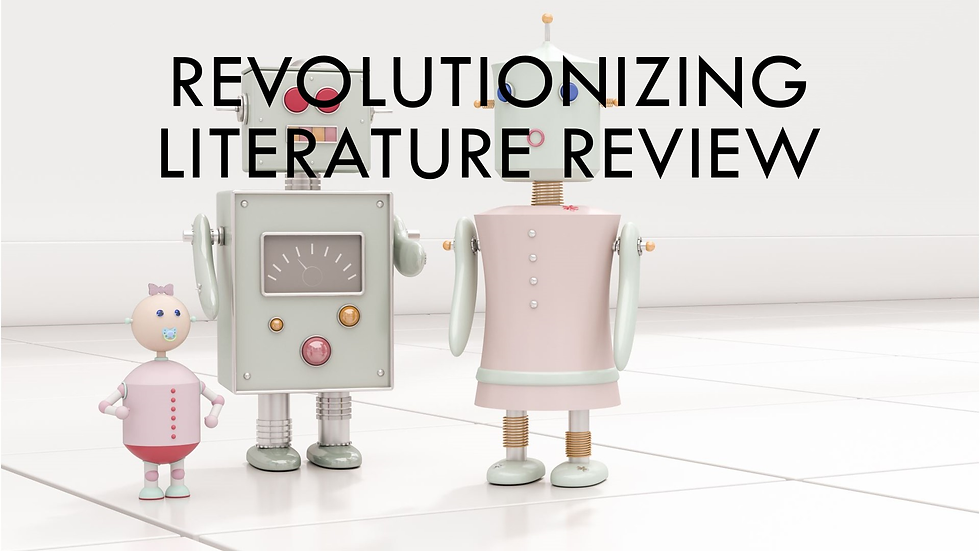
In the ever-expanding realm of research, literature reviews are fundamental for synthesizing existing knowledge, identifying gaps, and guiding future research. Traditionally, literature reviews are time-consuming and labor-intensive, often requiring manual searching, reading, and summarizing of vast amounts of literature. However, advancements in artificial intelligence (AI) and machine learning (ML) have revolutionized this process. By leveraging these technologies, researchers can enhance the accuracy and efficiency of literature reviews, making it possible to handle large volumes of data with greater precision. This blog explores how AI and ML improve literature review accuracy, detailing their applications, benefits, and practical implementation strategies.
1. Understanding AI and ML in the Context of Literature Reviews
Artificial Intelligence (AI) refers to the simulation of human intelligence in machines that are programmed to perform tasks that typically require human cognition. Machine Learning (ML), a subset of AI, involves the use of algorithms that enable machines to learn from data and improve their performance over time without explicit programming. In literature reviews, AI and ML technologies can automate and enhance various stages of the review process, from search and retrieval to analysis and synthesis.
2. Enhancing Search and Retrieval
a. Automated Literature Search
Traditional literature searches involve manually entering keywords and reviewing results, which can be inefficient and prone to oversight. AI-driven search engines and ML algorithms improve this process by:
Semantic Search: AI-powered search engines use natural language processing (NLP) to understand the context and meaning of search queries beyond simple keyword matching. This allows for more relevant and accurate search results by considering synonyms, related terms, and contextual meanings.
Contextual Recommendations: ML algorithms analyze user search patterns and previously accessed articles to recommend relevant literature. This personalized approach helps in discovering articles that may not have been identified through traditional search methods.
b. Advanced Filtering and Sorting
AI and ML can refine search results through advanced filtering and sorting capabilities:
Automatic Categorization: ML models categorize articles based on their content, relevance, and quality. This automatic tagging helps in organizing literature into meaningful groups, such as themes or topics, facilitating easier navigation and review.
Dynamic Filtering: AI algorithms apply dynamic filters based on user preferences and historical data, allowing researchers to focus on the most relevant studies and exclude irrelevant ones.
3. Improving Data Extraction and Summarization
a. Text Mining and NLP
AI and ML techniques in text mining and NLP significantly enhance data extraction and summarization:
Information Extraction: NLP algorithms can extract key information from research articles, such as study objectives, methods, results, and conclusions. This automated extraction reduces the manual effort required to gather and summarize data.
Abstract and Full-Text Summarization: AI-powered summarization tools generate concise summaries of abstracts and full texts, capturing the essential points of each study. This helps researchers quickly assess the relevance and quality of the literature without reading every document in full.
b. Identifying Key Themes and Patterns
AI and ML can analyze large volumes of text to identify key themes and patterns:
Topic Modeling: ML algorithms, such as Latent Dirichlet Allocation (LDA), identify underlying topics within a collection of articles. This helps in understanding the main themes and trends in the literature.
