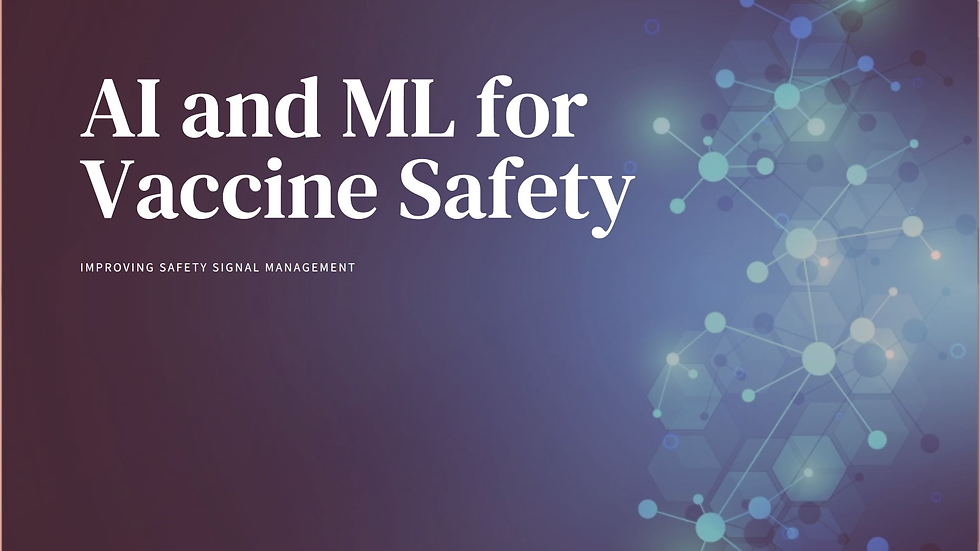
advent of artificial intelligence (AI) and machine learning (ML) has revolutionized numerous fields, and vaccine safety management is no exception. These technologies are proving indispensable in the intricate process of monitoring, assessing, and managing safety signals related to vaccines. As vaccine administration becomes more widespread, optimizing safety signal management is crucial to ensure public health and confidence in vaccination programs. This blog delves into how AI and ML improve safety signal management in vaccine safety, highlighting their roles, benefits, and implementation strategies.
Understanding Safety Signal Management:
Before exploring the impact of AI and ML, it's essential to grasp what safety signal management entails. Safety signals refer to information indicating potential new or previously unrecognized risks associated with a vaccine. Signal management involves:
Signal Detection: Identifying potential safety signals from data sources such as clinical trials, post-marketing surveillance, and spontaneous reports.
Signal Assessment: Evaluating the significance of detected signals by analyzing their frequency, severity, and potential causal relationship with the vaccine.
Signal Prioritization: Determining which signals require immediate attention based on their potential impact on public health.
Risk Communication: Communicating findings and actions to stakeholders, including regulatory authorities, healthcare professionals, and the public.
Risk Mitigation: Implementing strategies to minimize or manage identified risks, which may involve updating vaccine guidelines, labeling changes, or additional research.
The Role of AI and ML in Safety Signal Management:
AI and ML enhance safety signal management by automating and refining the processes involved. Here’s how these technologies contribute:
1. Enhanced Signal Detection
AI and ML Algorithms: Machine learning algorithms can process vast amounts of data from diverse sources, such as electronic health records (EHRs), vaccine registries, and social media. These algorithms use pattern recognition to identify potential safety signals that might be missed by traditional methods. For instance, ML models can analyze trends and anomalies in adverse event reports to flag unusual patterns.
Natural Language Processing (NLP): NLP, a subset of AI, enables the extraction of relevant information from unstructured data sources. This includes free-text reports from patients and healthcare providers, social media posts, and scientific literature. NLP algorithms can identify and categorize mentions of adverse events, enhancing the breadth of signal detection.
Predictive Analytics: AI-driven predictive analytics can forecast potential safety signals before they become widespread issues. By analyzing historical data and current trends, these models can predict emerging safety concerns, allowing for early intervention.
2. Improved Signal Assessment
Automated Data Analysis: AI tools can automate the assessment of safety signals by analyzing large datasets for patterns and correlations. This reduces the time and effort required for manual analysis and helps identify significant signals more quickly.
Causality Assessment: ML models can assist in determining the causal relationship between a vaccine and adverse events. By analyzing data from various sources and applying statistical methods, these models can provide insights into whether a signal is likely related to the vaccine or coincidental.
Data Integration: AI can integrate data from disparate sources, such as clinical trials, post-marketing surveillance, and patient records. This comprehensive view enhances the accuracy of signal assessment by providing a more complete picture of the potential risk.
