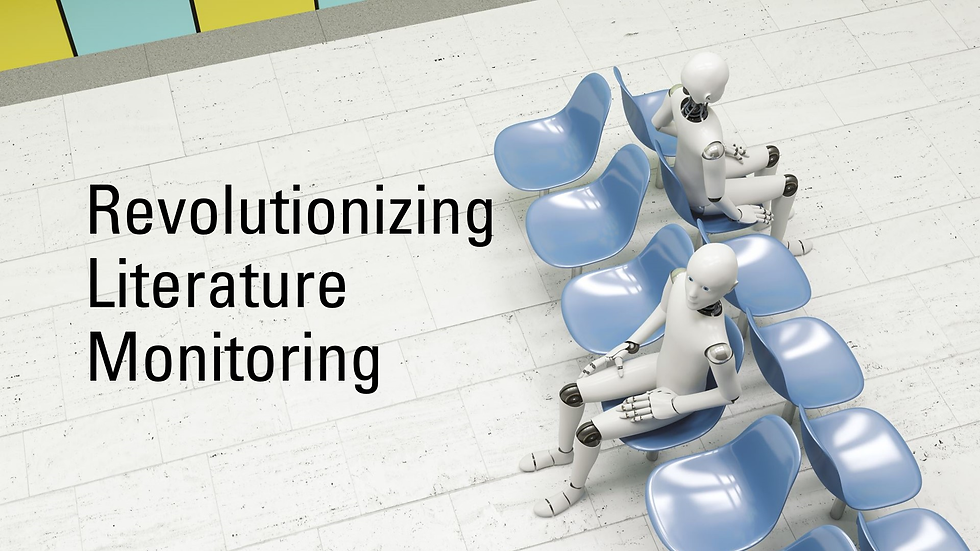
Pharmacovigilance, the practice of monitoring the effects of medical drugs after they have been licensed for use, is a crucial aspect of ensuring drug safety. A significant part of pharmacovigilance involves literature monitoring, where vast amounts of scientific and medical literature are reviewed to identify potential adverse drug reactions (ADRs) and other safety signals. Traditionally, this process has been labor-intensive, time-consuming, and prone to human error. However, the advent of artificial intelligence (AI) has revolutionized literature monitoring, making it more efficient, accurate, and responsive. In this blog, we explore how AI enhances literature monitoring processes in pharmacovigilance, focusing on the benefits, applications, and future implications of AI-driven solutions.
The Role of Literature Monitoring in Pharmacovigilance
Literature monitoring in pharmacovigilance involves systematically reviewing scientific publications, clinical studies, case reports, and other relevant sources to detect potential ADRs associated with pharmaceutical products. This process is vital for several reasons:
Early Detection of ADRs: Literature monitoring helps in the early identification of ADRs that may not have been observed during clinical trials or post-marketing surveillance.
Regulatory Compliance: Regulatory authorities, such as the European Medicines Agency (EMA) and the U.S. Food and Drug Administration (FDA), mandate continuous literature monitoring to ensure that all relevant safety information is captured and reported.
Risk Management: By identifying potential risks associated with a drug, literature monitoring supports the ongoing assessment of its benefit-risk profile, informing risk management decisions.
Patient Safety: Ultimately, literature monitoring contributes to patient safety by ensuring that any emerging risks are promptly addressed and communicated to healthcare professionals and patients.
Given the importance of literature monitoring in pharmacovigilance, it is essential to ensure that the process is as thorough and accurate as possible. This is where AI can make a significant impact.
Challenges in Traditional Literature Monitoring
Before diving into the ways AI enhances literature monitoring, it is important to understand the challenges associated with traditional approaches:
Volume of Literature: The sheer volume of literature published daily is overwhelming. Pharmaceutical companies need to review thousands of articles, making it difficult to ensure that all relevant information is captured.
Manual Data Extraction: Extracting relevant data from articles is a manual process, prone to errors and inconsistencies. This can lead to missed safety signals or incorrect data being reported.
Language Barriers: Literature is published in multiple languages, requiring pharmacovigilance teams to either rely on translations or have multilingual experts, which can be resource-intensive.
Timeliness: The time required to manually review and process literature can lead to delays in identifying and reporting ADRs, which can have serious regulatory and safety implications.
Resource Allocation: Literature monitoring is resource-intensive, requiring skilled professionals to review, interpret, and report findings. This can strain resources, especially for companies managing multiple products.
These challenges highlight the need for more efficient and reliable methods of literature monitoring, which is where AI comes into play.
How AI Enhances Literature Monitoring
AI has the potential to address the challenges of traditional literature monitoring in several ways:
1. Automation of Literature Searches
One of the most significant ways AI enhances literature monitoring is through the automation of literature searches. AI-powered tools can be programmed to conduct regular searches across multiple databases, using advanced algorithms to identify relevant articles based on predefined criteria. This automation offers several benefits:
Efficiency: Automated searches are faster and more efficient than manual searches, allowing companies to process a higher volume of literature in a shorter time frame.
Consistency: AI ensures that searches are conducted consistently, reducing the risk of human error or variability in search strategies.
Scalability: AI systems can easily scale to accommodate increases in the volume of literature or changes in search criteria, ensuring that no relevant information is missed.
By automating literature searches, AI frees up valuable time and resources, allowing pharmacovigilance teams to focus on more complex tasks, such as data interpretation and decision-making.
