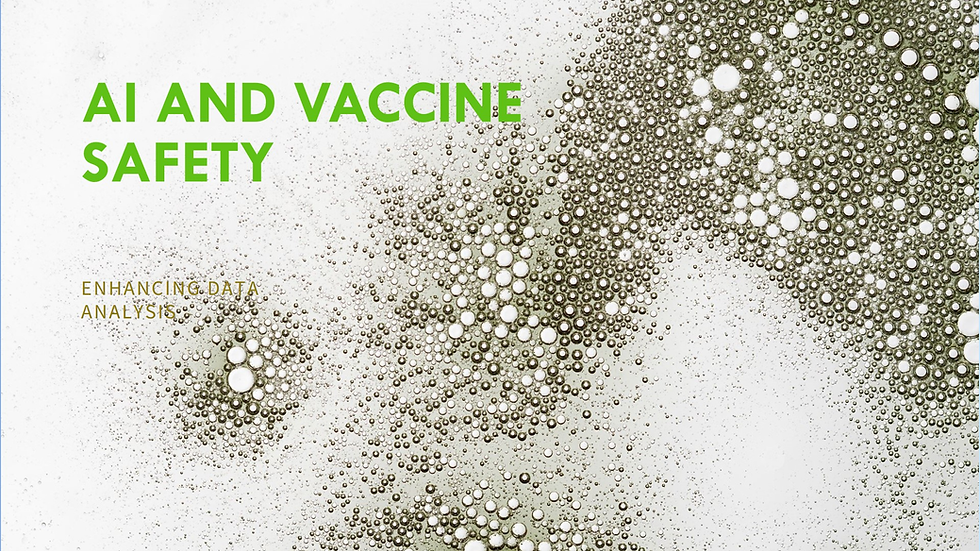
The rapid development and deployment of vaccines have been pivotal in combating infectious diseases, particularly highlighted during the COVID-19 pandemic. However, ensuring the safety and efficacy of vaccines post-licensure is paramount. Vaccine safety monitoring is an ongoing process that involves collecting, analyzing, and interpreting data related to adverse events following immunization (AEFI). Traditional data analysis methods, while effective, often struggle to manage the volume and complexity of modern data sets. This is where artificial intelligence (AI) steps in, revolutionizing how data is analyzed in vaccine safety monitoring. This blog explores how AI enhances data analysis, improving vaccine safety protocols, outcomes, and public health.
1. Understanding Vaccine Safety Monitoring
Vaccine safety monitoring involves systematic processes to detect, assess, and understand adverse events that may occur after vaccination. The primary objectives include:
Detection of Adverse Events: Identifying any potential adverse reactions or events that may arise post-vaccination.
Assessment of Causality: Determining whether there is a causal relationship between the vaccine and the reported adverse events.
Risk Management: Implementing strategies to mitigate identified risks and enhance the overall safety profile of vaccines.
Given the importance of vaccine safety, data analysis plays a critical role in informing these processes.
2. The Role of Data in Vaccine Safety
Data in vaccine safety monitoring can be categorized into various types:
Clinical Trial Data: Collected during pre-licensure clinical trials, providing initial insights into vaccine safety and efficacy.
Post-Marketing Surveillance Data: Gathered from spontaneous reports of adverse events, observational studies, and registries after the vaccine is licensed.
EHR Data: Electronic health records (EHR) provide comprehensive patient information, including medical history, vaccination status, and reported side effects.
Social Media and Public Reports: Online platforms where individuals share experiences related to vaccination, offering real-time insights into public sentiment and adverse events.
With vast amounts of data generated from these sources, traditional analysis methods can become cumbersome and inefficient. AI offers innovative solutions to streamline and enhance this process.
3. How AI Enhances Data Analysis in Vaccine Safety Monitoring
AI technologies, particularly machine learning (ML) and natural language processing (NLP), are transforming the landscape of data analysis in vaccine safety monitoring. Here’s how:
3.1. Improved Data Integration
AI enables the integration of disparate data sources, allowing for a comprehensive view of vaccine safety. For example:
Heterogeneous Data Sources: AI can consolidate data from clinical trials, EHRs, social media, and spontaneous reports into a unified framework, facilitating a holistic analysis of vaccine safety.
Real-Time Data Processing: AI systems can process real-time data streams from various sources, enabling timely detection of adverse events as they occur.
This integration enhances the accuracy of analyses and allows for quicker responses to potential safety signals.
3.2. Automated Signal Detection
Signal detection refers to identifying unusual patterns or trends in data that may indicate potential safety concerns. AI significantly enhances this process by:
Machine Learning Algorithms: Algorithms can analyze large volumes of data to identify potential signals, such as increased incidence rates of specific adverse events in vaccinated populations compared to unvaccinated groups.
