How AI and ML Transform Signal Detection in Drug Safety
- Chaitali Gaikwad
- Oct 28, 2024
- 5 min read
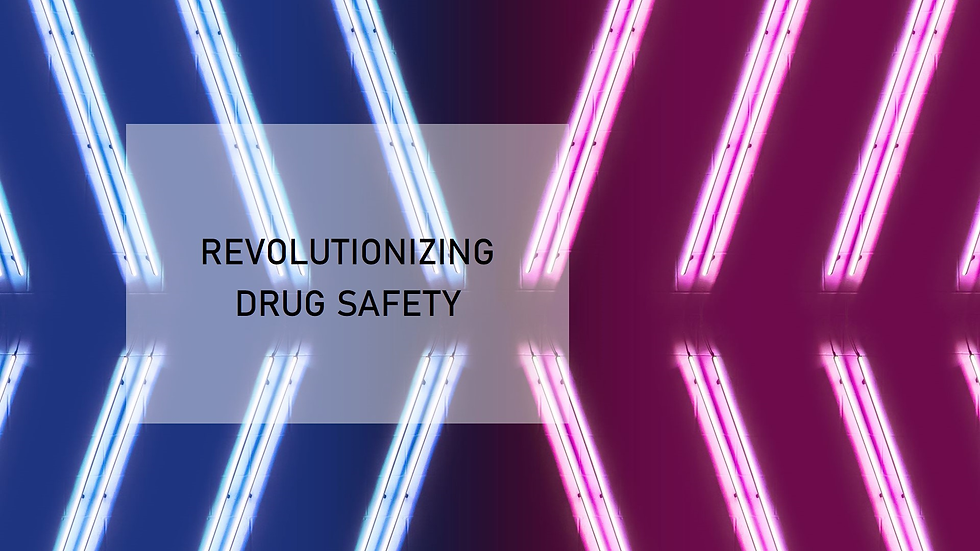
In the field of drug safety, signal detection plays a critical role in identifying potential risks associated with medicinal products. Traditional methods for signal detection often involve manual processes, statistical reviews, and individual case safety reports (ICSRs). However, the increasing volume of data—ranging from clinical trials to real-world evidence—has made it challenging for pharmacovigilance teams to identify adverse drug reactions (ADRs) efficiently and accurately. Artificial Intelligence (AI) and Machine Learning (ML) are revolutionizing signal detection by enhancing accuracy, scalability, and speed, providing more timely insights to protect public health. This blog explores how AI and ML are transforming signal detection in drug safety, their benefits, and practical strategies for implementation.
1. Understanding Signal Detection in Drug Safety
Signal detection refers to the process of identifying new, unexpected, or changing risks associated with pharmaceutical products. A signal is defined as information that suggests a causal association between a drug and an adverse event that was previously unknown or not fully established. Pharmacovigilance professionals analyze spontaneous reports, scientific literature, electronic health records (EHRs), and social media to detect potential safety signals.
However, challenges arise when vast amounts of data need to be evaluated. Traditional statistical tools such as disproportionality analysis (e.g., the reporting odds ratio) and Bayesian inference require significant manual effort and are limited in scope. This is where AI and ML come into play, automating the detection process and improving both efficiency and precision.
2. How AI and ML Enhance Signal Detection
2.1 Automated Data Mining
AI and ML algorithms excel at processing large datasets to identify meaningful patterns. Using automated data mining techniques, ML systems can comb through spontaneous adverse event reports, scientific journals, and clinical records to detect signals faster than manual methods. Tools like Natural Language Processing (NLP) enable machines to read and extract relevant insights from unstructured text, such as patient narratives and published case reports.
Example:
NLP-powered models can automatically scan adverse event databases to detect frequently reported ADRs, reducing the need for labor-intensive manual reviews.
2.2 Enhanced Pattern Recognition
ML models, such as neural networks and random forests, can identify non-obvious correlations between drugs and adverse events by analyzing complex datasets. Traditional tools may struggle to detect weak signals, especially when dealing with rare adverse events. However, ML models improve signal sensitivity by recognizing intricate patterns, even from low-frequency events.
Example:
A random forest model trained on historical ADR data can highlight subtle correlations between drug interactions and adverse outcomes, providing early warning signals to pharmacovigilance teams.
2.3 Faster Identification of Emerging Risks
AI systems can monitor real-time sources of data, such as EHRs, wearables, and social media, to detect early indicators of drug-related risks. This capability ensures that potential threats are identified as soon as they emerge, enabling pharmaceutical companies and regulators to act quickly. By leveraging predictive analytics, AI systems forecast possible ADR trends before they fully materialize, helping to mitigate risks proactively.
Example:
AI-powered surveillance systems scan social media posts for mentions of side effects linked to new drugs, allowing faster identification of potential safety concerns.
