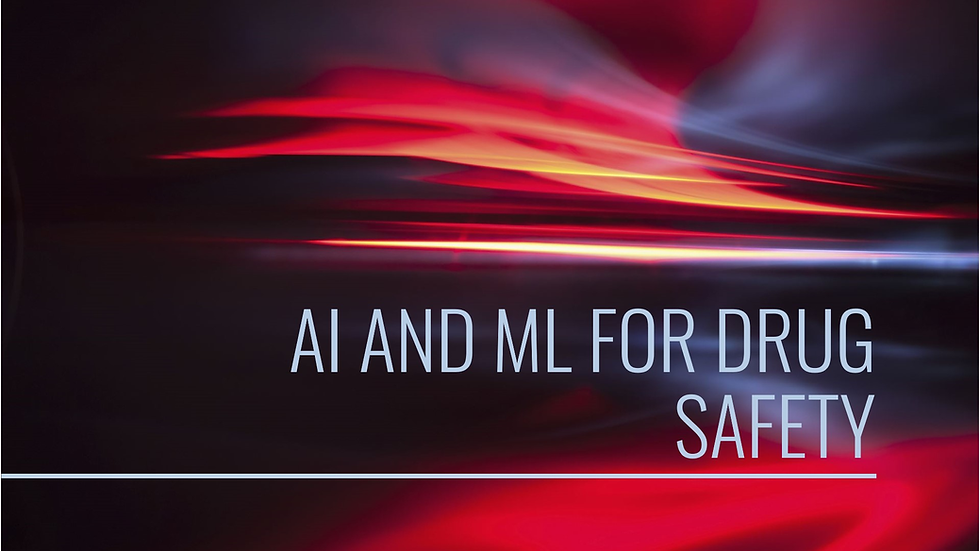
In the dynamic landscape of drug safety, the management of safety signals has become a critical focus for regulatory authorities, pharmaceutical companies, and healthcare providers. Safety signals refer to information that suggests a potential causal relationship between a drug and an adverse event, prompting further investigation. Traditionally, signal detection and management have relied heavily on manual processes, which can be time-consuming and prone to errors. However, the integration of Artificial Intelligence (AI) and Machine Learning (ML) is revolutionizing how safety signals are identified, evaluated, and managed. This blog explores how AI and ML enhance safety signal management in drug safety, leading to improved patient outcomes and regulatory compliance.
1. Understanding Safety Signals in Drug Safety
Safety signals arise from various sources, including spontaneous adverse event reports, clinical trials, and epidemiological studies. These signals play a crucial role in pharmacovigilance—the science and activities related to the detection, assessment, understanding, and prevention of adverse effects or any other drug-related problems. The effective management of safety signals is essential for:
Protecting Patient Safety: Timely detection and management of safety signals can prevent harm to patients and ensure that potential risks are communicated to healthcare professionals and the public.
Regulatory Compliance: Pharmaceutical companies are required to report safety signals to regulatory authorities, ensuring that they comply with legal and ethical standards.
Informed Decision-Making: Accurate signal management allows healthcare providers to make informed decisions regarding the use of medications, improving patient care.
Despite its importance, the traditional approaches to signal detection and management have several limitations that AI and ML can effectively address.
2. Challenges in Traditional Safety Signal Management
Traditional safety signal management approaches face several challenges, including:
Data Overload: With the increasing volume of adverse event reports and clinical data, manually sifting through vast amounts of information can overwhelm safety teams, leading to delays in signal detection.
Subjectivity in Signal Evaluation: Human evaluators may have different interpretations of data, leading to inconsistencies in signal identification and management.
Time-Consuming Processes: The manual processes involved in signal detection, evaluation, and reporting can take significant time, delaying necessary actions to protect patient safety.
Limited Predictive Capability: Traditional methods may struggle to identify emerging safety signals that have not yet been widely recognized, potentially leaving patients at risk.
Given these challenges, the adoption of AI and ML technologies in safety signal management represents a significant advancement in the field of pharmacovigilance.
3. The Role of AI and ML in Safety Signal Management
AI and ML technologies offer innovative solutions that can enhance safety signal management processes in several key ways:
3.1. Automated Signal Detection
AI algorithms can analyze large datasets from various sources, including electronic health records, social media, and clinical trial data, to detect safety signals automatically. Key features include:
Natural Language Processing (NLP): NLP techniques allow AI systems to understand and interpret unstructured text data, such as clinical notes and patient reports. This capability enables the identification of relevant safety signals that may be buried within vast amounts of textual information.
Pattern Recognition: Machine learning algorithms can identify patterns and trends in data that may indicate potential safety signals. By learning from historical data, these algorithms can refine their detection capabilities over time.
Real-Time Monitoring: AI-powered systems can continuously monitor incoming data for safety signals, enabling timely detection and response to emerging concerns.
Automated signal detection significantly reduces the time and effort required for manual reviews, allowing safety teams to focus on evaluating and managing identified signals.
3.2. Enhanced Signal Evaluation
Once a safety signal is detected, AI and ML can facilitate a more efficient and objective evaluation process. This includes:
Data Integration: AI systems can aggregate data from multiple sources, including clinical trials, spontaneous reports, and electronic health records, providing a comprehensive view of the safety signal. This integration allows for more informed decision-making.
