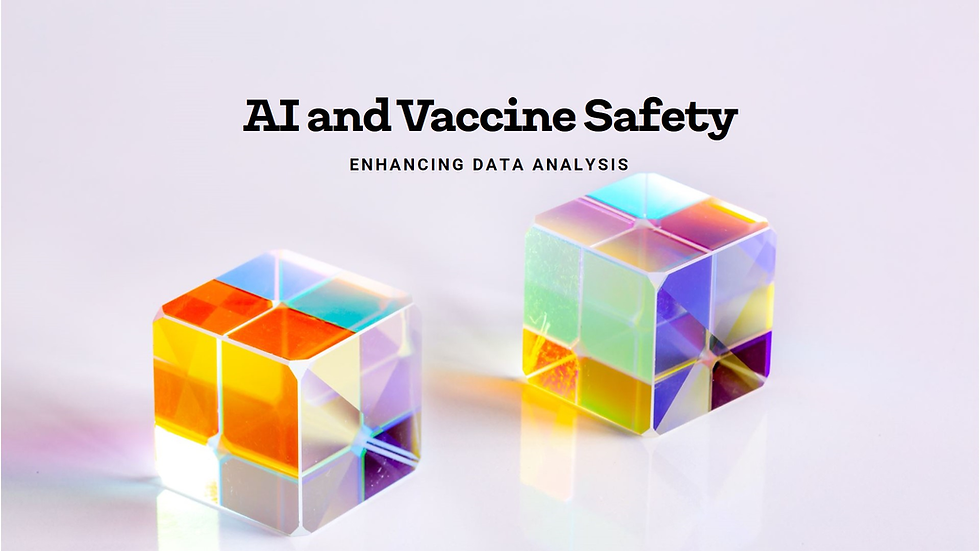
The success of vaccination programs hinges not only on the efficacy of vaccines but also on ensuring their safety through continuous monitoring. In an era where vast amounts of health data are generated daily, traditional methods of vaccine safety monitoring face challenges in managing, analyzing, and responding to this information effectively. Artificial Intelligence (AI) has emerged as a powerful tool to address these challenges, offering transformative potential in vaccine safety systems. By enabling more efficient data analysis, AI enhances the detection of adverse events, strengthens public health decision-making, and fosters a proactive approach to vaccine safety.
In this blog, we explore how AI is revolutionizing data analysis in vaccine safety systems, focusing on the technological advances that make it possible, the practical applications of AI in this field, and the impact on global public health.
1. The Need for Advanced Data Analysis in Vaccine Safety
Vaccine safety systems are tasked with monitoring the post-marketing safety of vaccines, identifying rare adverse events, and ensuring that vaccines remain safe for the public. These systems rely on vast amounts of data from various sources, including clinical trials, real-world evidence, electronic health records (EHRs), and spontaneous reporting systems like the Vaccine Adverse Event Reporting System (VAERS). The complexity and volume of data can overwhelm traditional statistical methods and manual processes.
AI, particularly in the form of machine learning (ML) and natural language processing (NLP), addresses this challenge by offering advanced techniques for analyzing data at scale. These technologies not only accelerate the analysis process but also uncover patterns and trends that may be difficult or impossible to detect through traditional methods.
2. The Role of AI in Vaccine Safety Data Analysis
AI enhances vaccine safety data analysis through several key functionalities, including automated data processing, pattern recognition, and predictive modeling. Below are the main ways AI contributes to improving vaccine safety systems:
a. Automated Adverse Event Detection
One of the most critical tasks in vaccine safety monitoring is the detection of adverse events following immunization (AEFIs). AI, through machine learning algorithms, can automatically scan large datasets for patterns that may indicate a potential safety signal. This automated detection process reduces the need for manual data entry and review, speeding up the identification of possible adverse reactions.
For example, AI models can be trained to detect rare side effects by recognizing anomalies in patient data that might be indicative of an adverse event. These models can also rank adverse events by severity, allowing public health authorities to prioritize their responses.
b. Real-Time Monitoring and Surveillance
AI-powered systems enable real-time surveillance of vaccine safety, allowing for continuous monitoring of safety data as it is reported. By integrating data from multiple sources, including EHRs, patient-reported outcomes, and global vaccine databases, AI can provide a more comprehensive view of vaccine safety.
This real-time capability is especially valuable during mass vaccination campaigns, such as those seen during the COVID-19 pandemic. AI systems can analyze the influx of data in real time, identifying any emerging safety concerns quickly and accurately. This allows public health officials to take timely actions, such as issuing safety alerts or adjusting vaccination guidelines.
c. Natural Language Processing for Unstructured Data
A significant portion of vaccine safety data is unstructured, such as written reports from healthcare providers, patient comments, or case studies. Natural language processing (NLP) algorithms can process this unstructured data, extracting relevant information and converting it into structured formats for further analysis. By doing so, NLP enhances the ability of AI systems to analyze large volumes of textual data that would otherwise go unexamined in traditional approaches.
For instance, NLP can be used to analyze social media posts, blogs, and forums where people discuss their experiences with vaccines. This unstructured data, when combined with structured datasets, provides a richer source of information for AI-powered vaccine safety analysis.
d. Predictive Modeling for Risk Assessment
AI enables predictive modeling, a powerful tool for assessing the risk of adverse events before they occur. By analyzing historical data on vaccine safety, machine learning algorithms can predict the likelihood of specific adverse events based on factors such as patient demographics, pre-existing conditions, and vaccine type. This predictive capability allows healthcare providers and regulatory agencies to identify high-risk populations and take preventive measures.