Trend Analysis: AI tools can track and analyze trends over time, providing insights into the evolution of research topics and identifying emerging areas of interest.
4. Enhancing Accuracy in Data Analysis
a. Citation Analysis
AI and ML improve citation analysis, which is crucial for evaluating the impact and relevance of research:
Citation Networks: ML algorithms analyze citation networks to identify influential papers and key contributors in a field. This helps in understanding the relationships between studies and recognizing seminal works.
Citation Prediction: AI models predict future citation trends based on historical data, helping researchers identify potentially impactful studies early on.
b. Identifying Research Gaps
AI and ML can assist in identifying gaps in the literature:
Gap Analysis: ML algorithms compare existing research with current trends and needs, highlighting areas that lack sufficient investigation. This informs researchers about potential research questions and opportunities for future studies.
Cluster Analysis: AI tools perform cluster analysis to group related studies and identify areas where research is sparse or fragmented.
5. Facilitating Efficient Review and Synthesis
a. Automated Review Tools
AI and ML facilitate the review and synthesis of literature:
Systematic Review Automation: AI-powered tools automate parts of the systematic review process, such as screening articles, assessing quality, and extracting data. This reduces the time and effort required for comprehensive reviews.
Synthesis Generation: ML algorithms synthesize findings from multiple studies to generate integrated summaries and insights. This helps in drawing overarching conclusions and making informed recommendations.
b. Collaborative Platforms
AI-enhanced collaborative platforms improve teamwork in literature reviews:
Real-Time Collaboration: AI tools support real-time collaboration by enabling researchers to share annotations, comments, and insights seamlessly. This enhances the efficiency and accuracy of collaborative reviews.
Knowledge Sharing: AI platforms facilitate knowledge sharing by integrating insights from different researchers and providing access to shared resources and findings.
6. Best Practices for Implementing AI and ML in Literature Reviews
To effectively leverage AI and ML for literature reviews, consider the following best practices:
a. Choose the Right Tools
Select AI and ML tools that align with your specific needs and research goals:
Evaluate Features: Assess the features and capabilities of various tools, such as search functionality, summarization quality, and data extraction accuracy.
Integration: Ensure that the chosen tools integrate seamlessly with existing research workflows and databases.
b. Train and Fine-Tune Models
Customize AI and ML models to improve their performance:
Model Training: Train models on relevant datasets to enhance their accuracy and relevance. Fine-tune algorithms to address specific research needs and contexts.
Continuous Learning: Implement continuous learning processes to update and refine models based on new data and evolving research trends.
c. Validate Results
Validate the accuracy and reliability of AI and ML-generated results:
Cross-Check Findings: Verify AI and ML outputs by cross-checking with manual reviews and expert assessments. Ensure that automated summaries and analyses align with actual content.
Feedback Loop: Establish a feedback loop to refine and improve AI and ML models based on user feedback and performance evaluations.
d. Address Ethical Considerations
Consider ethical implications when using AI and ML:
Data Privacy: Ensure that AI and ML tools comply with data privacy regulations and safeguard sensitive information.
Bias and Fairness: Address potential biases in AI models by using diverse datasets and implementing fairness checks to avoid skewed results.
7. Case Studies and Examples
a. AI-Powered Systematic Reviews
AI has been successfully used in systematic reviews to automate screening and data extraction. For example, tools like Covidence and Rayyan leverage AI to streamline the review process, improving efficiency and accuracy.
b. Machine Learning in Research Trend Analysis
ML algorithms have been employed to analyze research trends and identify emerging topics. Dimensions and Altmetric use AI to track research impact and trends, helping researchers stay informed about the latest developments.
c. NLP for Literature Summarization
NLP techniques are used to summarize vast amounts of literature efficiently. SciBERT, a variant of BERT (Bidirectional Encoder Representations from Transformers), has demonstrated effectiveness in generating summaries and extracting key information from scientific texts.
Conclusion:
AI and ML technologies have transformed the landscape of literature reviews, offering significant improvements in accuracy, efficiency, and scalability. By automating search and retrieval, enhancing data extraction and summarization, and facilitating accurate analysis and synthesis, these technologies enable researchers to handle vast volumes of information with greater precision. Implementing AI and ML tools effectively requires careful selection, customization, validation, and ethical considerations. As these technologies continue to evolve, they hold the potential to further revolutionize literature reviews, making them more accurate and insightful, and ultimately advancing the frontiers of knowledge across various fields.
Comments