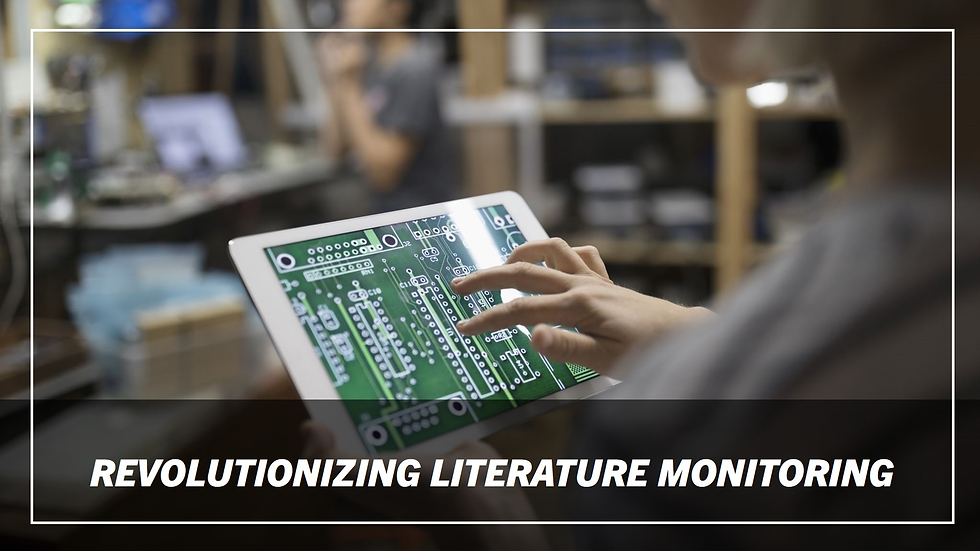
In today's information-driven world, organizations across various industries are constantly inundated with vast amounts of data and literature. From scientific research papers to regulatory updates, the sheer volume of information that needs to be monitored and analyzed can be overwhelming. Traditional literature monitoring processes, which often rely on manual review and analysis, are no longer sufficient to keep up with the pace of information generation. This is where Artificial Intelligence (AI) comes into play. AI has the potential to revolutionize literature monitoring processes by automating tasks, improving accuracy, and enabling real-time analysis. In this blog, we will explore how AI enhances literature monitoring processes, its key components, benefits, challenges, and real-world applications.
Understanding Literature Monitoring
Literature monitoring is the process of systematically tracking, reviewing, and analyzing relevant literature to stay informed about developments in a specific field. This can include scientific research, regulatory updates, legal precedents, market trends, and more. Effective literature monitoring is crucial for organizations to maintain compliance with regulations, make informed decisions, and stay ahead of competitors. However, traditional methods of literature monitoring are often time-consuming, labor-intensive, and prone to human error.
The Role of AI in Literature Monitoring
AI, with its advanced capabilities in data processing, pattern recognition, and natural language understanding, offers a transformative approach to literature monitoring. By automating many of the tasks traditionally performed by human analysts, AI enhances the efficiency, accuracy, and scalability of literature monitoring processes.
1. Automating Data Collection and Integration
One of the most significant challenges in literature monitoring is the sheer volume of data that needs to be collected and integrated from various sources. These sources can include academic journals, news outlets, regulatory databases, social media, and more. AI-powered systems can automate the process of data collection by using web scraping, APIs, and other techniques to gather information from a wide range of sources in real-time. This eliminates the need for manual data collection, saving time and reducing the risk of missing critical information.
AI also facilitates data integration by standardizing and organizing the collected data, making it easier to analyze. This is particularly important when dealing with unstructured data, such as free-text articles or social media posts. AI algorithms can automatically categorize and tag relevant content, ensuring that it is readily accessible for further analysis.
2. Enhancing Data Analysis with Natural Language Processing (NLP)
Natural Language Processing (NLP) is a branch of AI that focuses on the interaction between computers and human language. NLP plays a crucial role in literature monitoring by enabling AI systems to understand, interpret, and analyze textual data. This includes identifying key phrases, extracting relevant information, and summarizing content.
For example, in the pharmaceutical industry, AI-powered NLP tools can analyze scientific papers and extract important information related to drug safety, efficacy, and side effects. This allows pharmaceutical companies to quickly identify potential issues and take appropriate action. Similarly, in the legal field, AI can analyze court rulings and legal documents to identify relevant case law and precedents, helping legal professionals stay informed about important developments.
3. Improving Accuracy with Machine Learning (ML)
Machine Learning (ML) is another key component of AI that enhances literature monitoring processes. ML algorithms are designed to learn from data and improve their performance over time. In the context of literature monitoring, ML can be used to train AI systems to recognize patterns and trends in data, predict future developments, and identify outliers.
For instance, an ML algorithm can be trained to identify patterns in research papers that indicate emerging trends in a particular field. This allows organizations to stay ahead of the curve and anticipate future developments. ML can also be used to identify outliers or anomalies in data, such as unusual side effects reported in clinical trials, which may warrant further investigation.
4. Enabling Real-Time Monitoring and Alerts
One of the most significant advantages of AI-powered literature monitoring is the ability to conduct real-time monitoring and generate alerts. Traditional literature monitoring processes often involve periodic reviews, which can result in delays in identifying and responding to critical information. AI, on the other hand, can continuously monitor data sources and provide real-time alerts when relevant information is identified.