Predictive modeling also supports the development of personalized vaccination strategies, where certain individuals may receive tailored recommendations based on their risk profile. This targeted approach can help reduce the incidence of adverse events while ensuring that individuals receive the vaccines they need.
3. AI's Impact on Vaccine Safety Decision-Making
The integration of AI into vaccine safety systems enhances decision-making at various levels, from individual healthcare providers to global public health organizations. Here’s how AI improves decision-making in vaccine safety:
a. Enhancing Regulatory Oversight
Regulatory agencies like the U.S. Food and Drug Administration (FDA) and the European Medicines Agency (EMA) are responsible for ensuring that vaccines are safe for public use. AI facilitates regulatory oversight by providing these agencies with advanced tools for analyzing vaccine safety data. With AI, regulatory bodies can quickly evaluate large datasets, identify potential safety signals, and make evidence-based decisions on vaccine approvals, warnings, or recalls.
Moreover, AI allows regulators to conduct more robust post-market surveillance, ensuring that any long-term or rare adverse events are detected even after a vaccine has been approved for widespread use. This ongoing monitoring helps maintain the safety and efficacy of vaccines over time.
b. Supporting Healthcare Providers
Healthcare providers are often the first to encounter patients who experience adverse events following vaccination. AI enhances the ability of healthcare providers to make informed decisions by providing real-time safety data and risk assessments. For example, AI-powered clinical decision support tools can alert healthcare providers to potential adverse events based on patient-specific factors, helping them make safer vaccination recommendations.
These tools can also integrate with EHR systems, enabling seamless data sharing between providers and public health authorities, which further improves the accuracy and timeliness of adverse event reporting.
c. Informing Public Health Policies
AI-generated insights play a crucial role in shaping public health policies related to vaccination. By analyzing trends in vaccine safety data, AI systems can identify patterns that may suggest changes in vaccination protocols, such as adjusting dosage intervals or prioritizing certain populations for vaccination.
For instance, during the COVID-19 vaccination rollout, AI tools were used to analyze real-time data on adverse events, helping public health agencies adjust their strategies in response to emerging safety signals. This data-driven approach ensures that public health policies remain flexible and responsive to the latest safety information.
4. AI in Global Vaccine Safety Systems
The benefits of AI in vaccine safety analysis extend beyond national borders. Vaccine safety is a global concern, and AI-powered systems facilitate international collaboration on vaccine safety monitoring. Global vaccine databases, such as the World Health Organization’s Global Vaccine Safety Initiative (GVSI), are increasingly adopting AI technologies to enhance their analytical capabilities.
a. Harmonizing Data Across Borders
AI enables the harmonization of vaccine safety data from different countries, ensuring that safety signals can be detected and addressed on a global scale. For example, machine learning models can analyze data from various national vaccine safety databases to identify cross-border trends in adverse events. This harmonized approach allows public health organizations to respond to emerging safety concerns more quickly and effectively.
b. AI-Powered Collaboration
International collaboration is essential for monitoring vaccine safety during global vaccination campaigns, such as those seen during the COVID-19 pandemic. AI-powered systems enable the rapid sharing of safety data between countries, ensuring that emerging safety concerns are identified and addressed promptly. This global exchange of data enhances the overall safety of vaccines and promotes a unified approach to public health.
5. Overcoming Challenges in AI-Driven Vaccine Safety Analysis
While AI offers significant advantages for vaccine safety systems, it is not without challenges. Addressing these challenges is essential to fully realize the potential of AI in enhancing vaccine safety:
a. Data Quality and Bias
The effectiveness of AI algorithms depends on the quality of the data they analyze. Incomplete, inaccurate, or biased data can lead to incorrect conclusions and potentially harmful decisions. Ensuring high-quality data is critical for AI systems to function correctly in vaccine safety analysis. Additionally, AI developers must be vigilant about minimizing algorithmic bias, which can arise from unrepresentative training data.
b. Regulatory and Ethical Considerations
The use of AI in healthcare, particularly in vaccine safety, raises important regulatory and ethical questions. Regulatory agencies must establish clear guidelines for the development and deployment of AI systems, ensuring that these technologies are safe, transparent, and accountable. Ethical considerations, such as patient privacy and data security, must also be addressed to build public trust in AI-driven vaccine safety systems.
c. Integration with Existing Systems
AI-powered vaccine safety systems must be seamlessly integrated with existing public health infrastructure, including vaccine registries, EHRs, and global safety databases. Interoperability standards, such as HL7 and FHIR, are essential for ensuring that AI systems can communicate effectively with other healthcare technologies.
Conclusion
AI is transforming the way vaccine safety data is analyzed, offering powerful tools for detecting adverse events, improving regulatory oversight, and supporting public health decision-making. Through advanced techniques such as machine learning, natural language processing, and predictive modeling, AI enhances the efficiency and accuracy of vaccine safety monitoring systems.
As global vaccination efforts continue to expand, particularly in response to emerging infectious diseases, AI-driven vaccine safety systems will play an increasingly important role in safeguarding public health. However, realizing the full potential of AI in vaccine safety will require addressing challenges related to data quality, regulatory oversight, and system integration. With continued investment and collaboration, AI will remain a key driver of innovation in vaccine safety systems, ensuring that vaccines remain safe, effective, and trusted by the public.
Comments