Predictive Analytics: Machine learning models can predict the likelihood of adverse events based on historical data and identify risk factors associated with specific drugs. These predictive capabilities can help prioritize signals for further investigation.
Automated Causality Assessment: AI algorithms can assist in determining the causal relationship between a drug and an adverse event by analyzing relevant data. This automation reduces subjectivity and variability in causality assessments.
By enhancing the signal evaluation process, AI and ML contribute to more accurate and timely assessments of potential safety concerns.
3.3. Improved Risk Communication
Effective communication of safety signals is essential for ensuring that healthcare professionals and patients are aware of potential risks associated with medications. AI and ML can improve risk communication in the following ways:
Tailored Communication Strategies: AI systems can analyze the preferences and behaviors of target audiences to develop tailored communication strategies. This personalization ensures that messages about safety signals reach the right stakeholders in a timely manner.
Real-Time Alerts: AI-powered platforms can generate real-time alerts for healthcare providers regarding newly identified safety signals, enabling them to make informed decisions about patient care.
Risk Visualization: Data visualization tools powered by AI can present safety signal data in an easily digestible format, allowing stakeholders to grasp complex information quickly.
Improved risk communication ensures that relevant parties are informed about safety signals and can take appropriate actions to mitigate risks.
3.4. Continuous Learning and Adaptation
One of the most significant advantages of AI and ML in safety signal management is their ability to learn and adapt over time. Key features include:
Feedback Loops: AI systems can incorporate feedback from safety evaluations and outcomes to improve their detection and evaluation algorithms continuously. This iterative learning process enhances the accuracy and reliability of signal management.
Identification of Emerging Signals: Machine learning models can identify emerging safety signals that may not have been recognized through traditional methods, allowing for proactive risk management.
Longitudinal Analysis: AI can analyze data trends over time, providing insights into the long-term safety profile of medications and identifying potential issues before they become widespread.
This continuous learning capability ensures that safety signal management processes remain current and effective in addressing evolving drug safety concerns.
4. Case Studies and Real-World Applications
Several organizations have successfully integrated AI and ML into their safety signal management processes. Here are a few notable examples:
4.1. Pharmaceutical Industry
A leading pharmaceutical company implemented an AI-driven signal detection platform to enhance its pharmacovigilance operations. By automating the detection of safety signals from spontaneous reports and clinical trial data, the company reduced its signal detection time by 60%. This efficiency allowed the safety team to focus on evaluating and managing identified signals, ultimately improving patient safety outcomes.
4.2. Regulatory Authorities
A regulatory authority adopted AI algorithms to monitor social media platforms for adverse drug reactions. By analyzing real-time data from social media, the authority was able to identify safety signals that emerged outside of traditional reporting channels. This proactive approach led to timely warnings and risk communication to healthcare professionals and the public.
4.3. Clinical Research
A clinical research organization (CRO) utilized machine learning algorithms to enhance the safety monitoring of clinical trials. By integrating data from electronic health records and trial databases, the CRO improved its ability to identify potential safety signals early in the trial process. This capability allowed for more informed decision-making regarding patient safety and trial adjustments.
5. Overcoming Challenges and Concerns
While the integration of AI and ML into safety signal management offers numerous benefits, several challenges and concerns must be addressed:
Data Quality and Consistency: The effectiveness of AI algorithms relies on the quality and consistency of the data being analyzed. Ensuring that data is accurate and representative is crucial for reliable signal detection and evaluation.
Regulatory Compliance: As AI and ML technologies evolve, regulatory authorities will need to establish guidelines and standards for their use in safety signal management. Compliance with these regulations is essential to maintain public trust.
Transparency and Explainability: The "black box" nature of some AI algorithms raises concerns about transparency and explainability. Ensuring that stakeholders understand how AI systems make decisions is vital for building confidence in these technologies.
Ethical Considerations: The use of AI and ML in safety signal management raises ethical questions regarding data privacy, consent, and the potential for bias in algorithmic decision-making. Addressing these concerns is essential for responsible AI deployment.
6. The Future of Safety Signal Management with AI and ML
The future of safety signal management is poised for significant advancements as AI and ML technologies continue to evolve. Some potential developments include:
Integration with Real-World Evidence: The incorporation of real-world evidence (RWE) into safety signal management processes will enhance the understanding of drug safety in diverse patient populations and real-world settings.
Collaboration Between Stakeholders: Enhanced collaboration between pharmaceutical companies, regulatory authorities, and healthcare providers will facilitate more effective signal management and risk communication.
Proactive Risk Management: As AI algorithms become more sophisticated, they will enable proactive risk management strategies, allowing for early identification and mitigation of potential safety concerns.
7. Conclusion
The integration of AI and ML into safety signal management represents a transformative shift in pharmacovigilance practices. By automating signal detection, enhancing evaluation processes, improving risk communication, and enabling continuous learning, these technologies empower organizations to manage safety signals more effectively and efficiently.
As the landscape of drug safety continues to evolve, embracing AI and ML tools will be crucial for ensuring patient safety, regulatory compliance, and informed decision-making. By harnessing the power of these technologies, stakeholders can proactively address safety concerns and enhance the overall quality of drug safety practices.
Final Thoughts
In conclusion, AI and ML are not just enhancing safety signal management; they are reshaping the entire paradigm of drug safety. As these technologies advance, they will unlock new possibilities for understanding and mitigating risks associated with medications, ultimately leading to improved patient outcomes and
Comentários