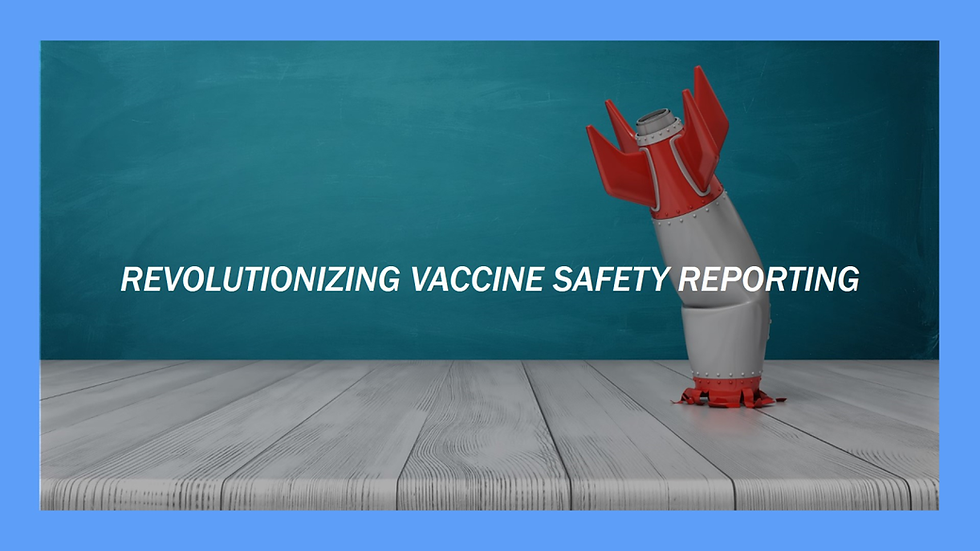
The introduction of artificial intelligence (AI) and machine learning (ML) in the field of vaccine safety has revolutionized how we monitor and ensure the safety of vaccines. These technologies have significantly enhanced the accuracy of reporting adverse events, streamlined data analysis, and improved overall public health outcomes. This blog explores the pivotal roles AI and ML play in improving reporting accuracy in vaccine safety.
The Importance of Accurate Vaccine Safety Reporting
Vaccines are among the most effective tools in preventing infectious diseases. However, ensuring their safety is paramount to maintaining public trust and achieving widespread immunization coverage. Accurate reporting of adverse events following immunization (AEFI) is critical for identifying potential safety issues, assessing the risk-benefit ratio of vaccines, and implementing appropriate public health measures.
Traditionally, vaccine safety monitoring relies on passive reporting systems, such as the Vaccine Adverse Event Reporting System (VAERS) in the United States. While these systems are crucial, they have inherent limitations, including underreporting, delayed reporting, and the potential for incomplete or inaccurate data. This is where AI and ML come into play, offering advanced solutions to enhance the accuracy and efficiency of vaccine safety monitoring.
AI and ML: Transformative Technologies in Vaccine Safety:
Enhanced Data Collection and Integration
One of the primary challenges in vaccine safety monitoring is the sheer volume and complexity of data. AI and ML technologies can process vast amounts of data from diverse sources, including electronic health records (EHRs), social media, clinical trials, and passive reporting systems. By integrating these data sources, AI and ML algorithms can provide a comprehensive view of vaccine safety.
For instance, natural language processing (NLP) algorithms can extract relevant information from unstructured text in EHRs and social media posts. This capability enables the identification of potential adverse events that might not be reported through traditional channels. Additionally, AI-powered chatbots can facilitate real-time reporting of adverse events by interacting with patients and healthcare providers, ensuring timely and accurate data collection.
Improved Signal Detection and Analysis:
Detecting safety signals—indications that a vaccine may be causing an adverse event—is a critical aspect of vaccine safety monitoring. Traditional methods often involve manual review and analysis, which can be time-consuming and prone to human error. AI and ML algorithms, on the other hand, excel at detecting patterns and anomalies in large datasets.
Machine learning models can be trained to identify associations between vaccines and adverse events by analyzing historical data. These models can then be used to continuously monitor new data for emerging signals. For example, Bayesian network models and other statistical learning techniques can evaluate the likelihood of a causal relationship between a vaccine and an adverse event, providing a more accurate and timely assessment of potential risks.
Enhanced Causality Assessment:
Determining whether a reported adverse event is causally related to a vaccine is a complex process that requires careful evaluation of evidence. AI and ML can assist in this process by automating and standardizing causality assessment frameworks.
For example, algorithms can be developed to apply the World Health Organization (WHO) causality assessment criteria to reported cases. These algorithms can analyze multiple factors, such as the temporal relationship between vaccination and the event, the consistency of the event with known vaccine-related adverse reactions, and the presence of alternative explanations. By doing so, AI and ML can provide more consistent and objective causality assessments, reducing variability and potential biases in the evaluation process.
