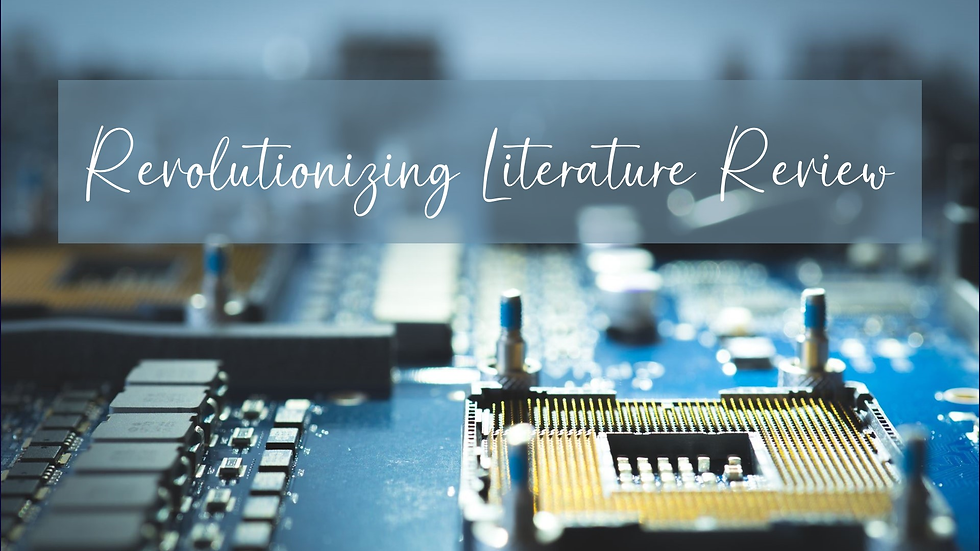
Pharmacovigilance, the science of monitoring the safety of drugs and medical devices, plays a crucial role in ensuring patient safety. One of its key components is literature review—systematically identifying, analyzing, and reporting adverse events and safety signals from scientific publications, case reports, and other relevant sources. As the volume of scientific literature grows exponentially, traditional methods of manual literature review are becoming increasingly challenging to maintain. This is where artificial intelligence (AI) steps in, transforming literature review processes and making pharmacovigilance more efficient, accurate, and timely.
In this blog, we will explore how AI enhances literature review in pharmacovigilance, highlighting its benefits, the technologies driving this transformation, challenges, and best practices for implementation.
The Role of Literature Review in Pharmacovigilance
Literature review is a key component of pharmacovigilance, responsible for identifying adverse events, safety concerns, and emerging risks associated with pharmaceutical products. Regulatory authorities such as the U.S. Food and Drug Administration (FDA) and the European Medicines Agency (EMA) mandate that pharmaceutical companies and healthcare organizations continuously monitor scientific publications and other literature for potential safety issues.
Traditionally, literature review in pharmacovigilance has been a labor-intensive and time-consuming process, requiring manual search, screening, and review of thousands of publications. This manual approach often results in delays, missed safety signals, and human errors, particularly as the number of scientific publications continues to grow. Moreover, literature is often published in different languages and formats, making it difficult for teams to stay up-to-date and comprehensive.
AI-powered solutions offer a way to automate and enhance this process, allowing pharmacovigilance teams to handle vast amounts of literature more efficiently, accurately, and in compliance with regulatory requirements.
The Benefits of AI in Literature Review for Pharmacovigilance
Implementing AI in literature review provides several key benefits that help address the limitations of manual processes.
1. Improved Efficiency and Speed
AI-driven systems can process large volumes of literature in a fraction of the time it would take a human reviewer. By automating the search, extraction, and analysis of relevant information, AI accelerates the literature review process and reduces the workload on pharmacovigilance teams. This allows companies to keep up with the increasing volume of scientific publications without compromising on the quality of the review.
Natural language processing (NLP) algorithms can rapidly analyze unstructured text from journal articles, clinical trial reports, case studies, and other literature, identifying key safety signals or adverse event reports. As a result, organizations can process more information in less time, allowing them to detect safety signals earlier and take proactive measures to ensure patient safety.
2. Enhanced Accuracy and Consistency
Manual literature review is prone to human error, especially when reviewers are required to sift through vast amounts of information. Important safety signals may be missed, and inconsistent application of screening criteria can lead to unreliable results. AI algorithms, on the other hand, provide consistent and accurate reviews by analyzing text based on predefined parameters and learning from past data.
Machine learning models can be trained to identify specific keywords, phrases, or patterns related to adverse events, drug interactions, and other safety concerns. As AI systems learn from previous reviews and outcomes, they become increasingly accurate in detecting relevant information, improving both the consistency and precision of the review process.
3. Scalability
As the volume of published literature grows, AI-powered systems can easily scale to accommodate larger datasets. Unlike manual processes that would require additional manpower to manage an increasing workload, AI systems can handle growing datasets with minimal additional resources. This scalability ensures that organizations can maintain comprehensive literature monitoring without sacrificing speed or accuracy, even as scientific publications proliferate.
4. Cost Savings
Automating literature review with AI reduces the need for large teams of manual reviewers, leading to significant cost savings. By streamlining workflows and reducing the amount of time spent on manual tasks, organizations can allocate resources more efficiently and focus on higher-value activities, such as analyzing and responding to safety signals. AI also reduces the costs associated with missed safety signals or delayed reporting by ensuring timely identification of adverse events.
