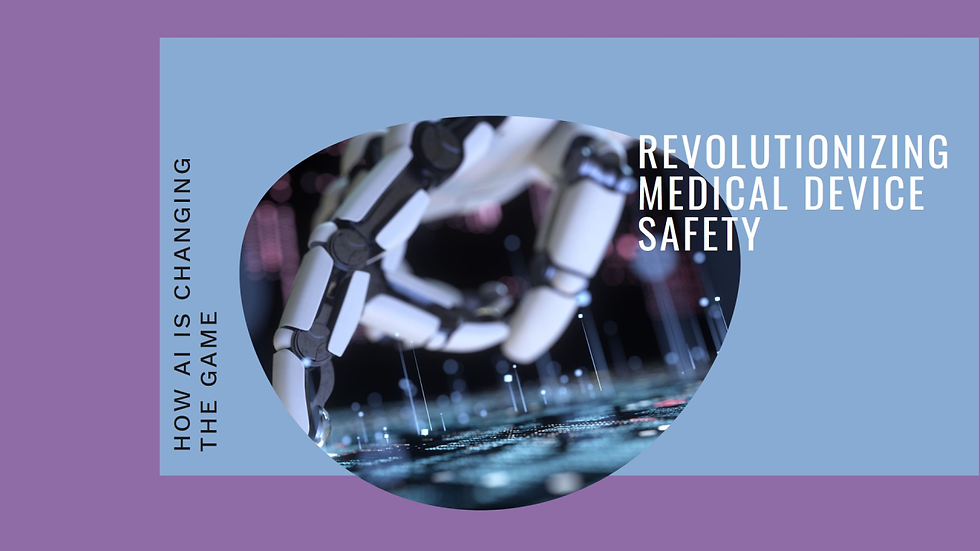
In today's rapidly evolving healthcare landscape, ensuring the safety and efficacy of medical devices is paramount. With the advent of Artificial Intelligence (AI), there has been a paradigm shift in how we approach medical device safety monitoring. AI offers unprecedented opportunities to analyze vast amounts of data, identify patterns, and detect potential safety issues with greater speed and accuracy than ever before. In this blog, we will explore how AI enhances monitoring for medical device safety and its implications for patient care and regulatory oversight.
The Role of AI in Medical Device Safety Monitoring:
AI has transformed medical device safety monitoring by enabling more efficient and proactive approaches to data analysis and signal detection. Traditional methods of monitoring relied heavily on manual review processes, which were time-consuming and often prone to human error. In contrast, AI algorithms can analyze diverse data sources, including adverse event reports, electronic health records, and real-world evidence, to identify safety signals and trends in near real-time. By leveraging machine learning techniques, AI systems can continuously learn from new data and adapt their algorithms to improve the accuracy and reliability of safety monitoring.
Enhanced Signal Detection:
One of the key benefits of AI in medical device safety monitoring is its ability to enhance signal detection capabilities. AI algorithms can sift through large volumes of data and identify subtle patterns or correlations that may indicate potential safety issues. These algorithms can analyze structured and unstructured data, including text, images, and sensor data, to uncover hidden insights and detect emerging trends. By detecting safety signals early, AI-powered systems enable regulators and healthcare providers to take proactive measures to mitigate risks and protect patient safety.
Predictive Analytics for Risk Stratification:
AI facilitates predictive analytics for risk stratification, allowing stakeholders to prioritize their resources and interventions effectively. Machine learning models can analyze historical data to predict the likelihood of adverse events or safety concerns associated with specific medical devices. By considering factors such as patient demographics, device characteristics, and clinical outcomes, these models can stratify patients based on their risk profiles and identify high-risk populations that may require closer monitoring or intervention. By targeting resources where they are most needed, predictive analytics powered by AI can optimize patient care and regulatory oversight.
Real-Time Surveillance and Monitoring:
AI enables real-time surveillance and monitoring of medical device safety, providing stakeholders with timely insights into potential safety issues. AI algorithms can continuously analyze incoming data streams, such as electronic health records, wearable device data, and social media posts, to identify safety signals and trends as they emerge. By leveraging natural language processing and sentiment analysis, AI systems can monitor online forums and social media platforms to detect patient-reported adverse events and sentiments related to specific medical devices. This real-time surveillance capability enables regulators and healthcare providers to respond promptly to safety concerns and take proactive measures to protect patient safety.
Automated Adverse Event Detection and Reporting:
AI automates adverse event detection and reporting processes, streamlining data collection and analysis for regulatory reporting purposes. Natural language processing algorithms can parse unstructured text data from electronic health records, clinical notes, and adverse event reports to extract relevant information and classify events according to predefined criteria. By automating these tasks, AI-powered systems reduce the burden on healthcare professionals and regulatory authorities, enabling them to focus their efforts on more critical tasks, such as risk assessment and regulatory decision-making. Moreover, automated adverse event detection and reporting improve the efficiency and accuracy of safety monitoring, leading to faster identification and response to safety concerns.
Challenges and Considerations:
While AI offers significant potential to enhance monitoring for medical device safety, several challenges and considerations must be addressed. These include issues related to data quality, algorithm bias, interpretability of results, privacy concerns, and regulatory compliance. Ensuring transparency and accountability in AI-driven decision-making processes is essential to maintain trust and credibility among stakeholders. Moreover, ongoing validation and validation of AI models are necessary to verify their performance and reliability in real-world settings. By addressing these challenges and considerations, stakeholders can maximize the benefits of AI in medical device safety monitoring while minimizing potential risks and drawbacks.
Conclusion:
AI is revolutionizing medical device safety monitoring by enabling more efficient, proactive, and data-driven approaches to identify and mitigate safety risks. By leveraging advanced analytics, predictive modeling, real-time surveillance, and automated adverse event detection, AI-powered systems empower regulators and healthcare providers to monitor medical device safety more effectively and protect patient health. However, realizing the full potential of AI in medical device safety monitoring requires addressing various challenges and considerations, including data quality, algorithm bias, interpretability, privacy, and regulatory compliance. Through collaboration, innovation, and continuous improvement, stakeholders can harness the power of AI to enhance patient care and regulatory oversight in the dynamic landscape of healthcare.
Comments