How AI and ML Simplify Case Processing for Medical Devices
- Chaitali Gaikwad
- Jun 3, 2024
- 6 min read
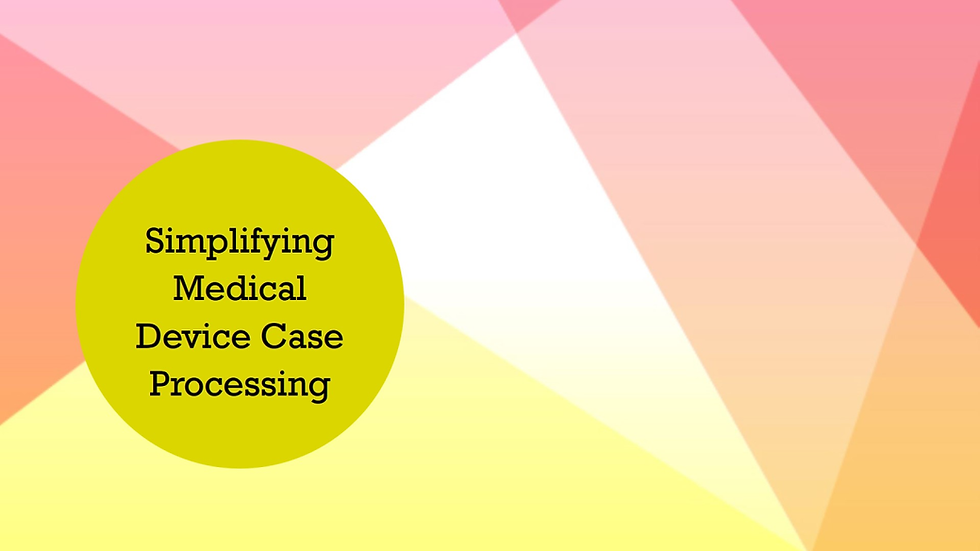
The integration of artificial intelligence (AI) and machine learning (ML) in the healthcare sector has revolutionized various processes, including the case processing for medical devices. These technologies offer unprecedented efficiency, accuracy, and predictive capabilities, transforming how medical device cases are managed from development through post-market surveillance. This blog explores the myriad ways AI and ML simplify case processing for medical devices, enhancing safety, compliance, and innovation.
The Role of AI and ML in Medical Device Case Processing:
Medical device case processing encompasses a range of activities, from adverse event reporting and investigation to regulatory submissions and post-market surveillance. AI and ML technologies are proving to be invaluable in streamlining these processes through automation, pattern recognition, predictive analytics, and data integration.
1. Automation of Routine Tasks
One of the primary ways AI and ML simplify case processing is through the automation of routine and repetitive tasks. Traditionally, case processing involves significant manual effort, including data entry, sorting, and initial analysis. AI-driven automation can handle these tasks more quickly and with fewer errors.
For example, natural language processing (NLP) algorithms can extract relevant information from unstructured data sources such as clinical notes, adverse event reports, and customer feedback. This automated data extraction accelerates the initial processing stages, allowing human experts to focus on more complex and critical aspects of case management.
2. Enhanced Data Analysis and Pattern Recognition
Medical device case processing generates vast amounts of data, which can be challenging to analyze manually. AI and ML algorithms excel at analyzing large datasets to identify patterns, correlations, and anomalies that might not be immediately apparent to human analysts.
Machine learning models can be trained to recognize patterns in adverse event reports, helping to identify potential safety issues earlier. For instance, clustering algorithms can group similar adverse events, revealing trends that warrant further investigation. This capability is particularly valuable in post-market surveillance, where early detection of emerging risks is crucial for patient safety.
3. Predictive Analytics for Risk Assessment
Predictive analytics powered by AI and ML can transform risk assessment in medical device case processing. By analyzing historical data and identifying trends, these technologies can predict potential future events, allowing for proactive risk management.
For example, predictive models can estimate the likelihood of device failures based on historical performance data and external factors such as usage patterns and patient demographics. This foresight enables manufacturers to implement preventive measures, such as design modifications or targeted recalls, before widespread issues occur.
Streamlining Adverse Event Reporting:
Adverse event reporting is a critical component of medical device case processing, ensuring that potential safety concerns are identified and addressed promptly. AI and ML streamline this process by improving the accuracy, timeliness, and completeness of adverse event reports.
1. Improving Report Quality
The quality of adverse event reports can vary significantly, impacting the ability to detect and address safety issues effectively. AI and ML technologies can enhance report quality by standardizing data entry and flagging incomplete or inconsistent information.
For instance, NLP algorithms can parse adverse event narratives to extract key details, ensuring that reports contain all necessary information. Additionally, AI can cross-reference new reports with existing data to identify discrepancies and prompt further investigation if needed.
2. Accelerating Report Processing
Traditionally, adverse event reports undergo a lengthy manual review process, delaying the identification of critical safety issues. AI-driven automation can accelerate this process by triaging reports based on severity and relevance.
