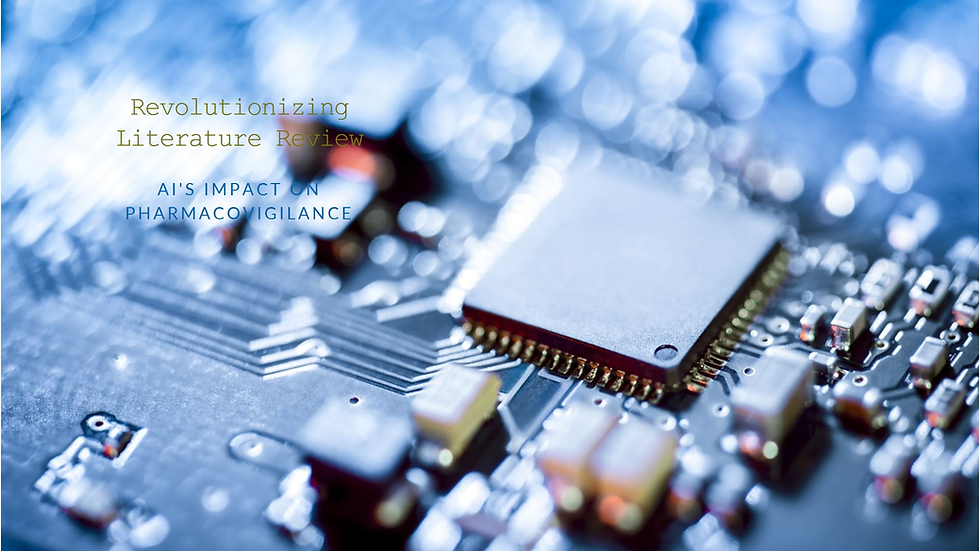
In the field of pharmacovigilance, the safety monitoring of pharmaceuticals is crucial to ensuring that drugs on the market do not pose undue risks to patients. As new research is published continuously, pharmacovigilance professionals must keep up with vast amounts of literature to identify potential safety signals, assess risks, and support regulatory compliance. Traditionally, literature reviews in pharmacovigilance have been time-consuming and labor-intensive. However, the advent of artificial intelligence (AI) is revolutionizing this process, making literature reviews more efficient, accurate, and comprehensive. This blog explores how AI enhances literature review in pharmacovigilance, detailing its benefits, applications, challenges, and future prospects.
1. The Importance of Literature Reviews in Pharmacovigilance
Literature reviews are a fundamental component of pharmacovigilance activities. They involve systematically searching, analyzing, and synthesizing research articles, clinical studies, and case reports to gather evidence regarding the safety and efficacy of medications. Key reasons for conducting literature reviews in pharmacovigilance include:
Identifying Safety Signals: By analyzing reported adverse events, literature reviews help detect potential safety signals that warrant further investigation.
Supporting Regulatory Compliance: Regulatory authorities require pharmaceutical companies to conduct literature reviews to ensure ongoing monitoring of drug safety and effectiveness.
Informing Risk Management Strategies: Comprehensive literature reviews provide the necessary data to develop risk management plans and ensure that healthcare providers and patients are informed of potential risks.
Contributing to Clinical Guidelines: Literature reviews synthesize evidence that can influence clinical guidelines and treatment protocols, ultimately improving patient care.
Given the rapid pace of research publication, it is essential to adopt innovative approaches to streamline the literature review process. This is where AI comes into play.
2. How AI Transforms Literature Reviews
AI technologies, particularly natural language processing (NLP) and machine learning (ML), have the potential to significantly enhance literature reviews in pharmacovigilance. Here are some key ways in which AI transforms this process:
A. Automated Literature Search and Retrieval
Efficient Search Algorithms: AI algorithms can perform literature searches across multiple databases, such as PubMed, Scopus, and Web of Science, using advanced search queries that go beyond simple keyword matching. These algorithms can identify relevant articles more accurately and quickly than traditional search methods.
Filtering and Prioritization: AI can filter search results based on relevance, publication date, and citation metrics, allowing pharmacovigilance professionals to prioritize high-quality studies that are more likely to provide valuable insights.
Continuous Monitoring: AI-powered systems can continuously monitor scientific literature for new publications related to specific drugs or adverse events, ensuring that pharmacovigilance teams remain updated with the latest research.
B. Data Extraction and Analysis
Automated Data Extraction: AI can automate the extraction of critical data from research articles, including adverse event types, patient demographics, and study methodologies. This reduces the time and effort required for manual data extraction and minimizes the risk of human error.
Sentiment Analysis: AI techniques, such as sentiment analysis, can assess the tone of literature related to specific drugs or adverse events, helping identify whether the sentiment is positive, negative, or neutral. This can provide insights into public perception and concerns regarding drug safety.
Cohort Identification: AI can assist in identifying relevant patient cohorts from existing studies, helping researchers understand how specific populations respond to medications and identify risk factors for adverse events.
C. Enhanced Signal Detection
Machine Learning Algorithms: ML algorithms can analyze vast datasets to detect safety signals that may not be apparent through traditional methods. By identifying patterns and correlations in adverse event data, AI can enhance the sensitivity of signal detection.
Predictive Analytics: AI can use historical data to develop predictive models that estimate the likelihood of adverse events occurring based on specific patient characteristics, treatment regimens, or drug interactions. This allows for proactive risk assessment and management.
