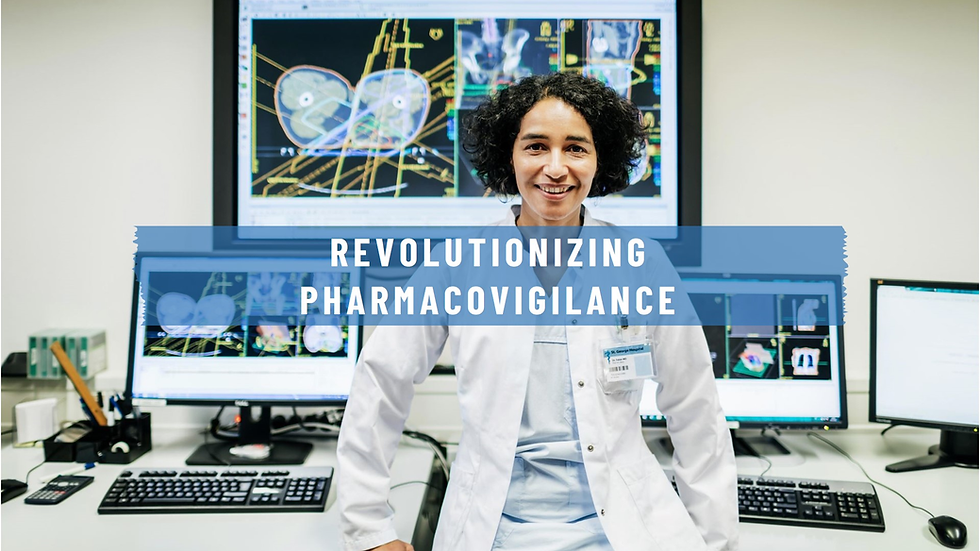
The advent of Artificial Intelligence (AI) has been transformative across various industries, with healthcare and pharmaceuticals being no exception. One of the most critical aspects of pharmaceutical safety is pharmacovigilance, which involves monitoring the effects of drugs after they have been released to the market. Post-market surveillance in pharmacovigilance is a vital process for ensuring drug safety, identifying adverse drug reactions (ADRs), and mitigating risks associated with medicinal products. Traditionally, this process has relied on manual reporting, data analysis, and periodic review, but AI is rapidly changing the landscape. This blog explores how AI enhances post-market surveillance in pharmacovigilance, offering increased efficiency, accuracy, and proactivity in drug safety monitoring.
Understanding Post-Market Surveillance in Pharmacovigilance:
Post-market surveillance refers to the continuous monitoring of drugs once they are available to the public. Unlike pre-market clinical trials, which involve controlled environments and limited populations, post-market surveillance covers a broader, more diverse patient population over a longer period. This stage is crucial for identifying rare or long-term adverse effects that might not have been evident during clinical trials.
Pharmacovigilance activities during post-market surveillance include:
Adverse Drug Reaction (ADR) Reporting: Collecting reports from healthcare professionals, patients, and regulatory agencies about adverse reactions linked to drug use.
Signal Detection: Identifying potential safety signals, which are pieces of evidence that suggest a new, potentially causal relationship between a drug and an adverse event.
Risk Management: Developing and implementing strategies to minimize identified risks, including updating drug labels, issuing warnings, or, in extreme cases, withdrawing a drug from the market.
Regulatory Compliance: Ensuring that all activities comply with regulatory requirements and guidelines set by bodies such as the U.S. Food and Drug Administration (FDA) and the European Medicines Agency (EMA).
The traditional approach to post-market surveillance is often reactive, relying heavily on voluntary reporting and retrospective analysis. However, with the integration of AI, pharmacovigilance is becoming more proactive, predictive, and precise.
The Role of AI in Enhancing Post-Market Surveillance:
AI, with its capabilities in machine learning (ML), natural language processing (NLP), and data mining, offers significant enhancements to various aspects of post-market surveillance. These technologies enable more effective data analysis, faster signal detection, and better risk management strategies.
