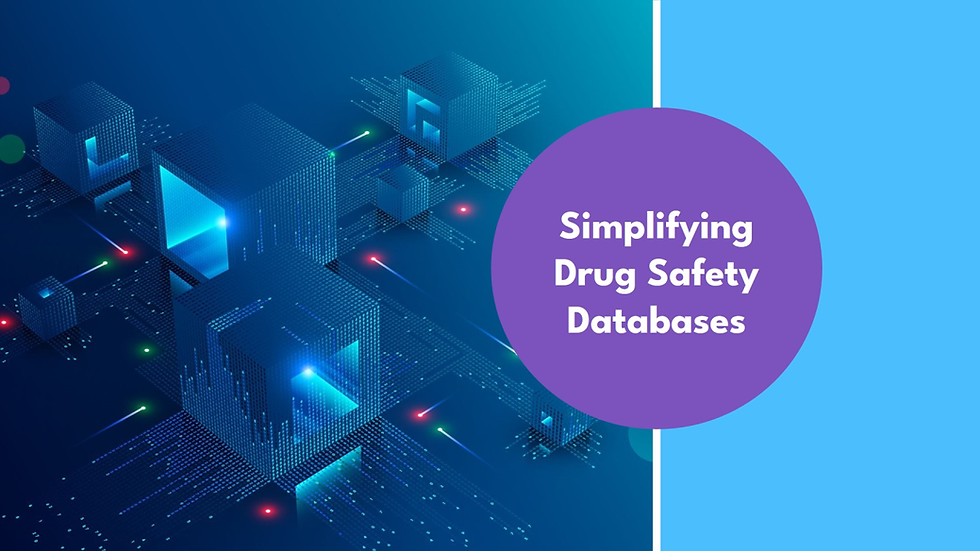
The advent of Artificial Intelligence (AI) and Machine Learning (ML) has revolutionized numerous industries, and the pharmaceutical sector is no exception. Among the various applications of these technologies in the pharma industry, one of the most impactful is their role in simplifying case processing in drug safety databases. This blog will delve into how AI and ML are transforming drug safety monitoring, also known as pharmacovigilance, and enhancing the efficiency, accuracy, and overall management of drug safety data.
Drug safety databases, also referred to as pharmacovigilance databases, are critical tools used by pharmaceutical companies and regulatory bodies to collect, analyze, and monitor adverse drug reactions (ADRs). These databases compile data from various sources, including clinical trials, post-marketing surveillance, healthcare professionals, and patients, to ensure that drugs on the market are safe for public use.
Traditionally, the processing of this data has been a labor-intensive and time-consuming task. It involves the collection of reports, manual data entry, assessment of causality, and regulatory reporting. Given the volume and complexity of data, there is always a risk of human error, which can lead to significant consequences, including delayed identification of safety signals and non-compliance with regulatory requirements.
AI and ML technologies are uniquely suited to address the challenges associated with traditional pharmacovigilance. They offer the ability to process vast amounts of data quickly and accurately, identify patterns, predict outcomes, and automate routine tasks. Here’s how AI and ML are simplifying case processing in drug safety databases:
1. Automated Data Entry and Preprocessing
One of the primary benefits of AI and ML is the automation of data entry and preprocessing tasks. Natural Language Processing (NLP), a subset of AI, can be used to extract relevant information from various unstructured data sources such as clinical notes, social media posts, and patient records. NLP algorithms can identify and categorize adverse event reports, reducing the need for manual data entry and minimizing the risk of human error.
For example, an NLP system can scan through thousands of patient reports to identify mentions of specific symptoms or adverse reactions, categorize these mentions, and enter the relevant information into the database. This not only speeds up the data entry process but also ensures a higher degree of accuracy and consistency.
2. Enhanced Signal Detection
Signal detection is a critical aspect of pharmacovigilance, involving the identification of new or rare adverse drug reactions that were not detected during clinical trials. Traditional methods of signal detection rely heavily on statistical techniques and manual review, which can be time-consuming and may miss subtle patterns.
AI and ML algorithms can significantly enhance signal detection by analyzing large datasets to identify patterns and correlations that might be indicative of a safety signal. Machine learning models can be trained to recognize the characteristics of known adverse reactions and apply this knowledge to identify potential new signals. These models can also continuously learn and improve over time as more data becomes available, leading to more accurate and timely detection of safety issues.
