How AI Enhances Predictive Modelling for Vaccine Safety
- Chaitali Gaikwad
- Oct 7, 2024
- 6 min read
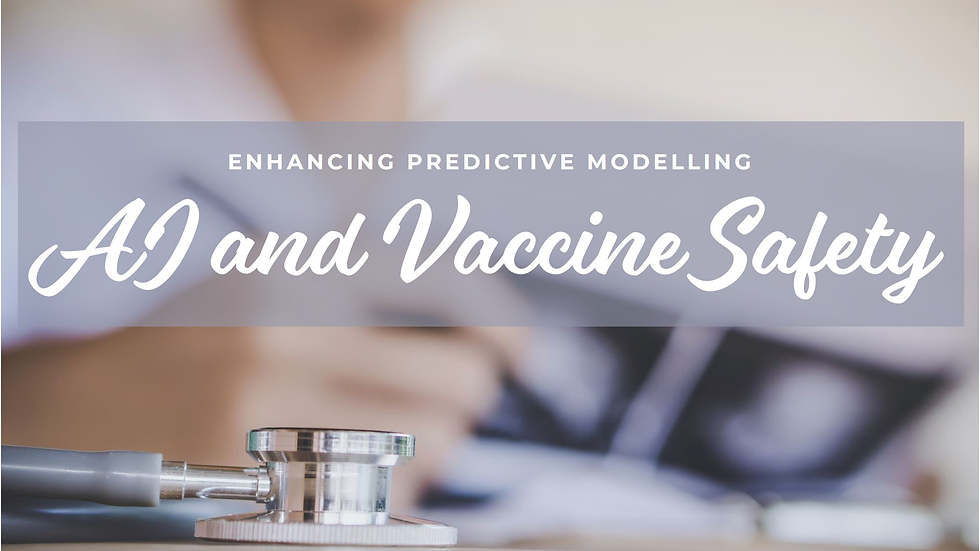
The development and deployment of vaccines have proven to be one of the most effective public health strategies in combating infectious diseases. However, as the complexity of vaccines increases and their use expands globally, the need for robust safety monitoring becomes paramount. Predictive modeling, enhanced by artificial intelligence (AI), has emerged as a powerful tool to improve vaccine safety assessments. This blog explores how AI enhances predictive modeling for vaccine safety, the methodologies employed, and the implications for public health.
Understanding Predictive Modeling in Vaccine Safety:
Predictive modeling in vaccine safety involves using statistical techniques and algorithms to analyze historical data and identify potential safety signals. This process allows health authorities and manufacturers to predict and evaluate the risk of adverse events following vaccination. Predictive models can help in various ways:
Signal Detection: Identifying adverse events that may not have been previously recognized.
Risk Assessment: Estimating the likelihood of specific adverse events occurring in various populations.
Safety Monitoring: Continuously assessing vaccine safety as new data becomes available.
While traditional predictive modeling techniques have been useful, they often struggle to process large volumes of data or identify complex patterns. This is where AI comes into play.
The Role of AI in Predictive Modeling for Vaccine Safety:
AI, particularly through machine learning (ML) and deep learning techniques, can analyze vast datasets at unprecedented speeds and accuracy. Here are several ways in which AI enhances predictive modeling for vaccine safety:
1. Handling Large and Complex Datasets
Vaccine safety monitoring generates massive amounts of data from various sources, including clinical trials, post-marketing surveillance, electronic health records, and social media. AI algorithms can process this data efficiently, identifying patterns and trends that may be invisible to traditional methods.
For instance, machine learning models can analyze millions of adverse event reports from databases like the Vaccine Adverse Event Reporting System (VAERS) to detect signals of potential safety concerns. By leveraging natural language processing (NLP), AI can also analyze unstructured data, such as clinical notes or social media posts, to identify adverse events that may not be reported in formal channels.
2. Improving Signal Detection
AI algorithms excel at detecting anomalies within datasets, making them particularly well-suited for signal detection in vaccine safety. Traditional statistical methods often require predefined hypotheses, limiting their ability to identify unexpected adverse events. In contrast, AI can analyze data without prior assumptions, uncovering hidden safety signals.
For example, deep learning models can learn complex relationships within data, identifying patterns that might indicate a potential safety issue. By employing techniques such as ensemble learning, where multiple models are combined, AI can enhance the accuracy of signal detection, reducing false positives and negatives.
3. Enhancing Risk Prediction
AI can significantly improve risk prediction for adverse events associated with vaccines. Traditional models often rely on historical data and established risk factors, which may not account for new variables or changing demographics. AI algorithms can analyze vast amounts of data, considering multiple variables simultaneously, and generate more accurate risk predictions.
For instance, AI can incorporate factors such as patient demographics, comorbidities, and genetic predispositions to create personalized risk profiles. This level of granularity allows for more targeted safety monitoring and can help identify high-risk populations that may require additional oversight.