Data Collection and Integration
AI facilitates the collection and integration of vast amounts of data from multiple sources, including electronic health records (EHRs), social media, clinical trials, patient registries, and spontaneous reporting systems. Traditionally, data collection has been fragmented and time-consuming, but AI can streamline this process by automatically extracting relevant information and integrating it into a centralized database for analysis.
For example, NLP algorithms can scan through unstructured data, such as clinical notes and social media posts, to identify mentions of adverse drug reactions that might otherwise go unnoticed. This real-time data collection allows for a more comprehensive view of a drug's safety profile in the real world.
Advanced Signal Detection
One of the most critical functions of post-market surveillance is signal detection. AI enhances this process by using machine learning algorithms to identify patterns and correlations in large datasets that human analysts might miss. These algorithms can analyze thousands of variables simultaneously, detecting potential safety signals more quickly and accurately than traditional methods.
For instance, AI can apply supervised and unsupervised learning techniques to detect unusual patterns of adverse events that may indicate a new safety concern. By continuously learning from new data, AI systems can adapt and improve over time, leading to more robust signal detection capabilities.
Predictive Analytics
AI's predictive analytics capabilities are transforming post-market surveillance from a reactive to a proactive process. Instead of waiting for adverse events to be reported, AI can predict potential safety issues before they occur. Predictive models can analyze historical data, patient demographics, genetic information, and drug characteristics to identify patients who are at higher risk of experiencing adverse reactions.
This predictive approach enables healthcare providers and pharmaceutical companies to take preemptive measures, such as adjusting dosages, providing additional monitoring, or recommending alternative treatments for high-risk patients. Ultimately, this reduces the likelihood of adverse events and improves patient safety.
Automation of Routine Tasks
Post-market surveillance involves many routine tasks, such as data entry, report generation, and regulatory submissions. AI-powered automation can significantly reduce the time and resources required for these tasks. For example, AI can automatically generate periodic safety update reports (PSURs) by compiling data from various sources, analyzing trends, and summarizing findings.
Automation also reduces the risk of human error, ensuring that reports are accurate and compliant with regulatory requirements. This allows pharmacovigilance teams to focus on more strategic activities, such as risk management and decision-making.
Natural Language Processing (NLP) for Case Processing
Case processing is a labor-intensive part of pharmacovigilance, involving the review and classification of individual adverse event reports. NLP, a subset of AI, can enhance this process by automatically extracting relevant information from unstructured text, such as patient narratives and healthcare provider notes.
NLP algorithms can identify key details, such as drug names, symptoms, and outcomes, and classify cases based on severity and likelihood of causality. This accelerates the case processing workflow and ensures that potential safety signals are identified and addressed promptly.
Improved Risk Management
AI supports more effective risk management by providing a deeper understanding of drug safety profiles and patient populations. AI algorithms can analyze data to identify subpopulations that may be more susceptible to certain adverse reactions, allowing for targeted risk minimization strategies.
For example, AI can identify genetic markers or comorbidities that increase the risk of an adverse reaction in specific patient groups. This information can be used to update drug labels, develop targeted patient education materials, or implement additional monitoring for at-risk populations.
Regulatory Compliance and Reporting
Regulatory compliance is a critical aspect of post-market surveillance, requiring timely and accurate reporting of adverse events to regulatory bodies. AI can streamline the reporting process by automating the generation of regulatory submissions and ensuring that all required information is included.
AI can also monitor regulatory updates and guidelines, automatically adjusting reporting processes to comply with new requirements. This ensures that pharmaceutical companies remain compliant with evolving regulations and avoid costly penalties.
Real-Time Monitoring and Decision Support
AI enables real-time monitoring of drug safety by continuously analyzing data as it becomes available. This allows for the rapid identification of emerging safety concerns and the prompt implementation of risk mitigation strategies.
