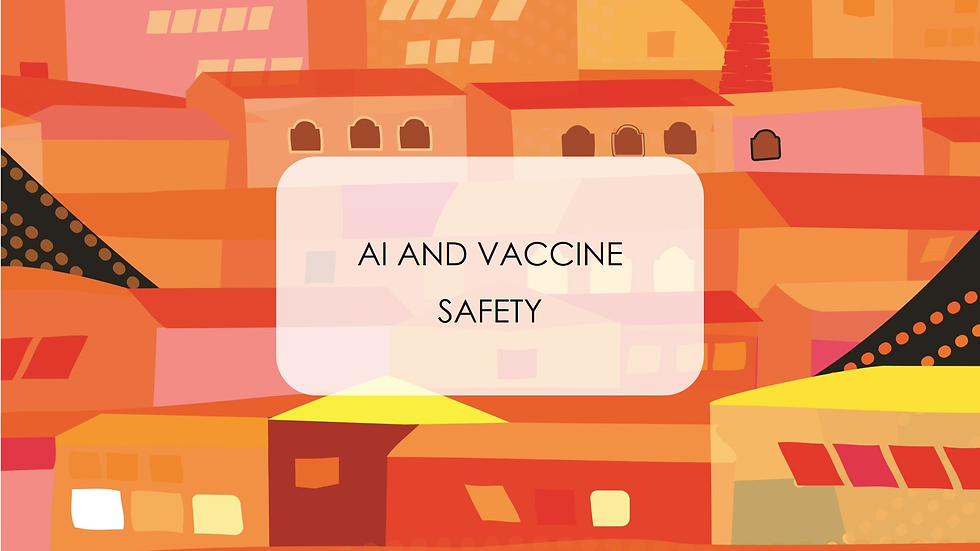
Vaccine safety is paramount in ensuring public trust and the effectiveness of immunization programs. With the unprecedented speed at which vaccines are developed, distributed, and administered, monitoring their safety has become more complex than ever. The vast amounts of data generated during vaccine rollouts, especially in global vaccination campaigns, pose a significant challenge for traditional data analysis methods. Artificial intelligence (AI) has emerged as a transformative tool, offering enhanced capabilities in data analysis, pattern recognition, and predictive modeling, ultimately improving vaccine safety monitoring.
In this blog, we will explore how AI enhances data analysis in vaccine safety monitoring, discussing its role in detecting adverse events, improving real-time surveillance, automating data processing, and ensuring regulatory compliance.
1. The Need for AI in Vaccine Safety Monitoring
Traditional vaccine safety monitoring systems, such as passive reporting systems, rely heavily on manual processes, making them prone to delays, underreporting, and inefficiencies. With the rise of global vaccine distribution, especially during the COVID-19 pandemic, these systems were stretched to their limits, necessitating more efficient and accurate methods for monitoring vaccine safety.
AI addresses these challenges by:
Automating data analysis: AI systems can process vast amounts of data from various sources quickly and efficiently, reducing the time needed for manual review.
Identifying patterns in real time: AI’s ability to detect patterns in large datasets helps identify potential safety issues faster than traditional methods.
Enhancing decision-making: AI systems can provide actionable insights and predictions, aiding public health authorities and pharmaceutical companies in making timely decisions.
By leveraging AI, vaccine safety monitoring becomes more proactive, efficient, and precise, ensuring better outcomes for public health.
2. AI in Adverse Event Detection
One of the most critical aspects of vaccine safety monitoring is the detection of adverse events following immunization (AEFI). Traditional systems, such as the Vaccine Adverse Event Reporting System (VAERS) in the U.S., are passive and rely on healthcare providers and patients to report adverse reactions. While these systems provide valuable data, they suffer from underreporting and delays, which can hinder timely safety assessments.
AI significantly enhances adverse event detection by:
a. Automating Data Mining and Reporting
AI algorithms can analyze vast amounts of unstructured data from multiple sources, including social media, healthcare records, and spontaneous reporting systems. These algorithms are trained to recognize patterns associated with vaccine-related adverse events, allowing them to automatically flag potential safety concerns.
Natural Language Processing (NLP): AI-powered NLP tools can process unstructured data, such as patient narratives, social media posts, or electronic health records (EHR), to detect keywords and phrases related to adverse events.
Machine Learning Models: Machine learning (ML) models can be trained to identify adverse event signals by analyzing patterns in the data and detecting anomalies that may indicate a vaccine safety issue.
By automating data mining and adverse event reporting, AI reduces the workload on healthcare providers and regulators, allowing them to focus on addressing potential safety concerns more efficiently.
b. Improving Early Signal Detection
AI excels at detecting weak signals that may indicate emerging safety concerns. Traditional methods often rely on a significant number of reports before a signal is detected, but AI can identify smaller clusters of adverse events that may not be immediately apparent.
Pattern Recognition: AI algorithms can detect correlations and trends in data that human analysts might miss. For example, AI can identify rare side effects by finding patterns across different populations, regions, or age groups.
Predictive Modeling: Using historical data, AI can create predictive models that forecast potential adverse events before they occur. This allows regulatory bodies and healthcare organizations to take preventive measures and communicate risks to the public proactively.
Early signal detection ensures that potential safety issues are addressed before they escalate, improving vaccine safety monitoring's overall effectiveness.