Anomaly Detection: AI models can automatically detect anomalies in data, flagging cases that warrant further investigation.
Automated signal detection reduces the time and effort required for manual analyses, enabling safety monitoring teams to focus on investigating and addressing potential issues.
3.3. Advanced Causality Assessment
Predictive Modeling: Machine learning models can be trained on historical data to predict the likelihood of adverse events based on patient characteristics and vaccination status.
Causal Inference Techniques: AI can apply advanced statistical methods to assess causal relationships, helping researchers understand whether observed adverse events are likely related to vaccination.
These techniques enhance the rigor and reliability of causality assessments, supporting informed decision-making.
3.4. Natural Language Processing for Text Data
A significant portion of vaccine safety data comes from unstructured text sources, such as medical records, social media, and case reports. NLP, a branch of AI, can extract valuable insights from these text-based data sources:
Data Extraction: NLP algorithms can automatically extract relevant information from free-text reports, such as symptoms, demographic data, and timing of events.
Sentiment Analysis: AI can analyze public sentiment on social media regarding vaccine safety, providing real-time insights into public perception and potential concerns.
By transforming unstructured text into structured data, NLP enhances the comprehensiveness of analyses in vaccine safety monitoring.
3.5. Enhanced Data Visualization
AI can improve data visualization techniques, making it easier for stakeholders to understand complex analyses:
Interactive Dashboards: AI-driven dashboards can present real-time data visualizations, enabling public health officials to monitor vaccine safety metrics at a glance.
Dynamic Reporting: AI can generate dynamic reports that update automatically based on new data, ensuring that stakeholders have access to the latest information.
Enhanced visualization helps stakeholders quickly grasp trends and insights, supporting informed decision-making.
4. Case Studies and Applications of AI in Vaccine Safety Monitoring
Several organizations and research teams have successfully implemented AI technologies in vaccine safety monitoring. Here are a few notable examples:
4.1. Vaccine Adverse Event Reporting System (VAERS)
The Vaccine Adverse Event Reporting System (VAERS) in the United States has utilized AI to enhance its monitoring capabilities. By applying machine learning algorithms to historical data, VAERS can identify potential safety signals more efficiently, improving the detection of adverse events following vaccination.
4.2. The European Medicines Agency (EMA)
The EMA employs AI-driven tools to analyze large datasets from clinical trials and post-marketing surveillance. By integrating data from multiple sources and applying predictive modeling, the EMA can quickly identify potential risks associated with vaccines, ensuring timely responses to safety concerns.
4.3. Global Vaccine Safety Initiative
The World Health Organization’s Global Vaccine Safety Initiative has leveraged AI to improve its safety monitoring capabilities. By utilizing NLP techniques to analyze reports from various countries, the initiative can identify emerging safety signals and trends in vaccine-related adverse events on a global scale.
5. Challenges and Considerations in AI-Driven Vaccine Safety Monitoring
While AI offers significant advantages in vaccine safety monitoring, several challenges and considerations must be addressed:
Data Privacy and Security: The integration of sensitive health data raises concerns regarding patient privacy and data security. Organizations must implement robust measures to protect patient information.
Bias and Fairness: Machine learning models can inherit biases from training data, leading to skewed analyses. Ensuring fairness in AI algorithms is essential to avoid misinterpretations of vaccine safety.
Regulatory Compliance: AI applications in vaccine safety monitoring must comply with regulatory standards and guidelines, ensuring that analyses are conducted transparently and ethically.
Interpretability: The complexity of AI algorithms can make it challenging to interpret results. Researchers must strive for transparency in their methodologies to facilitate understanding among stakeholders.
6. The Future of AI in Vaccine Safety Monitoring
The future of AI in vaccine safety monitoring is promising, with several trends likely to shape its evolution:
Increased Automation: As AI technologies advance, the automation of data analysis processes will become more sophisticated, allowing for faster and more accurate signal detection and assessment.
Integration of Real-World Evidence: AI will play a critical role in integrating real-world evidence from diverse data sources, enhancing the understanding of vaccine safety in various populations.
Collaboration Across Sectors: Collaborative efforts between public health agencies, researchers, and technology companies will foster the development of innovative AI solutions for vaccine safety monitoring.
7. Conclusion
The integration of artificial intelligence in vaccine safety monitoring represents a transformative shift in how data is analyzed and interpreted. By leveraging AI technologies such as machine learning and natural language processing, public health officials can enhance the efficiency, accuracy, and comprehensiveness of vaccine safety monitoring processes. As we continue to navigate the complexities of vaccine safety, AI will play a vital role in safeguarding public health, ensuring that vaccines remain safe and effective for all populations.
In conclusion, the ongoing collaboration between AI technologies and vaccine safety monitoring will empower public health initiatives, foster trust in vaccination programs, and ultimately contribute to better health outcomes worldwide.
Comments