2.4 Multi-Source Signal Detection
Traditional pharmacovigilance methods often rely heavily on spontaneous reporting systems. However, AI and ML tools can integrate data from multiple sources, including clinical trials, EHRs, claims databases, and post-market surveillance reports, for more comprehensive signal detection. This multi-source approach minimizes the risk of missing critical signals and improves the quality of safety assessments.
Example:
ML models can combine data from multiple databases—such as patient health records and insurance claims—to detect overlapping patterns, improving the reliability of signal detection.
2.5 Real-Time Signal Validation
AI-powered systems can prioritize and validate signals based on predefined criteria, such as signal strength, frequency, and clinical relevance. Once a signal is identified, ML algorithms perform statistical validation to determine whether the observed association is genuine or due to chance. This process reduces false positives, ensuring that only meaningful signals are escalated for further investigation.
Example:
An ML-based alert system automatically flags and validates safety signals in real-time, allowing pharmacovigilance teams to focus on the most critical risks.
3. Benefits of AI and ML in Signal Detection
3.1 Improved Accuracy
AI algorithms can analyze vast datasets with high precision, reducing the chances of human error. This ensures that even subtle safety signals are detected, improving the overall reliability of pharmacovigilance processes.
3.2 Increased Efficiency
By automating data collection, analysis, and validation, AI and ML tools reduce the time required to identify safety concerns. This allows organizations to respond to risks more rapidly, minimizing potential harm to patients.
3.3 Scalability
As the volume of safety data grows, AI systems can scale to accommodate new information sources and datasets. This ensures that pharmacovigilance teams remain agile in handling both current and emerging challenges.
3.4 Better Resource Allocation
AI-driven systems streamline the signal detection process, allowing pharmacovigilance professionals to focus their efforts on high-priority cases. This improves the allocation of resources and enhances decision-making.
3.5 Early Risk Mitigation
Predictive models enable organizations to act proactively, mitigating risks before they escalate into widespread safety issues. This approach not only improves patient safety but also strengthens public trust in the pharmaceutical industry.
4. Challenges in Implementing AI and ML for Signal Detection
4.1 Data Quality and Integrity
AI and ML models rely on high-quality data to produce accurate results. Inconsistent or incomplete data can lead to incorrect conclusions, compromising the safety signal detection process.
4.2 Regulatory Compliance
Pharmaceutical companies must ensure that AI-based signal detection tools comply with stringent regulatory guidelines. The European Medicines Agency (EMA) and the U.S. Food and Drug Administration (FDA) emphasize transparency and accountability in using AI for pharmacovigilance.
4.3 Interpretability of ML Models
ML models, especially deep learning algorithms, can sometimes function as "black boxes," making it difficult for users to understand how specific conclusions were reached. Ensuring the interpretability of models is essential for regulatory acceptance and trust among pharmacovigilance professionals.
4.4 Integration with Existing Systems
Seamlessly integrating AI-powered signal detection tools with legacy pharmacovigilance systems can be challenging. Organizations must invest in data integration frameworks to ensure smooth interoperability.
4.5 Ethical Considerations
AI tools must be designed with fairness and transparency in mind to avoid bias. For example, models trained on biased datasets might disproportionately flag certain demographic groups, leading to inequitable safety outcomes.
5. Best Practices for Implementing AI and ML in Signal Detection
Collaborate with Regulators: Work closely with regulatory agencies to ensure AI tools meet compliance requirements.
Focus on Data Quality: Implement robust data validation protocols to ensure input data is accurate and complete.
Develop Explainable Models: Use interpretable AI models, such as decision trees or linear models, to enhance transparency.
Invest in Training: Train pharmacovigilance teams to understand how AI and ML tools work, enabling them to make informed decisions.
Adopt a Multi-Source Approach: Leverage data from multiple sources, such as EHRs, social media, and clinical trials, for more comprehensive signal detection.
6. Future Trends in AI-Driven Signal Detection
6.1 Integration with Wearable Devices
Wearable technology offers real-time health data that can be used to monitor patients continuously. AI systems will increasingly leverage data from wearables to detect potential ADRs and provide personalized safety monitoring.
6.2 Advanced NLP Models
With the rapid advancement of NLP, future AI systems will be capable of analyzing vast amounts of unstructured data, such as patient forums and scientific articles, with even greater accuracy.
6.3 Collaborative AI Models
Pharmaceutical companies may adopt federated learning, where AI models learn from decentralized datasets without sharing sensitive information. This approach ensures privacy while improving the robustness of signal detection.
Conclusion
AI and ML are transforming signal detection in drug safety by enhancing the speed, accuracy, and scalability of pharmacovigilance processes. These technologies enable real-time monitoring, predictive analytics, and multi-source data integration, allowing organizations to detect and mitigate risks proactively. While challenges such as data quality, regulatory compliance, and model interpretability must be addressed, the benefits far outweigh the risks. As the pharmaceutical industry continues to embrace AI and ML, the future of drug safety will become more efficient, transparent, and patient-centric.
Very informative. for more information visit us on medical coding training