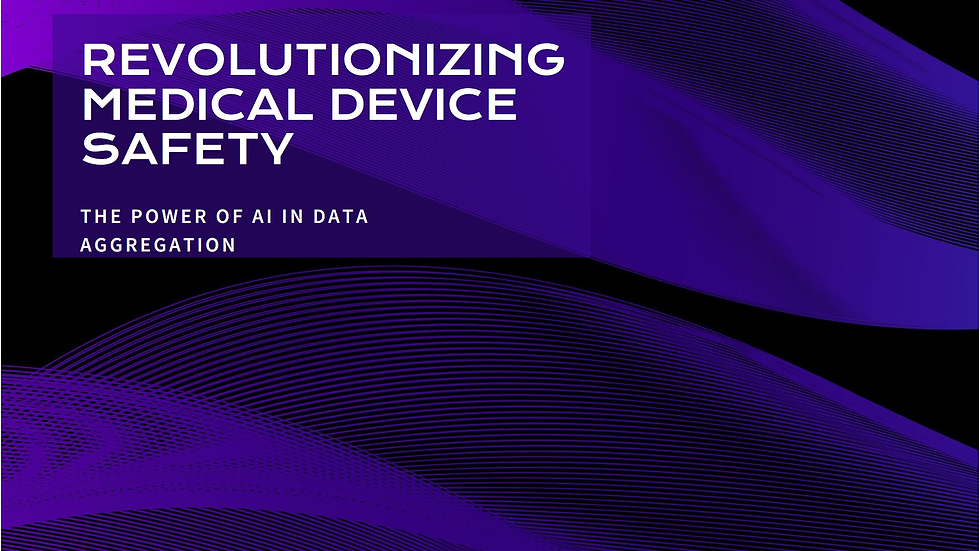
In the fast-evolving landscape of healthcare technology, the safety of medical devices has become a paramount concern. As medical devices become increasingly complex and integrated into patient care, the need for robust data aggregation methods has never been more critical. Artificial Intelligence (AI) is at the forefront of revolutionizing how data is collected, analyzed, and utilized in ensuring medical device safety. This blog explores the role of AI in enhancing data aggregation in medical device safety, detailing its applications, benefits, challenges, and future prospects.
Understanding Data Aggregation in Medical Device Safety
Data aggregation refers to the process of collecting, organizing, and summarizing data from various sources to provide meaningful insights. In the context of medical device safety, this involves gathering data from clinical trials, post-market surveillance, patient feedback, electronic health records (EHRs), and adverse event reports. The aggregated data is crucial for identifying potential safety issues, understanding device performance, and ensuring compliance with regulatory requirements.
The traditional methods of data aggregation in medical device safety are often time-consuming and prone to errors. Manual data entry, disparate data sources, and lack of standardization can lead to incomplete or inaccurate datasets. As a result, there is an urgent need for innovative solutions to streamline data aggregation processes, enhance data quality, and ultimately improve patient safety.
The Role of AI in Data Aggregation
AI technologies, particularly machine learning (ML) and natural language processing (NLP), play a significant role in enhancing data aggregation in medical device safety. Here are some key areas where AI is making an impact:
1. Automating Data Collection
One of the most significant advantages of AI is its ability to automate data collection from various sources. Traditional methods often require extensive manual effort, resulting in delays and potential human errors. AI algorithms can automatically extract relevant data from clinical trial reports, EHRs, and social media platforms, significantly reducing the time and resources needed for data collection.
For instance, AI-powered web scraping tools can scour online platforms for real-time information on medical devices, including user reviews and adverse event reports. This allows safety teams to gather a more comprehensive dataset, enabling them to identify potential safety signals quickly.
2. Enhancing Data Standardization
Inconsistencies in data formats and terminologies pose significant challenges in data aggregation. AI can help standardize data by converting various data types into a unified format, making it easier to analyze and interpret. For example, NLP algorithms can understand and standardize clinical terminology, ensuring that data from different sources is consistent and comparable.
By employing AI-driven data normalization techniques, organizations can enhance the quality of their aggregated data, leading to more accurate insights and improved decision-making.
3. Advanced Data Analysis
Once data is collected and standardized, the next step is analysis. AI algorithms can analyze vast amounts of data at unprecedented speeds, uncovering patterns and correlations that may not be immediately apparent through traditional methods. For example, machine learning models can identify trends in adverse events related to specific devices, helping manufacturers and regulators assess safety risks more effectively.
Furthermore, AI can support predictive analytics by forecasting potential safety issues based on historical data. By identifying patterns in adverse events, organizations can proactively address safety concerns and implement corrective actions before issues escalate.
4. Improving Data Visualization
Effective data visualization is crucial for communicating safety insights to stakeholders, including healthcare providers, regulatory authorities, and patients. AI-enhanced data aggregation tools can generate dynamic visualizations that illustrate trends, correlations, and anomalies in the data.
