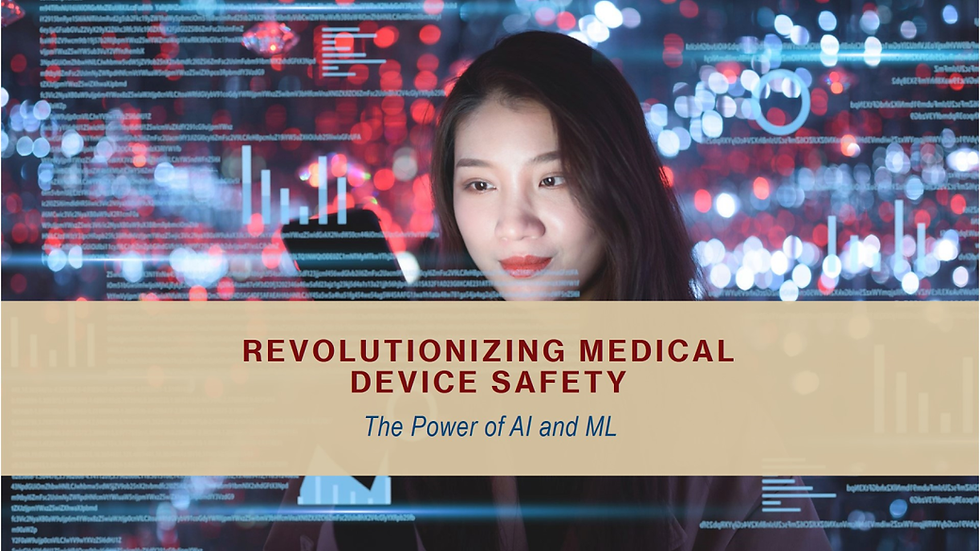
In the realm of healthcare, ensuring the safety and efficacy of medical devices is paramount. Every year, millions of medical devices are utilized worldwide to diagnose, treat, and monitor various medical conditions, ranging from pacemakers to insulin pumps and beyond. However, despite stringent regulations and rigorous testing procedures, adverse events related to medical devices can still occur, posing risks to patient safety. In this context, the integration of Artificial Intelligence (AI) and Machine Learning (ML) technologies has emerged as a game-changer, offering innovative solutions to enhance reporting accuracy and mitigate potential risks associated with medical devices.
The Significance of Accurate Reporting in Medical Device Safety:
Accurate reporting of adverse events associated with medical devices is crucial for several reasons. Firstly, it facilitates early detection of potential safety issues, allowing regulatory authorities and healthcare providers to take prompt corrective actions to minimize harm to patients. Secondly, comprehensive and precise data on adverse events serve as valuable inputs for post-market surveillance and risk assessment, enabling manufacturers to improve product design, labeling, and instructions for use. Moreover, transparent reporting fosters public trust and confidence in the healthcare system, ensuring that patients and healthcare professionals are well-informed about the risks and benefits of using specific medical devices.
However, traditional methods of adverse event reporting in healthcare settings are often labor-intensive, time-consuming, and prone to underreporting or inaccuracies. Manual reporting processes rely heavily on healthcare professionals to recognize, document, and submit adverse event reports, which can lead to delays and incomplete data capture. Furthermore, the subjective nature of human judgment may introduce variability and bias in reporting practices, hindering the timely identification of emerging safety concerns. In this context, the adoption of AI and ML technologies offers a promising solution to enhance the accuracy, efficiency, and reliability of medical device safety reporting.
Leveraging AI and ML for Enhanced Reporting:
Accuracy
AI and ML algorithms excel in processing vast amounts of data, identifying patterns, and extracting actionable insights with remarkable speed and accuracy. When applied to medical device safety reporting, these technologies can revolutionize the way adverse events are detected, documented, and analyzed. Here are some key ways in which AI and ML improve reporting accuracy in medical device safety:
Automated Detection of Adverse Events:
AI-powered algorithms can analyze electronic health records, medical device usage data, and other healthcare databases to automatically detect potential adverse events associated with specific devices. By continuously monitoring patient outcomes and device performance metrics in real-time, AI systems can flag anomalies or patterns indicative of adverse events, enabling early intervention and timely reporting.
Natural Language Processing (NLP) for Text Analysis:
NLP techniques enable computers to understand and interpret human language, making it easier to extract relevant information from unstructured text sources such as medical notes, incident reports, and social media posts. By parsing through free-text descriptions of adverse events, NLP algorithms can categorize and classify reports based on severity, clinical manifestations, and potential causal factors, facilitating standardized and consistent reporting practices.
Predictive Analytics for Risk Assessment:
ML models trained on historical data can predict the likelihood of adverse events occurring with specific medical devices based on patient demographics, device characteristics, and environmental factors. By quantifying the risk profile associated with different devices, predictive analytics empower healthcare providers and regulatory agencies to prioritize resources, allocate interventions, and implement targeted surveillance strategies to mitigate potential safety risks proactively.
Signal Detection and Pharmacovigilance:
AI-driven signal detection algorithms can sift through large-scale pharmacovigilance databases, such as the FDA Adverse Event Reporting System (FAERS), to identify statistically significant associations between medical devices and adverse events. By analyzing temporal trends, co-occurrence patterns, and data mining algorithms, these algorithms can distinguish genuine safety signals from background noise, enabling regulatory authorities to take appropriate regulatory actions, such as issuing safety alerts or conducting post-market investigations.
Human-in-the-Loop Validation:
While AI and ML algorithms offer tremendous capabilities for automating various aspects of medical device safety reporting, human oversight remains essential to ensure the quality and reliability of reported data. Through a human-in-the-loop approach, healthcare professionals can validate the outputs generated by AI systems, provide context-specific insights, and incorporate domain expertise into the decision-making process. By combining the strengths of AI-driven automation with human judgment, organizations can achieve a balance between efficiency and accuracy in medical device safety reporting.
Overcoming Challenges and Ensuring Ethical Use:
Despite their potential benefits, the integration of AI and ML technologies into medical device safety reporting also presents certain challenges and ethical considerations. Privacy concerns related to the collection and sharing of sensitive patient data must be addressed through robust data governance frameworks and adherence to regulatory requirements such as HIPAA (Health Insurance Portability and Accountability Act) in the United States. Moreover, algorithmic biases and limitations in generalizability may affect the reliability and validity of AI predictions, particularly in diverse patient populations or underrepresented communities.
To address these challenges, stakeholders must collaborate to develop transparent and accountable AI systems that prioritize patient safety, equity, and fairness. Rigorous validation and ongoing monitoring of AI algorithms are essential to ensure their reliability and performance across diverse healthcare settings. Furthermore, efforts to enhance data interoperability and standardization can facilitate seamless integration of AI-driven solutions into existing reporting infrastructure, enabling interoperability and knowledge sharing across healthcare organizations and regulatory agencies.
Conclusion:
In conclusion, AI and ML technologies hold immense promise for improving reporting accuracy in medical device safety by automating detection, analysis, and prediction of adverse events. By harnessing the power of data-driven insights, natural language processing, predictive analytics, and signal detection algorithms, stakeholders can enhance post-market surveillance, risk assessment, and regulatory decision-making processes. However, realizing the full potential of AI in medical device safety reporting requires careful consideration of ethical, legal, and technical challenges, as well as continued collaboration between industry, academia, and regulatory bodies. By embracing innovation while upholding patient-centered values and principles, we can leverage AI and ML to usher in a new era of enhanced safety and quality in healthcare delivery.
Comments