3. Predictive Analytics
Predictive analytics, powered by AI and ML, can forecast potential safety issues before they become apparent. By analyzing historical data, machine learning models can predict the likelihood of certain adverse reactions occurring in specific patient populations. This predictive capability allows pharmaceutical companies to proactively address potential safety concerns, improving patient outcomes and reducing the risk of regulatory non-compliance.
For instance, predictive models can help identify patient subgroups that are at higher risk of experiencing adverse reactions based on factors such as age, gender, genetic markers, and comorbidities. This information can be used to tailor treatment plans, implement additional monitoring, or adjust dosing recommendations to enhance patient safety.
4. Case Triage and Prioritization
Not all adverse event reports are of equal importance; some require immediate attention, while others may be less urgent. AI and ML can assist in the triage and prioritization of cases by automatically categorizing reports based on their severity and potential impact. Machine learning algorithms can analyze the content of each report, assess its seriousness, and prioritize cases that need immediate investigation.
This automated triage system ensures that critical cases are addressed promptly, reducing the time to action for serious safety issues. It also helps to manage the workload of pharmacovigilance teams, allowing them to focus their efforts on the most significant cases.
5. Improved Data Quality and Consistency
Ensuring data quality and consistency is essential for effective pharmacovigilance. AI and ML can help maintain high data standards by identifying and correcting errors, inconsistencies, and duplicates in drug safety databases. Machine learning models can be trained to recognize patterns of common errors and apply corrections automatically, reducing the need for manual data cleaning.
Additionally, AI can standardize data from various sources, ensuring that all information is compatible and comparable. This is particularly important when integrating data from different systems or jurisdictions, where variations in terminology and reporting standards can pose significant challenges.
6. Facilitating Regulatory Compliance
Pharmaceutical companies must comply with stringent regulatory requirements for drug safety reporting. AI and ML can streamline the compliance process by automating the generation of regulatory reports, ensuring that all necessary information is included and formatted correctly. This automation reduces the risk of errors and omissions, which can lead to regulatory penalties and delays in bringing products to market.
Furthermore, AI-driven systems can monitor changes in regulatory requirements and update reporting processes accordingly, ensuring ongoing compliance with the latest standards. This proactive approach to regulatory compliance helps pharmaceutical companies avoid costly delays and legal issues.
7. Enhanced Risk Management
AI and ML contribute to more effective risk management by providing a comprehensive view of drug safety data. Advanced analytics can integrate data from multiple sources, including clinical trials, post-marketing surveillance, and real-world evidence, to create a holistic view of a drug’s safety profile. This integrated approach enables better identification of risk factors and more informed decision-making.
For example, AI-driven risk management systems can aggregate and analyze data on drug interactions, patient demographics, and treatment outcomes to identify potential risks and develop strategies to mitigate them. This proactive approach to risk management helps ensure patient safety and supports the long-term success of pharmaceutical products.
8. Continuous Monitoring and Real-Time Insights
Traditional pharmacovigilance processes often involve periodic reviews of safety data, which can result in delays in identifying and addressing safety issues. AI and ML enable continuous monitoring of drug safety databases, providing real-time insights into emerging safety signals. Machine learning models can continuously analyze incoming data, detect anomalies, and alert pharmacovigilance teams to potential issues as they arise.
This real-time monitoring capability ensures that safety signals are identified and addressed promptly, reducing the risk of adverse events and improving patient outcomes. It also allows pharmaceutical companies to respond quickly to new information, adapting their safety strategies as needed.
While the benefits of AI and ML in drug safety case processing are substantial, there are also challenges and considerations to address. Implementing AI and ML systems requires significant investment in technology and expertise, as well as robust data governance and security measures. Ensuring the quality and completeness of data is critical, as the effectiveness of AI and ML models depends on the accuracy of the input data.
Moreover, there are ethical considerations related to the use of AI in healthcare, including issues of transparency, accountability, and bias. It is essential to develop AI and ML systems that are transparent in their decision-making processes, accountable for their actions, and designed to minimize bias.
Conclusion:
AI and ML are transforming the landscape of pharmacovigilance, offering powerful tools to simplify case processing in drug safety databases. By automating data entry and preprocessing, enhancing signal detection, providing predictive analytics, and improving risk management, these technologies are revolutionizing how pharmaceutical companies monitor and ensure the safety of their products.
As AI and ML continue to advance, their role in drug safety will only become more significant, offering new opportunities to enhance patient safety and streamline regulatory compliance. Embracing these technologies is essential for the pharmaceutical industry to keep pace with the growing complexity of drug safety data and to ensure the ongoing protection of public health.
Comentários