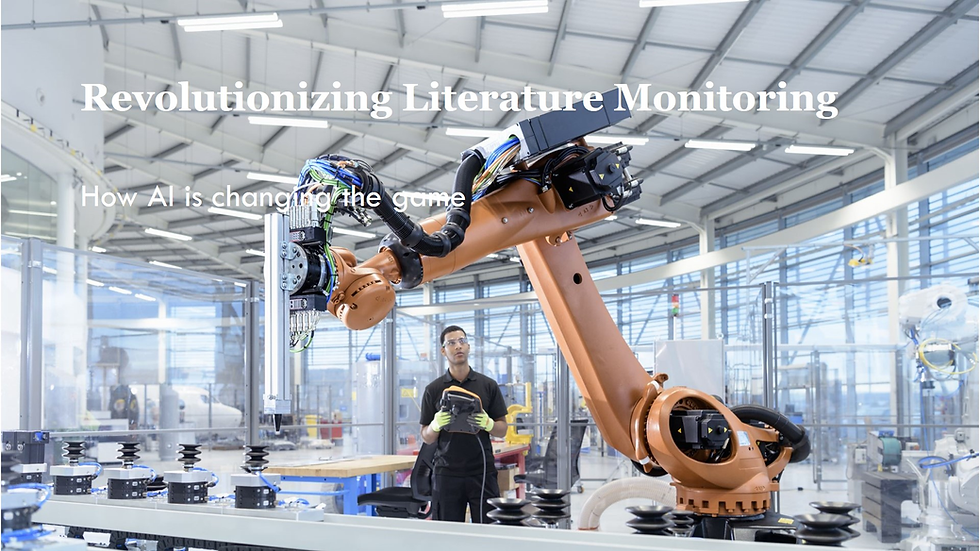
In an age where the sheer volume of information produced daily can be overwhelming, artificial intelligence (AI) has emerged as a game-changer in various fields, including literature monitoring. The integration of AI into literature monitoring processes has transformed how scholars, researchers, and institutions manage, analyze, and interpret literary works and academic publications. This blog explores how AI enhances literature monitoring, the technologies involved, and the implications for the future of literary studies.
The Challenges of Traditional Literature Monitoring
Before delving into the benefits of AI, it’s crucial to understand the challenges faced by traditional literature monitoring methods:
Volume and Diversity: The sheer amount of literature published across various disciplines and genres makes it challenging for researchers to stay current. New books, journal articles, conference papers, and online publications are continually emerging, creating a deluge of information.
Relevance and Quality: Identifying relevant and high-quality literature from the vast sea of publications is often a laborious task. Researchers must sift through numerous sources to find material pertinent to their field of study.
Data Management: Traditional literature monitoring involves manual processes for organizing and managing literature. This can be time-consuming and prone to errors, especially when dealing with large datasets.
Interdisciplinary Insights: With the increasing overlap between disciplines, discovering connections between disparate fields can be difficult. Traditional methods may not always highlight these interdisciplinary insights effectively.
AI-Driven Solutions for Literature Monitoring
AI technologies offer innovative solutions to these challenges, significantly enhancing literature monitoring processes. Here are some key ways AI is transforming this field:
1. Automated Literature Review
AI-powered tools can perform automated literature reviews by analyzing vast amounts of text data quickly and efficiently. Natural Language Processing (NLP) algorithms, a subset of AI, are particularly effective at understanding and summarizing academic content. These tools can:
Extract Key Information: AI can identify and extract key information such as research findings, methodologies, and conclusions from academic papers. This saves researchers time and helps them focus on the most relevant content.
Summarize Studies: By generating summaries of academic papers, AI tools provide concise overviews, allowing researchers to grasp the essential points without reading the entire document.
Track Trends: AI can track trends and patterns across numerous publications, providing insights into emerging research areas and shifts in academic focus.
2. Enhanced Search Capabilities
AI enhances literature search capabilities through advanced algorithms that improve search accuracy and relevance. Traditional keyword-based searches can be limited, but AI-powered search engines use:
Semantic Search: Unlike keyword searches, semantic search understands the context and meaning behind queries. This allows AI to retrieve more relevant results even if they use different terminology or phrasing.
Contextual Understanding: AI can understand the context in which certain terms are used, helping researchers find literature that is contextually relevant to their interests.
Personalized Recommendations: AI algorithms can analyze a researcher’s past work and interests to provide personalized literature recommendations, ensuring that they are kept informed about the most pertinent studies.
3. Data Mining and Analysis
AI-driven data mining tools can process and analyze large datasets from academic literature, revealing insights that might not be apparent through manual methods. These tools can:
Identify Research Gaps: By analyzing patterns and trends in existing literature, AI can help identify gaps in research, guiding future studies and encouraging new lines of inquiry.
Uncover Hidden Connections: AI can discover connections between studies that may not be immediately obvious, facilitating interdisciplinary research and collaboration.
Visualize Data: AI tools can create visualizations of data, such as graphs and networks, that illustrate relationships between different studies and concepts. These visualizations can aid in understanding complex information and presenting findings in a more accessible manner.
4. Improved Quality Control
Ensuring the quality and reliability of academic literature is crucial. AI can assist in quality control by:
Detecting Plagiarism: AI tools can compare texts to identify potential instances of plagiarism, ensuring the integrity of academic work.
Assessing Credibility: AI can evaluate the credibility of sources by analyzing factors such as author reputation, publication venue, and citation metrics.
Identifying Bias: AI can help detect biases in research by analyzing the language and content of academic papers, promoting more balanced and objective studies.
Case Studies and Applications
Several AI-powered tools and platforms have already demonstrated the transformative impact of AI on literature monitoring:
Semantic Scholar: Developed by the Allen Institute for AI, Semantic Scholar uses AI to enhance academic search capabilities. It employs NLP algorithms to understand the context of queries and provide relevant research papers. The platform also includes features like citation graph analysis and paper summarization.
Zotero: Zotero is a reference management tool that incorporates AI to help researchers organize and manage their literature. AI features include automatic citation extraction and tagging, making it easier to categorize and retrieve academic sources.
Connected Papers: This tool uses AI to create visual graphs of related research papers. By analyzing citations and paper content, Connected Papers helps researchers explore the connections between different studies and identify influential works in their field.
The Future of AI in Literature Monitoring
As AI technology continues to evolve, its role in literature monitoring is likely to expand further. Here are some potential future developments:
Enhanced Multimodal Analysis: Future AI systems may incorporate multimodal analysis, combining text with other data forms such as images, audio, and video. This could provide a more comprehensive understanding of research outputs.
AI-Driven Collaboration Platforms: AI could facilitate more effective collaboration between researchers by identifying complementary expertise and suggesting potential research partners.
Predictive Analytics: AI may develop predictive capabilities to forecast future research trends and potential breakthroughs, helping researchers stay ahead of emerging developments.
Ethical Considerations: As AI becomes more integrated into literature monitoring, ethical considerations will become increasingly important. Ensuring transparency, avoiding bias, and maintaining academic integrity will be crucial in developing and deploying AI tools.
Conclusion:
AI has revolutionized literature monitoring by addressing the challenges of volume, relevance, and data management. Through automated literature reviews, enhanced search capabilities, data mining, and quality control, AI has streamlined the research process, enabling scholars and researchers to focus on innovation and discovery. As AI technology advances, its impact on literature monitoring will likely grow, offering new opportunities for improving research efficiency and uncovering insights across disciplines. Embracing these technological advancements will be key to navigating the ever-expanding landscape of academic literature and fostering a more connected and informed research community.
Comments