For instance, interactive dashboards powered by AI can present aggregated safety data in real-time, allowing users to explore the data and derive insights easily. This enhances decision-making and facilitates timely interventions to mitigate safety risks.
5. Facilitating Regulatory Compliance
Regulatory compliance is a significant concern for medical device manufacturers. AI can streamline compliance processes by automating the collection and reporting of required data. By aggregating safety data from various sources, AI can generate comprehensive reports that fulfill regulatory requirements, reducing the administrative burden on organizations.
Moreover, AI can help organizations stay informed about changing regulations and guidelines, ensuring that they remain compliant in an evolving landscape. This proactive approach to compliance not only enhances safety but also fosters trust among stakeholders.
Benefits of AI-Enhanced Data Aggregation
The integration of AI in data aggregation for medical device safety offers several benefits:
1. Increased Efficiency
AI-powered data aggregation tools significantly reduce the time and resources required for data collection and analysis. This allows organizations to focus on strategic decision-making rather than manual data handling, leading to improved overall efficiency.
2. Enhanced Data Quality
AI enhances the quality of aggregated data through automation, standardization, and advanced analysis. Higher quality data translates to more accurate insights, enabling organizations to make informed decisions regarding medical device safety.
3. Proactive Risk Management
With predictive analytics capabilities, AI can identify potential safety issues before they become critical. This proactive approach to risk management helps organizations implement timely interventions, enhancing patient safety and device performance.
4. Better Stakeholder Communication
AI-driven data visualization tools improve communication with stakeholders by presenting complex data in an understandable format. This fosters transparency and facilitates collaboration among manufacturers, regulators, and healthcare providers.
5. Cost Savings
By automating data aggregation processes and improving data quality, organizations can achieve significant cost savings. Reducing the time and resources spent on manual data handling allows for more efficient allocation of funds toward safety initiatives and research.
Challenges and Considerations
While the benefits of AI-enhanced data aggregation are significant, several challenges must be addressed:
1. Data Privacy and Security
The collection and aggregation of sensitive patient data raise concerns about privacy and security. Organizations must ensure compliance with regulations such as the Health Insurance Portability and Accountability Act (HIPAA) and implement robust data protection measures.
2. Data Integration
Aggregating data from various sources can be challenging due to differences in data formats, terminologies, and structures. Organizations must invest in AI solutions that can effectively integrate and standardize diverse datasets.
3. Algorithm Bias
AI algorithms can inadvertently introduce bias if they are trained on unrepresentative datasets. It is crucial for organizations to monitor and evaluate their AI systems regularly to mitigate the risk of biased outcomes.
4. Regulatory Acceptance
The use of AI in data aggregation for medical device safety is still a relatively new field. Regulatory bodies are in the process of developing guidelines and standards for AI applications in healthcare. Organizations must stay informed about these developments to ensure compliance and acceptance of their AI-driven solutions.
Future Prospects
The future of AI-enhanced data aggregation in medical device safety is promising. As technology continues to advance, we can expect the following trends:
1. Integration of Real-Time Data
The ability to aggregate real-time data from various sources will become increasingly important. AI will facilitate the integration of data from wearable devices, remote monitoring systems, and patient-reported outcomes, providing a more comprehensive view of device safety.
2. Enhanced Collaboration
AI will enable greater collaboration among stakeholders, including manufacturers, healthcare providers, and regulatory bodies. By sharing aggregated data and insights, organizations can work together to address safety concerns and improve device performance.
3. Improved Personalization
As AI continues to evolve, personalized approaches to medical device safety will become more feasible. AI algorithms can analyze individual patient data to identify specific risks associated with particular devices, allowing for tailored safety measures.
4. Increased Focus on Patient-Centric Approaches
The integration of patient feedback into data aggregation processes will become increasingly important. AI can help organizations gather and analyze patient-reported outcomes, enhancing the understanding of device safety from the patient's perspective.
5. Continuous Learning and Improvement
AI systems will continue to learn from new data, allowing for ongoing improvements in data aggregation methods. As organizations adapt to changing regulatory requirements and technological advancements, AI will play a crucial role in ensuring that data aggregation processes remain effective and relevant.
Conclusion:
AI is transforming the landscape of data aggregation in medical device safety, offering innovative solutions to enhance efficiency, data quality, and patient safety. By automating data collection, standardizing information, and providing advanced analytical capabilities, AI empowers organizations to make informed decisions and proactively address safety concerns. While challenges remain, the future of AI in medical device safety is promising, with the potential to improve collaboration, personalization, and continuous learning in data aggregation processes. As the healthcare industry continues to embrace AI, the benefits for medical device safety and patient outcomes will undoubtedly be significant.
Comments