In addition to real-time monitoring, AI can provide decision support by offering insights and recommendations based on the latest data. For example, AI algorithms can suggest changes to treatment protocols or identify alternative therapies for patients experiencing adverse reactions. This support enhances the decision-making process and improves patient outcomes.
Case Studies: AI in Action in Post-Market Surveillance:
Several case studies illustrate the impact of AI on post-market surveillance in pharmacovigilance:
Case Study 1: AI-Driven Signal Detection
A global pharmaceutical company implemented an AI-powered signal detection system to enhance its post-market surveillance efforts. The system analyzed vast amounts of data from spontaneous reporting systems, EHRs, and social media to identify potential safety signals. Within the first year, the AI system detected several new safety concerns that were not previously identified through traditional methods, leading to early interventions and improved patient safety.
Case Study 2: Predictive Analytics for Risk Management
A biotech firm leveraged AI’s predictive analytics capabilities to identify patients at high risk of adverse reactions to a new cancer drug. By analyzing patient data, the AI system identified specific genetic markers that increased the likelihood of severe side effects. The company used this information to develop a companion diagnostic test, ensuring that the drug was only prescribed to patients with a lower risk profile, thereby reducing adverse events.
Case Study 3: NLP for Case Processing
A large pharmaceutical company implemented NLP algorithms to automate the processing of adverse event reports. The NLP system extracted relevant information from unstructured text, such as patient narratives, and automatically classified cases based on severity. This reduced the time required for case processing by 50%, allowing the pharmacovigilance team to focus on more complex cases and signal detection activities.
Challenges and Considerations in Implementing AI in Post-Market Surveillance:
While AI offers significant benefits for post-market surveillance, its implementation is not without challenges. Pharmaceutical companies and regulatory bodies must consider several factors to ensure successful AI adoption:
Data Quality and Integration
AI systems rely on high-quality data for accurate analysis and decision-making. Ensuring that data is accurate, complete, and consistent across sources is critical for the success of AI-driven post-market surveillance. Data integration from disparate systems can also be challenging, requiring robust data management strategies.
Regulatory Acceptance
AI’s use in pharmacovigilance is still relatively new, and regulatory agencies may have concerns about the transparency and interpretability of AI-driven decisions. Companies must work closely with regulators to ensure that AI systems meet regulatory standards and that decisions made by AI are explainable and justifiable.
Ethical Considerations
AI systems must be designed and implemented with ethical considerations in mind, particularly when dealing with sensitive patient data. Companies must ensure that AI algorithms do not introduce biases or discrimination and that patient privacy is protected.
Skilled Workforce
The successful implementation of AI in post-market surveillance requires a skilled workforce with expertise in both AI technologies and pharmacovigilance. Companies must invest in training and development to build the necessary skills within their teams.
Cost and Resource Allocation
Implementing AI can be resource-intensive, requiring significant investment in technology, infrastructure, and personnel. Companies must carefully evaluate the costs and benefits of AI adoption and allocate resources accordingly.
Conclusion:
As AI continues to evolve, its role in post-market surveillance will undoubtedly expand, offering even greater enhancements to pharmacovigilance practices. The integration of AI in this field is transforming the way pharmaceutical companies monitor drug safety, shifting from reactive approaches to proactive and predictive models. By enabling real-time monitoring, advanced signal detection, and personalized risk management, AI is helping to ensure that drugs remain safe and effective throughout their lifecycle.
However, the successful implementation of AI in post-market surveillance requires careful consideration of data quality, regulatory compliance, ethical concerns, and workforce readiness. As the technology matures and becomes more widely accepted by regulatory bodies, its potential to revolutionize pharmacovigilance will become increasingly apparent.
In the future, AI is likely to become an indispensable tool in pharmacovigilance, driving continuous improvements in drug safety and patient outcomes. As pharmaceutical companies and healthcare providers embrace AI-driven solutions, they will be better equipped to manage the complexities of post-market surveillance, ultimately leading to safer, more effective therapies for patients worldwide.
Comentários