For example, in the financial services industry, AI-powered systems can monitor news outlets, social media, and regulatory updates to identify potential risks or opportunities. When relevant information is detected, the system can automatically generate alerts and notify the appropriate personnel, allowing them to take timely action.
Benefits of AI in Literature Monitoring
The integration of AI into literature monitoring processes offers several key benefits:
1. Increased Efficiency
AI significantly increases the efficiency of literature monitoring by automating time-consuming tasks such as data collection, integration, and analysis. This allows organizations to process larger volumes of data in less time, freeing up human resources for more strategic tasks.
2. Enhanced Accuracy
AI systems are less prone to human error, leading to more accurate and reliable results. This is particularly important in industries where accuracy is critical, such as pharmaceuticals and finance. AI can also help reduce bias in data analysis by providing objective, data-driven insights.
3. Scalability
AI-powered literature monitoring systems are highly scalable, making them ideal for organizations that need to monitor large amounts of data from multiple sources. As the volume of data increases, AI systems can easily adapt to handle the additional workload without compromising performance.
4. Cost Savings
By automating manual processes, AI reduces the need for large teams of analysts, leading to significant cost savings. Additionally, the increased efficiency and accuracy of AI systems can help organizations avoid costly mistakes and compliance breaches.
5. Customization and Flexibility
AI systems can be customized to meet the specific needs of an organization. Whether it’s monitoring particular types of literature, focusing on specific industries, or analyzing data in multiple languages, AI offers the flexibility to tailor the system to suit organizational requirements.
Challenges in Implementing AI for Literature Monitoring
While AI offers numerous benefits, implementing AI-powered literature monitoring systems is not without its challenges:
1. Data Quality and Availability
AI systems rely on large amounts of high-quality data to function effectively. If the data is incomplete, outdated, or biased, it can negatively impact the system’s performance. Ensuring the availability of high-quality data is crucial for the success of AI-powered literature monitoring.
2. Integration with Existing Systems
Integrating AI systems with existing literature monitoring processes and tools can be complex and time-consuming. Organizations need to ensure that the AI system can seamlessly connect with other tools, databases, and workflows to provide a cohesive solution.
3. Regulatory and Ethical Considerations
AI implementation must comply with various regulatory and ethical considerations, particularly when dealing with sensitive data. Organizations must ensure that their AI systems adhere to data privacy regulations, such as GDPR, and that they are used in an ethical and transparent manner.
4. Cost of Implementation
The initial cost of implementing AI-powered literature monitoring systems can be high, particularly for smaller organizations. However, these costs are often offset by the long-term benefits of increased efficiency and accuracy.
5. Need for Human Oversight
Despite the advanced capabilities of AI, human oversight is still necessary to ensure the accuracy and relevance of the results. Compliance professionals and subject matter experts need to review and validate the AI system’s findings to ensure they align with organizational goals and regulatory requirements.
Real-World Applications of AI in Literature Monitoring
1. Pharmaceutical Industry
In the pharmaceutical industry, AI-powered literature monitoring is used to track developments in drug safety, efficacy, and regulatory compliance. For example, pharmaceutical companies use AI to monitor scientific journals, clinical trial data, and adverse event reports to identify potential safety concerns and ensure compliance with regulatory requirements.
One notable example is the use of AI by Pfizer to monitor literature related to COVID-19 vaccines. AI systems were used to analyze vast amounts of scientific literature, news reports, and social media posts to identify emerging safety concerns and respond quickly to potential issues.
2. Financial Services
In the financial services industry, AI is used to monitor regulatory updates, market trends, and news reports to identify potential risks and opportunities. For instance, JPMorgan Chase has implemented AI-powered systems to monitor legal databases, news sources, and social media for relevant information, helping the company stay compliant with SEC regulations and avoid costly fines.
AI is also used to monitor financial literature for signs of market manipulation, insider trading, and other forms of financial misconduct. By analyzing large volumes of data in real-time, AI systems can detect suspicious activities and alert regulatory authorities, helping to maintain market integrity.
3. Legal Industry
Law firms are increasingly adopting AI for literature monitoring, particularly in areas such as intellectual property law, antitrust regulation, and corporate compliance. AI systems help lawyers stay informed about changes in case law, emerging legal trends, and new legislation, allowing them to provide better advice to their clients.
For example, AI-powered tools like ROSS Intelligence use NLP to analyze legal texts and provide lawyers with relevant case law and legal precedents. This not only saves time but also improves the accuracy of legal research, helping lawyers build stronger cases for their clients.
4. Healthcare
In the healthcare sector, AI is used to monitor medical literature, clinical guidelines, and regulatory updates to ensure compliance with healthcare regulations and improve patient care. For example, hospitals and healthcare providers use AI-powered systems to monitor the latest research on treatment protocols, drug interactions, and patient safety to provide evidence-based care.
AI is also used to monitor literature related to emerging infectious diseases, helping healthcare providers respond quickly to outbreaks and prevent the spread of disease. During the COVID-19 pandemic, AI systems played a crucial role in monitoring the latest research on the virus, informing public health decisions, and guiding the development of vaccines and treatments.
Conclusion
AI has the potential to transform literature monitoring processes across various industries by automating tasks, improving accuracy, and enabling real-time analysis. By leveraging AI technologies such as Natural Language Processing (NLP) and Machine Learning (ML).
Comments