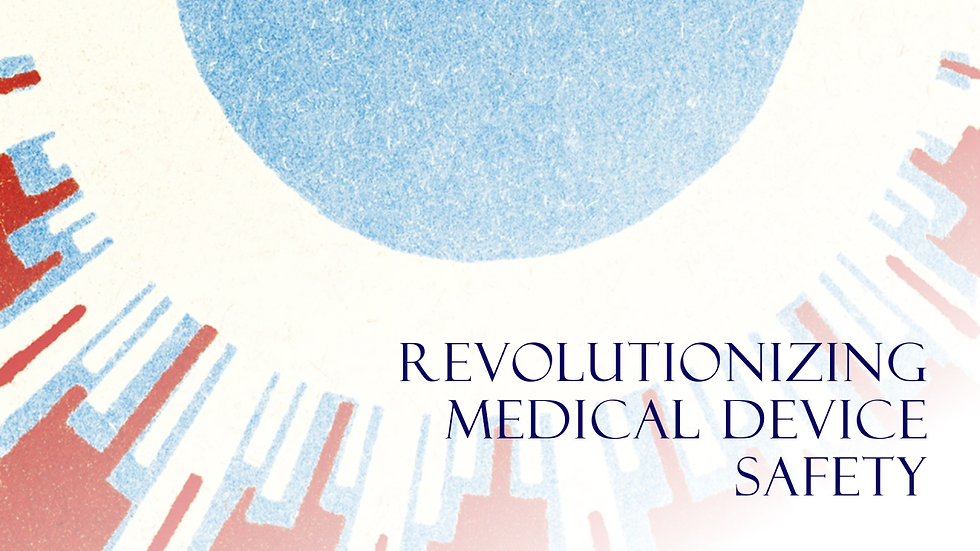
Artificial intelligence (AI) is revolutionizing various sectors, and healthcare is no exception. In the realm of medical device safety, AI offers unprecedented capabilities to analyze vast amounts of data, identify patterns, and predict adverse events. This blog explores how AI enhances data analysis for medical device safety, empowering healthcare professionals and regulatory authorities to improve patient outcomes and ensure the safety and efficacy of medical devices.
1. Automating Data Processing
Medical device safety involves monitoring and analyzing a wide range of data sources, including adverse event reports, clinical trials, and post-market surveillance data. AI algorithms can automate data processing tasks, such as data entry, normalization, and cleansing, significantly reducing the time and resources required for manual data management. By automating routine tasks, AI enables healthcare professionals to focus on more complex analyses and decision-making processes.
2. Identifying Safety Signals
One of the primary challenges in medical device safety is detecting safety signals—indicators of potential adverse events or device malfunctions—amidst large volumes of data. AI excels at pattern recognition and can identify subtle correlations and trends that may not be apparent to human analysts. Machine learning algorithms can analyze disparate data sources to identify safety signals and prioritize them for further investigation. By detecting safety signals early, AI helps healthcare professionals and regulatory authorities take proactive measures to mitigate risks and protect patient safety.
3. Predictive Analytics
AI enables predictive analytics by leveraging historical data to forecast future events, including adverse events and device failures. Machine learning models can analyze past safety data, device performance metrics, and patient characteristics to predict the likelihood of adverse events occurring. This predictive capability allows healthcare professionals to anticipate safety issues before they occur and implement preventive measures to minimize harm to patients. By leveraging predictive analytics, AI empowers healthcare organizations to optimize resource allocation and improve patient outcomes.
4. Enhancing Data Integration
Medical device safety data is often fragmented and dispersed across different systems and databases, making it challenging to analyze comprehensively. AI facilitates data integration by aggregating and harmonizing data from multiple sources, including electronic health records, adverse event databases, and medical device registries. By integrating diverse data sources, AI provides a unified view of device safety, enabling more robust analyses and insights. Enhanced data integration also facilitates collaboration among stakeholders, including healthcare providers, manufacturers, and regulatory authorities, to address safety concerns collaboratively.
5. Real-Time Monitoring
In the era of connected healthcare, medical devices generate vast amounts of real-time data that require continuous monitoring for safety issues. AI-powered systems can analyze streaming data from medical devices and wearables in real-time to detect anomalies and safety signals. By monitoring device performance and patient health indicators in real-time, AI enables proactive interventions to prevent adverse events and ensure patient safety. Real-time monitoring also enhances post-market surveillance by providing timely insights into device performance and safety.
6. Supporting Regulatory Compliance
Regulatory compliance is a critical aspect of medical device safety, requiring rigorous data analysis and reporting to regulatory authorities. AI streamlines regulatory compliance by automating data analysis, generating regulatory reports, and ensuring data accuracy and completeness. Machine learning algorithms can identify compliance issues and flag deviations from regulatory requirements, enabling timely corrective actions. By supporting regulatory compliance, AI helps manufacturers and healthcare organizations navigate complex regulatory landscapes and uphold safety standards.
Case Studies:
1. FDA's Sentinel Initiative
The FDA's Sentinel Initiative is a prime example of how AI enhances data analysis for medical device safety. The initiative leverages electronic health record data and claims data to monitor the safety of medical products, including medical devices, in near real-time. AI algorithms analyze vast amounts of healthcare data to identify safety signals and inform regulatory decision-making. The Sentinel Initiative demonstrates the power of AI in transforming post-market surveillance and improving patient safety.
2. IBM Watson for Drug Safety
IBM Watson for Drug Safety is another example of AI's impact on medical device safety. The platform utilizes natural language processing and machine learning to analyze unstructured data from adverse event reports, scientific literature, and social media to identify potential safety issues related to drugs and medical devices. By automating data analysis and generating actionable insights, IBM Watson for Drug Safety enables healthcare organizations to proactively manage safety risks and ensure compliance with regulatory requirements.
Challenges and Considerations:
While AI offers immense potential for enhancing data analysis in medical device safety, several challenges and considerations must be addressed:
Data Privacy and Security: Protecting patient privacy and ensuring data security are paramount concerns when analyzing healthcare data with AI.
Data Quality: Ensuring the quality and integrity of data used for AI analysis is essential to produce accurate and reliable insights.
Interoperability: Integrating data from disparate sources requires addressing interoperability challenges to ensure seamless data exchange and analysis.
Regulatory Compliance: Adhering to regulatory requirements and standards is crucial to ensure that AI-powered systems comply with safety regulations and guidelines.
Conclusion:
AI is transforming data analysis for medical device safety, enabling healthcare professionals and regulatory authorities to leverage data-driven insights to enhance patient safety and improve outcomes. By automating data processing, identifying safety signals, facilitating predictive analytics, enhancing data integration, supporting real-time monitoring, and ensuring regulatory compliance, AI empowers stakeholders to proactively manage safety risks and ensure the safety and efficacy of medical devices. While challenges remain, AI's potential to revolutionize medical device safety is undeniable, promising a future of safer and more effective healthcare.
Comments