3. Efficient Signal Prioritization
Risk Scoring Models: AI-driven risk scoring models can prioritize signals based on their potential impact and likelihood. These models use historical data and risk factors to assign scores to different signals, helping regulators and safety officers focus on the most critical issues.
Resource Allocation: AI can optimize resource allocation by identifying which signals require immediate investigation and which can be monitored over time. This ensures that resources are directed toward the most pressing safety concerns.
Dynamic Prioritization: ML models can adjust signal prioritization dynamically based on real-time data and emerging trends. This flexibility allows for a more responsive approach to managing safety signals.
4. Effective Risk Communication
Automated Reporting: AI systems can automate the generation of reports and summaries related to safety signals. These reports can be tailored to different audiences, including regulatory authorities, healthcare professionals, and the public, ensuring that relevant information is communicated effectively.
Real-Time Alerts: AI can provide real-time alerts and notifications about emerging safety signals. This ensures that stakeholders are promptly informed of new developments and can take appropriate actions.
Visualization Tools: Advanced AI tools offer visualization capabilities, such as interactive dashboards and graphical representations of safety data. These tools help stakeholders understand complex data and trends, facilitating better decision-making and communication.
5. Strategic Risk Mitigation
Actionable Insights: AI and ML provide actionable insights that inform risk mitigation strategies. By analyzing safety signals and their potential impact, these technologies can suggest targeted interventions, such as updating vaccine guidelines or implementing additional safety measures.
Simulation and Modeling: AI can simulate different risk scenarios and model the potential impact of various mitigation strategies. This helps in evaluating the effectiveness of proposed actions and selecting the most appropriate risk management strategies.
Continuous Monitoring: AI systems enable continuous monitoring of safety signals and the effectiveness of mitigation measures. This ongoing assessment ensures that risks are managed proactively and adjustments are made as needed.
Case Studies and Examples:
To illustrate the impact of AI and ML in vaccine safety signal management, let’s explore a few real-world examples:
1. The Case of the COVID-19 Vaccines
During the COVID-19 pandemic, AI and ML played a critical role in managing safety signals related to vaccines. For example:
Signal Detection: ML algorithms analyzed millions of adverse event reports from various sources, including EHRs and social media, to identify potential safety concerns related to COVID-19 vaccines.
Risk Assessment: AI-driven models evaluated the causality of adverse events reported after vaccination, helping to determine whether they were related to the vaccines or coincidental.
Real-Time Monitoring: AI tools provided real-time monitoring and analysis of vaccine safety data, enabling rapid response to emerging safety signals.
2. The Role of NLP in Vaccine Safety
Natural Language Processing has been instrumental in analyzing unstructured data sources:
Adverse Event Extraction: NLP algorithms extracted information about adverse events from free-text reports, social media posts, and scientific literature, enhancing signal detection capabilities.
Trend Analysis: NLP tools analyzed trends and patterns in adverse event reports, providing insights into potential safety concerns and informing risk management strategies.
Implementation Strategies:
To harness the full potential of AI and ML in safety signal management, organizations should consider the following implementation strategies:
1. Data Quality and Integration
Ensuring high-quality, integrated data is crucial for effective AI and ML applications. Organizations should focus on:
Data Cleaning: Regularly clean and validate data to ensure accuracy and consistency.
Data Integration: Integrate data from various sources to provide a comprehensive view of safety signals.
2. Collaboration and Expertise
Collaboration with AI and ML experts is essential for successful implementation:
Partnerships: Partner with technology providers and research institutions to leverage their expertise and resources.
Training: Train staff in AI and ML technologies to ensure effective use and interpretation of these tools.
3. Regulatory Compliance
Adhering to regulatory requirements is crucial when implementing AI and ML in safety signal management:
Guidelines: Follow regulatory guidelines for the use of AI and ML in pharmacovigilance and vaccine safety.
Validation: Validate AI and ML models to ensure they meet regulatory standards and provide reliable results.
4. Continuous Improvement
Continuous improvement is key to maximizing the benefits of AI and ML:
Feedback Loops: Establish feedback loops to refine AI and ML models based on real-world performance and user input.
Innovation: Stay updated on advancements in AI and ML technologies and incorporate new innovations to enhance safety signal management.
Conclusion:
AI and ML are transforming safety signal management in vaccine safety by enhancing signal detection, improving assessment and prioritization, and facilitating effective risk communication and mitigation. These technologies provide valuable tools for managing the complex and evolving landscape of vaccine safety, ultimately contributing to better public health outcomes. As AI and ML continue to advance, their integration into safety signal management will become even more crucial in ensuring the safety and efficacy of vaccines worldwide. Embracing these technologies and implementing them effectively will be key to addressing emerging safety challenges and maintaining public trust in vaccination programs.
Comments