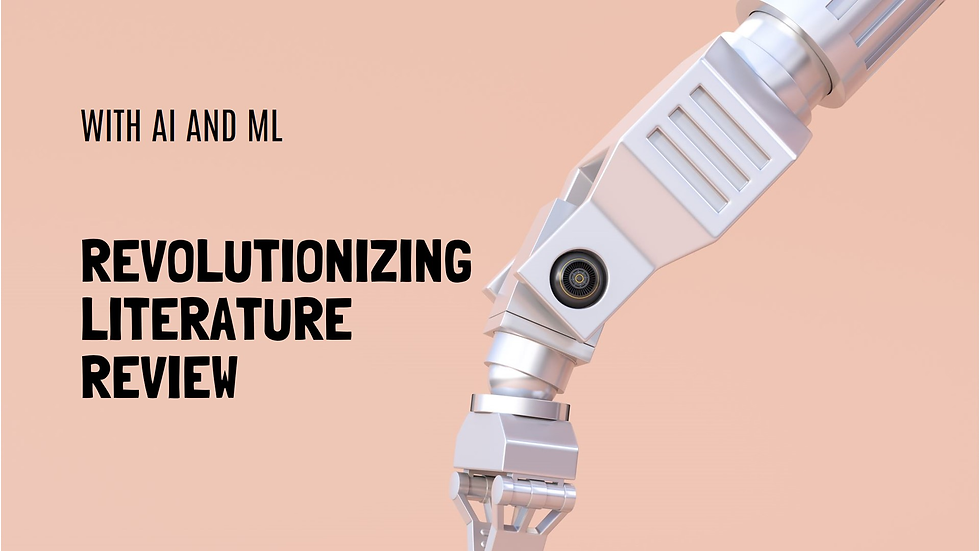
In an age of information overload, the ability to conduct efficient literature reviews has become increasingly vital across various fields, particularly in academia, healthcare, and pharmaceuticals. Traditional literature review methods often involve extensive manual searching, reading, and synthesizing of vast amounts of data, which can be time-consuming and prone to human error. However, the integration of Artificial Intelligence (AI) and Machine Learning (ML) into the literature review process is revolutionizing how researchers and organizations manage and synthesize information. This blog explores how AI and ML enhance literature review efficiency, transforming the landscape of research and compliance.
1. The Importance of Literature Reviews
Literature reviews are foundational in research, providing a comprehensive overview of existing knowledge on a specific topic. They serve several critical purposes, including:
Identifying Gaps in Knowledge: Literature reviews help researchers identify areas that require further exploration or inquiry.
Establishing Context: They provide background information that contextualizes new research findings within the existing body of knowledge.
Supporting Evidence-Based Practice: In fields like healthcare, literature reviews are crucial for ensuring that clinical practices are based on the best available evidence.
Informing Policy Decisions: Policymakers rely on literature reviews to understand the implications of research findings for public health and safety.
Given the increasing volume of published literature, the need for efficient and effective literature review methods has never been more pressing.
2. Challenges in Traditional Literature Reviews
Traditional literature reviews come with several challenges that can hinder efficiency, including:
Time-Consuming Processes: Manual searching through databases and reviewing articles can take weeks or even months, delaying research progress.
Information Overload: With thousands of new articles published daily, researchers may struggle to identify relevant studies amidst the noise.
Inconsistent Quality: Assessing the quality of studies can be subjective and inconsistent, leading to potential biases in the review.
Difficulty in Synthesis: Synthesizing findings from diverse studies with varying methodologies can be challenging and may result in overlooking critical insights.
These challenges underscore the need for innovative solutions that can streamline the literature review process.
3. The Role of AI and ML in Literature Reviews
AI and ML technologies are increasingly being adopted to enhance literature review efficiency. Here’s how these technologies can transform the review process:
3.1. Automated Literature Search
AI algorithms can automate the literature search process, significantly reducing the time and effort required to identify relevant studies. Key features include:
Natural Language Processing (NLP): NLP techniques enable AI systems to understand and interpret human language, allowing for more sophisticated search queries. Researchers can input questions or topics, and the AI can retrieve relevant articles based on context rather than just keyword matching.
Machine Learning Algorithms: These algorithms can learn from user interactions and feedback, continuously improving search accuracy over time. As researchers review articles and provide input on relevance, the AI refines its search criteria to better meet user needs.
By automating literature searches, researchers can focus on analyzing and synthesizing findings rather than spending excessive time on data collection.
3.2. Efficient Data Management
AI-powered literature review platforms offer robust data management features that streamline the organization and retrieval of articles. These features include:
Categorization and Tagging: AI systems can automatically categorize and tag articles based on keywords, topics, and relevance. This organization allows researchers to easily navigate their literature database and quickly locate relevant studies.
Citation Management: AI tools can manage citations and references, ensuring that researchers adhere to appropriate formatting styles and reduce the risk of citation errors.