Predictive Modeling and Risk Stratification:
AI and ML also enable predictive modeling, which can be used to identify individuals at higher risk of adverse events following vaccination. By analyzing patient characteristics, medical history, and genetic information, ML algorithms can predict the likelihood of an adverse event occurring in a specific individual.
These predictive models can inform personalized vaccination strategies, allowing healthcare providers to tailor vaccination plans based on individual risk profiles. For example, individuals identified as high-risk could be monitored more closely after vaccination or receive vaccines with a lower risk of adverse events. This approach not only enhances vaccine safety but also promotes confidence in vaccination programs by addressing individual concerns and needs.
Case Studies: AI and ML in Action:
The COVID-19 Vaccine Rollout
The rapid development and deployment of COVID-19 vaccines highlighted the need for robust vaccine safety monitoring systems. AI and ML played a crucial role in this unprecedented global vaccination effort.
For instance, the U.S. Food and Drug Administration (FDA) and the Centers for Disease Control and Prevention (CDC) implemented the Vaccine Safety Datalink (VSD) project, which utilized AI and ML to monitor the safety of COVID-19 vaccines in near real-time. By analyzing EHRs and claims data from participating healthcare organizations, the VSD project was able to quickly identify and investigate potential safety signals, ensuring timely responses to emerging safety concerns.
Additionally, AI-driven platforms like V-safe, a smartphone-based tool, enabled individuals to report their health status after receiving a COVID-19 vaccine. This tool collected vast amounts of data directly from vaccine recipients, which was then analyzed using ML algorithms to detect patterns and identify potential adverse events.
Pharmacovigilance in Routine Immunization Programs:
AI and ML are not limited to pandemic situations; they are also transforming routine immunization programs. The Global Vaccine Safety Initiative (GVSI), led by the WHO, has been exploring the use of AI and ML to enhance pharmacovigilance systems worldwide.
In countries with limited resources, traditional pharmacovigilance systems often struggle to keep up with the volume of data generated by vaccination programs. AI and ML offer scalable solutions to address these challenges. For example, machine learning algorithms can automate the classification and analysis of adverse event reports, reducing the burden on human analysts and improving the efficiency of safety monitoring.
In India, the Integrated Health Information Platform (IHIP) leverages AI and ML to monitor vaccine safety across the country. By integrating data from various sources, including EHRs, laboratory results, and passive reporting systems, the IHIP provides a comprehensive view of vaccine safety. ML algorithms analyze this data to identify potential safety signals and support timely decision-making by public health authorities.
Challenges and Future Directions:
While AI and ML offer tremendous potential in improving vaccine safety reporting, several challenges must be addressed to fully realize their benefits.
Data Quality and Standardization:
The accuracy and reliability of AI and ML algorithms depend on the quality and consistency of the data they analyze. Ensuring high-quality data collection, standardizing data formats, and addressing data privacy concerns are critical steps in implementing effective AI-driven vaccine safety monitoring systems.
Algorithm Transparency and Explain ability:
AI and ML algorithms can be complex and often function as "black boxes," making it difficult to understand how they arrive at specific conclusions. Ensuring transparency and explain ability in these algorithms is essential for building trust among healthcare providers, regulators, and the public. Efforts to develop interpretable AI models and provide clear explanations of their decision-making processes are crucial.
Ethical Considerations:
The use of AI and ML in vaccine safety monitoring raises important ethical considerations, including issues related to data privacy, informed consent, and algorithmic bias. Ensuring that AI-driven systems are developed and implemented ethically, with a focus on equity and fairness, is essential to avoid exacerbating existing health disparities.
Continuous Monitoring and Updating:
AI and ML models must be continuously monitored and updated to adapt to changing data and evolving vaccine safety profiles. Regular validation and recalibration of these models are necessary to ensure their ongoing accuracy and effectiveness.
Conclusion:
AI and ML have emerged as powerful tools in improving reporting accuracy in vaccine safety. By enhancing data collection, signal detection, causality assessment, and predictive modeling, these technologies offer significant advantages over traditional methods. The successful application of AI and ML during the COVID-19 vaccine rollout and in routine immunization programs demonstrates their potential to transform vaccine safety monitoring on a global scale.
As we continue to advance AI and ML technologies, it is crucial to address the challenges and ethical considerations associated with their use. By doing so, we can harness the full potential of these technologies to ensure the safety of vaccines, protect public health, and build confidence in vaccination programs worldwide. The future of vaccine safety monitoring is undoubtedly bright, with AI and ML paving the way for more accurate, efficient, and responsive systems.
Comments