4. Continuous Learning and Adaptation
One of the most significant advantages of AI in predictive modeling is its ability to continuously learn and adapt. Traditional models often require retraining or recalibration when new data becomes available. In contrast, AI algorithms can incorporate new information in real-time, enhancing their predictive capabilities.
This continuous learning process is particularly valuable in the context of vaccine safety, where data is constantly being generated from ongoing immunization campaigns and post-marketing surveillance. AI can adjust its predictions based on the latest data, ensuring that safety assessments remain relevant and accurate.
5. Improving Decision-Making
AI-enhanced predictive modeling can significantly improve decision-making processes related to vaccine safety. By providing real-time insights and forecasts, AI can help health authorities and manufacturers make informed decisions about vaccine deployment, safety monitoring, and risk management.
For example, predictive models powered by AI can assist in prioritizing safety investigations by identifying which vaccines or populations are at higher risk for specific adverse events. This targeted approach allows for more efficient resource allocation and timely interventions.
6. Facilitating Collaborative Approaches
AI can also facilitate collaborative approaches to vaccine safety monitoring by enabling data sharing among various stakeholders, including public health agencies, healthcare providers, and researchers. AI algorithms can integrate and analyze data from diverse sources, providing a more comprehensive view of vaccine safety.
This collaborative model can help build a more robust safety surveillance system, where findings from one jurisdiction can inform practices in others. AI’s ability to analyze data in real-time allows for quicker responses to emerging safety signals, enhancing the overall safety of vaccination programs.
Case Studies of AI in Vaccine Safety
Several case studies illustrate the successful application of AI in enhancing predictive modeling for vaccine safety:
Case Study 1: Monitoring COVID-19 Vaccine Safety
The rapid rollout of COVID-19 vaccines created an urgent need for effective safety monitoring. Researchers used AI-driven predictive modeling to analyze data from multiple sources, including VAERS and electronic health records, to detect potential adverse events.
Using machine learning algorithms, they identified signals related to specific adverse events, such as myocarditis and thrombosis, leading to timely public health communications and recommendations. This proactive approach allowed health authorities to address safety concerns quickly and transparently.
Case Study 2: Seasonal Influenza Vaccine Surveillance
AI has also been applied in monitoring the safety of seasonal influenza vaccines. Researchers developed machine learning models to analyze historical adverse event data and predict potential safety issues for specific demographics.
By incorporating real-world data and predictive analytics, these models successfully identified trends and risks associated with the influenza vaccine, allowing for timely adjustments to vaccination strategies and public health messaging.
Challenges and Limitations of AI in Predictive Modeling:
Despite its potential, the application of AI in predictive modeling for vaccine safety also faces several challenges:
Data Quality and Standardization: The effectiveness of AI algorithms relies heavily on the quality and consistency of the data. Variability in data collection methods, reporting standards, and data completeness can impact the accuracy of predictions.
Ethical Considerations: The use of AI in healthcare raises ethical concerns, particularly regarding data privacy and bias. Ensuring that algorithms are developed and deployed transparently and equitably is crucial to maintain public trust.
Regulatory Challenges: Regulatory frameworks for AI in healthcare are still evolving. Clear guidelines and standards are needed to ensure the safe and effective use of AI in vaccine safety monitoring.
Interpretability: Many AI algorithms, particularly deep learning models, can be complex and difficult to interpret. Ensuring that predictions can be understood and acted upon by public health professionals is essential for effective decision-making.
The Future of AI in Vaccine Safety:
The future of AI in predictive modeling for vaccine safety looks promising. As AI technologies continue to evolve, their applications in vaccine safety monitoring will likely expand. Key developments to watch for include:
Integration of Real-World Evidence: The incorporation of real-world data, including patient-reported outcomes and social media data, will enhance AI's ability to identify safety signals and assess risks.
Personalized Vaccine Safety Monitoring: As personalized medicine becomes more prevalent, AI may enable tailored vaccine safety monitoring approaches based on individual patient profiles.
Global Collaboration: Increased collaboration between public health agencies, manufacturers, and researchers will enhance the robustness of vaccine safety monitoring systems. AI can facilitate data sharing and analysis across borders, improving global vaccine safety efforts.
Improved Regulatory Frameworks: As the regulatory landscape adapts to the advancements in AI, clearer guidelines will emerge, ensuring the safe and effective application of AI in vaccine safety monitoring.
Conclusion:
AI has the potential to revolutionize predictive modeling for vaccine safety, enhancing the ability to detect signals, assess risks, and improve decision-making. By leveraging the power of AI, public health authorities and vaccine manufacturers can ensure a more robust and responsive safety monitoring system.
As we continue to navigate the complexities of vaccine safety in an increasingly interconnected world, embracing AI-driven predictive modeling will be essential to safeguard public health and enhance the overall safety of vaccination programs. With the right investment in data quality, ethical considerations, and regulatory frameworks, AI can significantly contribute to the safety and efficacy of vaccines, ultimately leading to better health outcomes for populations worldwide.
Commenti