5. Real-Time Monitoring
Traditional literature review processes are often reactive, with teams reviewing publications periodically, which can lead to delays in detecting safety signals. AI enables real-time monitoring of literature by continuously scanning new publications and immediately flagging relevant findings. This allows pharmacovigilance teams to respond to emerging safety concerns more quickly, ensuring that necessary actions are taken to mitigate risks and maintain regulatory compliance.
6. Regulatory Compliance
Regulatory bodies require organizations to continuously monitor the literature for adverse events and safety signals. AI systems can be programmed to ensure compliance with these requirements by automating the search and reporting processes. By systematically identifying and flagging relevant literature, AI helps organizations meet their regulatory obligations and avoid potential penalties or delays in product approvals.
AI Technologies Driving Literature Review in Pharmacovigilance
Several AI technologies are at the forefront of transforming literature review in pharmacovigilance. These technologies work together to automate and enhance the process of identifying safety signals and monitoring drug safety.
1. Natural Language Processing (NLP)
NLP is one of the most critical technologies driving AI-based literature review. It enables machines to understand, interpret, and process human language, allowing AI systems to analyze unstructured text from scientific publications. NLP algorithms can identify specific keywords, phrases, or safety signals related to adverse events, drug interactions, and patient outcomes.
For example, NLP can be used to detect mentions of adverse drug reactions in case reports or identify trends in clinical trials. By automating the extraction and analysis of this information, NLP significantly reduces the time and effort required for literature review.
2. Machine Learning (ML)
Machine learning is another essential technology used in AI-based literature review. ML algorithms learn from historical data and improve their ability to detect relevant information over time. In pharmacovigilance, machine learning models can be trained on large datasets of previously reviewed literature to identify patterns, keywords, and adverse event reports.
As ML models continue to learn from new data, they become more accurate in identifying safety signals and adverse events in future publications. This self-improving capability makes ML particularly valuable for continuously monitoring literature and ensuring that no critical information is missed.
3. Text Mining
Text mining refers to the process of extracting meaningful information from unstructured text data. In literature review, text mining algorithms can be used to search through large volumes of scientific articles and case reports, identifying relevant findings related to drug safety. By automating this extraction process, text mining reduces the burden on human reviewers and ensures that all relevant literature is considered.
4. Artificial Neural Networks (ANN)
Artificial neural networks are a subset of machine learning that mimics the way the human brain processes information. In pharmacovigilance literature review, ANNs can be used to analyze complex datasets, identify hidden patterns, and predict potential safety risks. These networks are particularly useful for processing large volumes of data and identifying subtle safety signals that may not be immediately obvious.
5. Sentiment Analysis
Sentiment analysis is a form of NLP that can be used to analyze the sentiment or tone of a piece of text. In the context of pharmacovigilance literature review, sentiment analysis can be applied to patient-reported outcomes, social media posts, or case reports to gauge public perception of a drug or identify emerging concerns related to its safety.
Challenges in Implementing AI in Literature Review
While AI offers numerous benefits for literature review in pharmacovigilance, implementing these technologies is not without challenges. Some of the key challenges include:
1. Data Quality and Availability
AI systems require high-quality data to function effectively. In some cases, scientific literature may be incomplete, poorly formatted, or published in multiple languages, making it difficult for AI systems to process. Ensuring that AI systems have access to accurate and comprehensive data is essential for their success.
2. Regulatory Compliance
AI-driven literature review must comply with stringent regulatory requirements, particularly in industries like pharmaceuticals, where patient safety is paramount. Organizations must ensure that their AI systems meet regulatory standards for data processing, reporting, and documentation.
3. Integration with Existing Systems
Integrating AI-based literature review tools with existing pharmacovigilance systems can be challenging, especially for organizations with legacy systems or fragmented data sources. Successful implementation requires seamless integration to ensure data flows efficiently between systems and that insights generated by AI are actionable.
4. Human Oversight
Despite the accuracy and efficiency of AI, human oversight is still essential for critical decision-making. Pharmacovigilance teams must work alongside AI systems, ensuring that findings are correctly interpreted and that necessary actions are taken to mitigate risks.
Best Practices for Implementing AI in Literature Review
To successfully implement AI in literature review for pharmacovigilance, organizations should follow these best practices:
1. Define Clear Objectives
Before implementing AI, organizations should define clear objectives for the initiative. This includes determining what types of literature need to be monitored, what specific safety signals or adverse events should be flagged, and what regulatory requirements must be met.
2. Select the Right AI Tools
Choosing the right AI tools and platforms is critical for success. Organizations should select AI tools that are designed for healthcare and pharmacovigilance, ensuring that they are capable of processing complex scientific literature and identifying relevant safety signals.
3. Train AI Models with High-Quality Data
AI models must be trained on high-quality, relevant data to ensure accuracy. Organizations should provide AI systems with comprehensive datasets of previously reviewed literature and adverse event reports, allowing the models to learn from past data.
4. Establish Human-AI Collaboration
While AI can automate much of the literature review process, human oversight is essential. Organizations should establish a system where AI works alongside human reviewers, ensuring that critical findings.
Comments