2. Natural Language Processing (NLP) for Data Extraction
Natural Language Processing (NLP) is a subset of AI that enables machines to understand, interpret, and generate human language. In the context of literature monitoring, NLP can be used to extract relevant data from unstructured text, such as abstracts, full-text articles, and case reports. Here’s how NLP enhances data extraction:
Accuracy: NLP algorithms can accurately identify and extract relevant information, such as drug names, ADRs, patient demographics, and outcomes, reducing the risk of errors associated with manual data extraction.
Speed: NLP processes text much faster than humans, allowing for rapid extraction of data from large volumes of literature.
Language Translation: NLP can be used to translate literature from different languages, ensuring that non-English publications are also reviewed for potential safety signals.
The use of NLP in literature monitoring not only improves the accuracy and efficiency of data extraction but also ensures that a broader range of literature is reviewed, enhancing the overall effectiveness of pharmacovigilance efforts.
3. AI-Powered Signal Detection
AI has the capability to detect patterns and trends in data that may not be immediately apparent to human reviewers. In literature monitoring, AI-powered tools can be used to identify potential safety signals by analyzing large datasets of literature and correlating them with other sources of data, such as clinical trial results and post-marketing surveillance reports. Here’s how AI enhances signal detection:
Pattern Recognition: AI algorithms can recognize patterns and trends in literature, such as the recurrence of specific ADRs or associations between certain drugs and adverse events.
Predictive Analytics: AI can use historical data to predict potential safety signals, enabling proactive risk management.
Data Integration: AI can integrate data from multiple sources, providing a comprehensive view of the safety profile of a drug and enhancing the accuracy of signal detection.
By enhancing signal detection, AI helps ensure that potential safety risks are identified and addressed promptly, reducing the likelihood of harm to patients.
4. Machine Learning (ML) for Continuous Improvement
Machine Learning (ML), a subset of AI, involves training algorithms to learn from data and improve over time. In literature monitoring, ML can be used to continuously refine and optimize the process:
Algorithm Training: ML algorithms can be trained on historical data to improve their accuracy and relevance in identifying potential safety signals.
Feedback Loops: ML systems can incorporate feedback from human reviewers to refine their performance, ensuring that they become more effective over time.
Adaptive Learning: ML allows AI systems to adapt to changes in literature and regulatory requirements, ensuring that literature monitoring remains relevant and compliant.
The use of ML in literature monitoring ensures that AI systems become more effective over time, enhancing the overall quality and reliability of the monitoring process.
5. Integration with Other Pharmacovigilance Activities
AI enhances literature monitoring by enabling seamless integration with other pharmacovigilance activities. By connecting literature monitoring with other data sources, such as electronic health records (EHRs), social media, and pharmacovigilance databases, AI can provide a more comprehensive view of a drug’s safety profile:
EHR Integration: AI can analyze data from EHRs alongside literature to identify potential safety signals that may not be captured in clinical studies.
Social Media Monitoring: AI can monitor social media platforms for mentions of ADRs, providing real-time insights into patient experiences and potential safety issues.
Cross-Database Analysis: AI can integrate data from multiple pharmacovigilance databases, such as the FDA’s Adverse Event Reporting System (FAERS) and the World Health Organization’s (WHO) Vigibase, to identify global safety trends.
By integrating literature monitoring with other pharmacovigilance activities, AI provides a more holistic approach to drug safety, enhancing the overall effectiveness of pharmacovigilance efforts.
Case Studies: AI in Action
Several examples illustrate how AI is already being used to enhance literature monitoring in pharmacovigilance:
Case Study 1: IBM Watson for Drug Safety
IBM Watson, an AI platform, has been used by pharmaceutical companies to enhance literature monitoring. Watson uses NLP and ML to analyze vast amounts of literature, identify potential safety signals, and provide insights into drug safety. By automating the literature review process, Watson has significantly reduced the time and resources required for literature monitoring, while also improving the accuracy and reliability of the data.
Case Study 2: AstraZeneca’s AI-Driven Literature Monitoring
AstraZeneca, a global pharmaceutical company, has implemented AI-driven literature monitoring as part of its pharmacovigilance strategy. The company uses AI tools to automate literature searches, extract relevant data, and detect potential safety signals. This approach has enabled AstraZeneca to process larger volumes of literature more efficiently, ensuring that all relevant safety information is captured and reported.
Case Study 3: GSK’s Use of AI in Pharmacovigilance
GlaxoSmithKline (GSK) has integrated AI into its pharmacovigilance processes, including literature monitoring. GSK uses AI-powered tools to analyze literature, detect safety signals, and assess the benefit-risk profile of its products. The use of AI has allowed GSK to enhance the efficiency and accuracy of its literature monitoring efforts, improving overall drug safety.
Future Implications of AI in Literature Monitoring
As AI technology continues to evolve, its impact on literature monitoring in pharmacovigilance is expected to grow. Here are some potential future implications:
Real-Time Literature Monitoring: AI could enable real-time literature monitoring, where safety signals are identified and reported as soon as new literature is published. This would enhance the timeliness of pharmacovigilance efforts, allowing for more rapid response to emerging safety issues.
Personalized Medicine: AI could be used to monitor literature related to specific patient populations, enabling more personalized approaches to pharmacovigilance. This would allow for the identification of safety signals that may be specific to certain demographics.
Comments