Version Control: AI systems can track changes made to literature reviews over time, allowing researchers to manage multiple iterations and collaborate effectively with colleagues.
These data management capabilities enhance the overall efficiency of the literature review process, making it easier for researchers to maintain organized and up-to-date databases.
3.3. Quality Assessment and Screening
AI and ML technologies can assist in assessing the quality of studies included in literature reviews, ensuring that researchers focus on high-quality evidence. This process involves:
Automated Screening: AI algorithms can screen large volumes of literature for specific inclusion and exclusion criteria, rapidly identifying studies that meet predefined quality standards.
Predictive Analytics: By analyzing historical data, AI can predict the quality and relevance of studies based on factors such as journal impact, author credibility, and study design.
Bias Detection: Machine learning models can help identify potential biases in studies, alerting researchers to any concerns that may impact the validity of their review.
By automating the quality assessment process, researchers can ensure that their literature reviews are built on a solid foundation of reliable evidence.
3.4. Enhanced Synthesis and Analysis
One of the most significant advantages of AI and ML in literature reviews is their ability to synthesize and analyze data efficiently. Key functionalities include:
Text Mining: AI systems can analyze large volumes of text to identify patterns, themes, and relationships within the literature. This capability allows researchers to extract key findings and insights that may not be immediately apparent from individual studies.
Visualization Tools: Many AI-powered platforms offer data visualization features that help researchers present findings in an easily digestible format. Visual representations, such as graphs and charts, can highlight trends and relationships within the literature.
Meta-Analysis Support: AI can assist researchers in conducting meta-analyses by automating data extraction and statistical analysis, enabling them to combine findings from multiple studies effectively.
These synthesis and analysis capabilities empower researchers to draw meaningful conclusions from their literature reviews, enhancing the overall impact of their work.
4. Case Studies and Real-World Applications
Several organizations and researchers have successfully leveraged AI and ML to improve literature review efficiency. Here are a few notable examples:
4.1. Pharmaceutical Research
A pharmaceutical company focused on drug development implemented an AI-driven literature review platform to streamline its research process. By automating the literature search and quality assessment, the company reduced the time spent on literature reviews by 50%. This efficiency allowed the research team to allocate more time to data analysis and experimental design, ultimately accelerating the drug development timeline.
4.2. Academic Research
A team of academic researchers utilized an AI-powered literature review tool to conduct a systematic review of studies related to mental health interventions. The platform’s automated screening and data synthesis features enabled the team to complete their review in a fraction of the time it would have taken using traditional methods. The findings from the review significantly informed their subsequent research and publications.
4.3. Public Health
In a public health initiative, researchers employed AI algorithms to monitor and review literature related to emerging infectious diseases. By automating the literature search and quality assessment processes, the team could quickly identify relevant studies and track the latest developments in real time. This information was crucial for informing public health policies and responses during disease outbreaks.
5. Overcoming Challenges and Concerns
While the integration of AI and ML into literature reviews offers numerous benefits, some challenges and concerns must be addressed:
Data Privacy and Security: As with any technology, ensuring the privacy and security of sensitive research data is paramount. Organizations must implement robust data protection measures to safeguard information.
Bias in Algorithms: AI systems can inadvertently perpetuate biases present in training data. Researchers must remain vigilant and critically assess AI-generated results to ensure unbiased conclusions.
Integration with Existing Workflows: Organizations may face challenges integrating AI-powered tools into their established research workflows. Providing adequate training and support can help ease this transition.
6. The Future of Literature Reviews with AI and ML
The future of literature reviews is poised for significant transformation as AI and ML technologies continue to evolve. Some potential developments include:
Improved Personalization: Future AI systems may offer even more personalized literature review experiences, adapting to individual researcher preferences and focusing on specific areas of interest.
Enhanced Collaboration: AI-powered platforms could facilitate collaboration among researchers by allowing real-time sharing of insights and findings, regardless of geographic location.
Greater Interdisciplinary Insights: As AI algorithms become more sophisticated, they may be able to identify connections between studies across different disciplines, fostering interdisciplinary collaboration and innovation.
7. Conclusion
The integration of AI and ML into the literature review process represents a significant leap forward in research efficiency and effectiveness. By automating literature searches, enhancing data management, supporting quality assessment, and facilitating synthesis and analysis, these technologies empower researchers to conduct thorough and timely literature reviews.
As the landscape of research continues to evolve, embracing AI and ML tools will be crucial for researchers and organizations aiming to stay competitive and innovative. By harnessing the power of these technologies, researchers can streamline their literature review processes, allowing them to focus on what matters most: advancing knowledge and driving positive change in their respective fields.
Final Thoughts
In conclusion, AI and ML are not just enhancing literature review efficiency; they are reshaping the very fabric of research itself. As these technologies advance, they will unlock new possibilities for understanding complex issues, fostering collaboration, and driving evidence-based decision-making across disciplines. Embracing these innovations will be key to thriving in the rapidly evolving landscape of research and academia.
Comentários