3. Real-time Monitoring and Surveillance
Real-time monitoring of vaccine safety is crucial, particularly during large-scale vaccination campaigns. Traditional safety monitoring systems often have delays in reporting, making it challenging to identify and respond to adverse events in real time. AI, however, enhances real-time surveillance by enabling continuous analysis of data from various sources.
a. Integration with Electronic Health Records (EHR)
AI can be integrated with EHR systems to monitor vaccine-related data in real time. By analyzing patient data as it is entered into the system, AI can detect any adverse events that occur after vaccination and notify healthcare providers and regulators immediately.
Automated Alerts: AI systems can be programmed to send automated alerts to healthcare providers and public health authorities when a potential adverse event is detected. This enables timely interventions and reduces the risk of widespread safety concerns.
Continuous Data Analysis: AI algorithms work 24/7, continuously analyzing incoming data from EHRs, surveillance systems, and other sources to detect emerging patterns and trends in vaccine safety.
b. Monitoring Social Media and Public Sentiment
Social media platforms have become a valuable source of real-time data for vaccine safety monitoring. AI-powered tools can analyze social media posts, forums, and online discussions to detect potential safety concerns or misinformation about vaccines.
Sentiment Analysis: AI tools can analyze the sentiment behind social media posts to gauge public opinion and identify concerns related to vaccine safety. This helps public health authorities address fears and misinformation before they spread.
Event Detection: AI can flag posts that mention specific adverse events or safety concerns, providing regulators with real-time insights into potential vaccine-related issues that may not be reported through traditional channels.
Real-time monitoring and surveillance improve the speed at which safety signals are identified and addressed, ensuring that public health responses are timely and effective.
4. Enhanced Data Processing and Integration
Vaccine safety monitoring involves analyzing data from a wide range of sources, including clinical trials, post-marketing surveillance, EHRs, and spontaneous reporting systems. Integrating and processing such diverse datasets manually is time-consuming and prone to errors. AI enhances this process by automating data integration and processing, allowing for a more comprehensive analysis of vaccine safety data.
a. Data Harmonization
One of the challenges in vaccine safety monitoring is the variety of data formats and sources, which makes it difficult to analyze data in a unified manner. AI can be used to harmonize data from different sources, ensuring that it is structured and standardized for analysis.
Automated Data Cleaning: AI algorithms can automatically clean and format data from different sources, ensuring consistency and accuracy in the analysis process.
Data Normalization: By normalizing data, AI ensures that information from different databases is comparable and can be analyzed together, providing a more comprehensive view of vaccine safety.
b. Big Data Analytics
The sheer volume of data generated during global vaccination campaigns requires powerful analytical tools. AI, particularly through big data analytics, can process and analyze large datasets far more quickly and accurately than traditional methods.
High-throughput Data Processing: AI systems can handle vast amounts of data, analyzing millions of records in a fraction of the time it would take using traditional methods. This is crucial for monitoring the safety of vaccines administered on a global scale.
AI-driven Insights: AI can extract insights from large datasets, identifying trends, correlations, and potential safety concerns that may not be immediately obvious.
By automating data processing and integration, AI ensures that vaccine safety monitoring is faster, more efficient, and less prone to human error.
5. AI and Regulatory Compliance
Regulatory bodies such as the FDA and EMA require pharmaceutical companies to monitor the safety of their vaccines and report adverse events in a timely manner. AI can help ensure regulatory compliance by automating reporting processes and improving the accuracy of safety assessments.
a. Automated Reporting
AI-powered systems can automate the reporting of adverse events to regulatory authorities, ensuring that companies meet their reporting obligations promptly and accurately.
Compliance with Reporting Timelines: AI systems can automatically generate and submit reports to regulatory bodies, ensuring compliance with reporting timelines and reducing the risk of penalties.
Accurate Data Submission: AI reduces the risk of errors in data submission by automating the process and ensuring that all relevant information is included in the reports.
b. Ensuring Transparency and Accountability
AI can also improve transparency and accountability in vaccine safety monitoring by providing auditable records of data analysis and decision-making processes. This is particularly important for regulatory reviews and inspections.
Audit Trails: AI systems can create audit trails that document the steps taken during data analysis and signal detection, providing regulators with a clear view of the process.
Automated Documentation: AI can automatically generate documentation and reports, ensuring that all necessary records are maintained for regulatory compliance.
By enhancing regulatory compliance, AI helps pharmaceutical companies maintain trust with regulators and the public, ensuring that vaccine safety is continuously monitored and assessed.
6. Challenges and Ethical Considerations
While AI offers significant benefits in vaccine safety monitoring, it also presents challenges and ethical considerations that must be addressed. These include:
Data Privacy: AI systems rely on vast amounts of data, raising concerns about patient privacy and data security. Ensuring that AI tools comply with data protection regulations, such as the GDPR, is essential.
Algorithm Bias: AI algorithms are only as good as the data they are trained on. If the data is biased or incomplete, the AI system may produce inaccurate results. Regular validation and auditing of AI algorithms are crucial to ensure fairness and accuracy.
Human Oversight: While AI can automate many aspects of data analysis, human oversight remains essential to ensure that AI-generated insights are interpreted correctly and that appropriate actions are taken.
Addressing these challenges is critical to ensuring that AI enhances vaccine safety monitoring without compromising ethical standards or public trust.
Conclusion:
AI is revolutionizing vaccine safety monitoring by enhancing data analysis, improving real-time surveillance, and ensuring regulatory compliance. By automating data processing, detecting adverse events early, and providing actionable insights
Commentaires