D. Synthesis and Reporting
Automated Synthesis Tools: AI can assist in synthesizing findings from multiple studies, providing concise summaries of the evidence related to specific drugs and adverse events. This enables pharmacovigilance teams to generate reports more efficiently.
Dynamic Reporting: AI-powered reporting tools can create dynamic reports that update in real time as new data becomes available. This ensures that stakeholders always have access to the most current information regarding drug safety.
3. Benefits of AI in Literature Reviews
The integration of AI into literature reviews in pharmacovigilance offers numerous benefits:
A. Increased Efficiency
AI significantly reduces the time required for literature reviews, allowing pharmacovigilance professionals to focus on analysis and interpretation rather than manual data collection. Automated searches, data extraction, and synthesis streamline the entire process, enabling faster decision-making.
B. Improved Accuracy
AI algorithms can analyze large volumes of data with a high degree of accuracy. By minimizing human errors in data extraction and analysis, AI enhances the reliability of findings and conclusions drawn from literature reviews.
C. Enhanced Insights
AI can uncover hidden patterns and correlations that may not be readily apparent to human reviewers. This leads to a deeper understanding of drug safety and potential risks, ultimately improving patient safety and outcomes.
D. Scalability
As the volume of scientific literature continues to grow, AI offers a scalable solution for literature reviews. AI systems can handle increasing amounts of data without compromising efficiency, making them ideal for monitoring multiple drugs and therapeutic areas simultaneously.
4. Challenges of Implementing AI in Literature Reviews
While AI offers significant advantages, several challenges must be addressed when integrating AI into literature reviews in pharmacovigilance:
A. Data Quality and Standardization
The accuracy of AI algorithms relies on the quality and consistency of the data used for training. In pharmacovigilance, diverse data sources may have varying formats and terminologies, making standardization challenging. Ensuring that AI systems can effectively process and analyze heterogeneous data is essential for successful implementation.
B. Interpretation of Results
AI can generate findings and insights, but human interpretation is crucial to contextualize and validate these results. Pharmacovigilance professionals must possess the expertise to evaluate AI-generated findings and make informed decisions based on the available evidence.
C. Ethical Considerations
The use of AI in pharmacovigilance raises ethical concerns related to data privacy, informed consent, and the potential for algorithmic bias. Ensuring compliance with regulations, such as the General Data Protection Regulation (GDPR), is critical to maintaining public trust in AI technologies.
D. Resistance to Change
The integration of AI into existing workflows may face resistance from professionals accustomed to traditional literature review methods. Providing training, demonstrating the value of AI, and fostering a culture of innovation can help overcome this resistance.
5. Case Studies and Real-World Applications
Several organizations have successfully implemented AI in their literature review processes in pharmacovigilance:
A. U.S. Food and Drug Administration (FDA)
The FDA has leveraged AI and NLP techniques to enhance its surveillance capabilities. The agency developed tools that automatically analyze large volumes of literature to identify potential safety signals associated with approved drugs. By integrating AI into its pharmacovigilance framework, the FDA can respond more rapidly to emerging safety concerns.
B. Pharmaceutical Companies
Many pharmaceutical companies are investing in AI-driven solutions for literature reviews. For instance, a global pharmaceutical company implemented an AI-based literature monitoring system that automated the search, extraction, and analysis of safety data from scientific publications. As a result, the company reduced the time spent on literature reviews by over 50%, enabling quicker responses to safety signals.
C. Academic Research Institutions
Academic institutions are also exploring AI applications in pharmacovigilance. Researchers have developed AI algorithms to analyze clinical trial data and published literature, providing insights into the safety profiles of various medications. These studies demonstrate the potential of AI to enhance the rigor of pharmacovigilance research.
6. The Future of AI in Pharmacovigilance Literature Reviews
As AI technologies continue to evolve, the future of literature reviews in pharmacovigilance looks promising. Several trends are likely to shape this landscape:
A. Enhanced Integration of AI and Human Expertise
The future of pharmacovigilance literature reviews will likely involve a collaborative approach that combines AI capabilities with human expertise. AI can handle large-scale data processing, while professionals can provide context, judgment, and critical thinking to interpret findings.
B. Development of Specialized AI Tools
The growing demand for AI in pharmacovigilance is likely to drive the development of specialized tools tailored to the unique needs of this field. These tools may include advanced signal detection algorithms, predictive modeling capabilities, and dynamic reporting features.
C. Continuous Learning and Adaptation
AI systems can learn and adapt over time, improving their performance based on new data and user feedback. Continuous learning will enable AI tools to refine their algorithms and enhance their accuracy, making them even more valuable for literature reviews.
D. Greater Focus on Patient-Centric Approaches
As pharmacovigilance increasingly emphasizes patient safety and outcomes, AI can play a pivotal role in understanding patient experiences with medications. AI-powered tools can analyze patient-reported outcomes and adverse events, providing valuable insights into drug safety from the patient's perspective.
Conclusion:
AI is transforming the landscape of literature reviews in pharmacovigilance, offering significant benefits in terms of efficiency, accuracy, and insights. By automating literature searches, data extraction, and analysis, AI empowers pharmacovigilance professionals to make informed decisions and enhance patient safety.
However, challenges such as data quality, interpretation of results, ethical considerations, and resistance to change must be addressed for successful implementation. By embracing AI technologies and fostering collaboration between AI systems and human expertise, the pharmacovigilance community can harness the full potential of AI to improve drug safety monitoring and ultimately protect public health.
Kommentare