Machine learning models can prioritize reports that indicate serious or unusual events, ensuring that they receive immediate attention. This rapid triage allows regulatory authorities and manufacturers to respond more swiftly to potential safety concerns, reducing the risk of harm to patients.
Enhancing Regulatory Submissions and Compliance:
Regulatory compliance is a complex and time-consuming aspect of medical device case processing. AI and ML simplify regulatory submissions and compliance by automating document preparation, ensuring adherence to regulatory standards, and facilitating communication with regulatory bodies.
1. Automating Document Preparation
Preparing regulatory submissions involves compiling extensive documentation, including clinical trial data, safety reports, and manufacturing information. AI-powered tools can automate the assembly of these documents, reducing the time and effort required.
For example, AI can extract relevant information from various sources and organize it according to regulatory requirements. This automation ensures that submissions are complete and formatted correctly, minimizing the risk of delays or rejections due to documentation errors.
2. Ensuring Regulatory Adherence
Regulatory requirements for medical devices vary across different regions and can change frequently. AI and ML can help manufacturers stay compliant by monitoring regulatory changes and updating processes accordingly.
AI-driven compliance management systems can track regulatory updates and automatically adjust internal procedures to align with new requirements. This proactive approach ensures that manufacturers remain compliant without the need for constant manual oversight.
3. Facilitating Communication with Regulatory Bodies
Effective communication with regulatory authorities is essential for timely approvals and ongoing compliance. AI-powered chatbots and virtual assistants can facilitate this communication by providing instant responses to regulatory inquiries and guiding manufacturers through the submission process.
For instance, AI-driven systems can generate real-time updates on the status of regulatory submissions and answer common questions from regulatory authorities. This streamlined communication enhances transparency and reduces the time required for regulatory interactions.
Improving Post-Market Surveillance:
Post-market surveillance is crucial for monitoring the ongoing safety and performance of medical devices. AI and ML enhance post-market surveillance by enabling continuous monitoring, real-time data analysis, and proactive risk management.
1. Continuous Monitoring and Real-Time Analysis
Traditional post-market surveillance relies on periodic reporting and manual data analysis, which can result in delayed detection of safety issues. AI and ML enable continuous monitoring of device performance and real-time analysis of data from various sources.
For example, AI algorithms can analyze data from electronic health records, patient registries, and social media to identify potential safety signals. This real-time surveillance allows for immediate action in response to emerging risks, improving patient safety and device reliability.
2. Proactive Risk Management
AI and ML technologies can predict potential safety issues before they become widespread, allowing for proactive risk management. Predictive models can assess the likelihood of adverse events based on historical data and current trends, enabling manufacturers to implement preventive measures.
For instance, if an AI model predicts a high risk of device failure in a specific patient population, manufacturers can conduct targeted recalls or provide additional training to healthcare providers. This proactive approach minimizes the impact of safety issues and enhances overall device performance.
Challenges and Future Directions:
While AI and ML offer significant benefits for medical device case processing, their implementation is not without challenges. Addressing these challenges is essential to fully realize the potential of these technologies.
1. Data Quality and Integration
The effectiveness of AI and ML depends on the quality and completeness of the data used for training and analysis. Ensuring that data is accurate, comprehensive, and well-integrated across different systems is a significant challenge.
Manufacturers and regulatory authorities must invest in robust data management practices, including data standardization, validation, and integration. High-quality data is essential for training reliable AI models and deriving actionable insights.
2. Regulatory Acceptance and Ethical Considerations
The use of AI and ML in medical device case processing raises regulatory and ethical considerations. Regulatory bodies must establish clear guidelines for the use of these technologies, ensuring that they meet safety and efficacy standards.
Additionally, ethical considerations such as data privacy, algorithmic bias, and transparency must be addressed. Manufacturers and regulators must work together to develop ethical frameworks that ensure AI and ML are used responsibly and equitably.
3. Continuous Learning and Adaptation
AI and ML technologies must continuously learn and adapt to evolving data and regulatory environments. Implementing continuous learning frameworks and updating models regularly is crucial for maintaining their effectiveness.
Manufacturers must establish processes for ongoing model training, validation, and updating. This iterative approach ensures that AI and ML technologies remain relevant and accurate in dynamic regulatory and clinical landscapes.
Conclusion:
AI and ML are transforming medical device case processing, offering unprecedented efficiency, accuracy, and predictive capabilities. By automating routine tasks, enhancing data analysis, and enabling proactive risk management, these technologies simplify the complex processes involved in managing medical device cases.
From adverse event reporting and regulatory submissions to post-market surveillance, AI and ML are driving significant improvements in safety, compliance, and innovation. However, realizing the full potential of these technologies requires addressing challenges related to data quality, regulatory acceptance, and ethical considerations.
As the healthcare sector continues to embrace AI and ML, the future of medical device case processing looks promising. By leveraging these advanced technologies, manufacturers and regulatory authorities can enhance patient safety, streamline compliance, and foster innovation in the medical device industry.
Комментарии