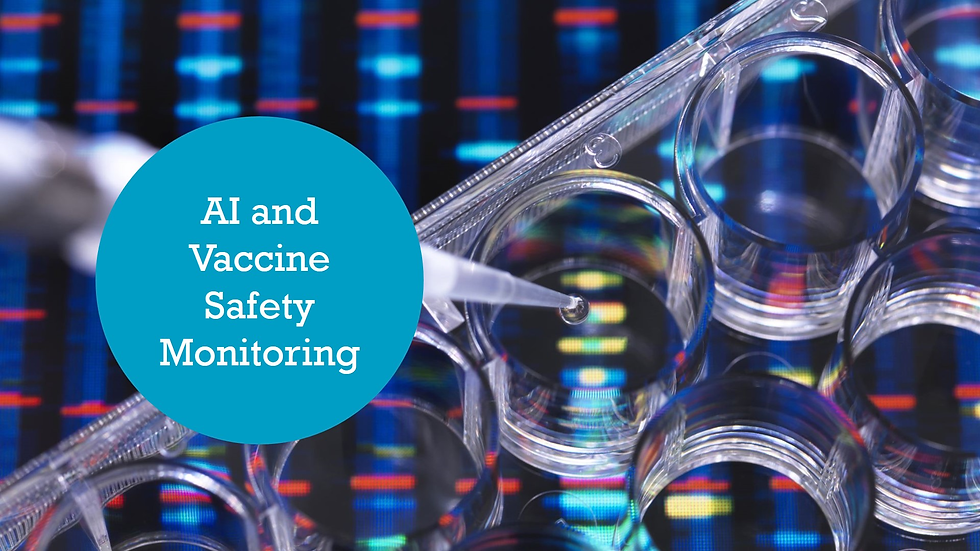
Vaccine safety monitoring is a crucial part of public health, ensuring that vaccines administered to the population are both safe and effective. With the global rollout of vaccines, especially during events like the COVID-19 pandemic, the need for fast, reliable, and accurate vaccine safety monitoring systems has never been greater. Predictive modeling, powered by Artificial Intelligence (AI), is transforming how vaccine safety is assessed and monitored. By leveraging AI algorithms, healthcare organizations and regulatory bodies can predict potential safety issues before they become widespread, thereby enhancing the overall safety of vaccines.
This blog explores the role of AI in predictive modeling for vaccine safety monitoring, examining its benefits, key applications, and challenges.
The Need for Predictive Modeling in Vaccine Safety Monitoring
Vaccines undergo extensive clinical testing before they are approved for public use. However, once they are widely administered, it becomes vital to continuously monitor their safety in real-world conditions. This process involves tracking adverse events and evaluating how vaccines interact with different population groups, such as those with pre-existing conditions or unique genetic profiles. Traditional methods of post-marketing surveillance often rely on passive reporting systems, like the Vaccine Adverse Event Reporting System (VAERS) in the United States, where healthcare professionals and the public can report adverse events.
While these systems are valuable, they are reactive, not predictive. Reports are typically submitted after an event has occurred, which delays corrective action. AI-based predictive modeling, on the other hand, can analyze vast amounts of data in real time, identify patterns, and predict potential adverse events before they occur, offering a proactive approach to vaccine safety monitoring.
How AI Enhances Predictive Modeling in Vaccine Safety
1. Big Data Integration and Analysis
One of the primary benefits of AI in predictive modeling is its ability to integrate and analyze large datasets from various sources, including clinical trials, electronic health records (EHRs), patient registries, and post-market surveillance systems. By processing these massive datasets, AI algorithms can uncover complex relationships and patterns that may not be immediately evident through traditional methods.
For example, AI can analyze genetic data to identify whether individuals with certain genetic markers are more likely to experience adverse reactions to a vaccine. It can also evaluate environmental factors, such as geographical location or exposure to other medications, to predict how these variables influence vaccine safety.
Moreover, AI can integrate global data, combining safety information from different countries and healthcare systems to create a comprehensive view of a vaccine’s safety profile. This enables regulatory bodies to make more informed decisions and ensures that vaccines are safe for diverse populations across the world.
2. Real-Time Data Processing
AI excels in real-time data processing, which is crucial for timely vaccine safety monitoring. Traditional systems may have a significant time lag between data collection, analysis, and reporting, which can delay the identification of safety signals. AI-based predictive models can analyze real-time data streams, detecting potential safety concerns almost instantaneously.
For instance, AI algorithms can continuously monitor vaccine-related data from various sources like social media, healthcare apps, wearable devices, and online forums. By processing this data in real time, AI can identify emerging patterns of adverse events and flag them for further investigation. This reduces the time it takes to respond to safety issues and can lead to quicker interventions, such as revising vaccine recommendations or issuing warnings to specific populations.
3. Machine Learning for Pattern Recognition
Machine learning (ML), a subset of AI, is particularly effective for identifying patterns and correlations in large, complex datasets. In vaccine safety monitoring, ML models can be trained on historical data to recognize patterns associated with adverse events. As new data is collected, these models continuously learn and refine their predictions, becoming more accurate over time.
For example, if certain adverse events are more likely to occur in older adults or people with autoimmune diseases, ML models can detect these patterns early. Once the models are trained, they can predict which population groups are at higher risk of adverse events following vaccination. This allows healthcare providers and regulatory agencies to take proactive measures, such as offering additional screening or altering vaccination schedules for at-risk groups.
Additionally, ML can help identify long-term effects of vaccines that may not have been apparent during initial clinical trials. By continuously analyzing post-market data, ML models can reveal delayed adverse events and provide insights that contribute to the long-term safety and efficacy of vaccines.
4. Predicting Rare Adverse Events
One of the challenges of vaccine safety monitoring is identifying rare adverse events. These events may only occur in a small percentage of the population, making them difficult to detect using traditional methods. AI can help by analyzing data from a broad range of sources and detecting rare but significant patterns.
For instance, after the rollout of the COVID-19 vaccines, there were concerns about rare blood clotting events linked to certain vaccines. AI-based models can scan vast datasets and detect such rare occurrences more efficiently than traditional statistical models. Once detected, the models can predict which individuals or population groups are at higher risk, enabling health authorities to take preventive measures, such as recommending alternative vaccines for certain groups.
5. Natural Language Processing (NLP) for Unstructured Data Analysis
Natural Language Processing (NLP) is another AI technique that is transforming vaccine safety monitoring. A significant amount of safety-related data is stored in unstructured formats, such as clinical notes, research papers, and social media posts. NLP algorithms can analyze these unstructured data sources, extracting valuable insights that would otherwise be missed.
For instance, NLP can be used to scan social media platforms for mentions of vaccine-related adverse events. By analyzing the language used in these posts, NLP models can determine whether the posts are related to genuine safety concerns and whether further investigation is needed. Additionally, NLP can be applied to medical records, extracting relevant information about vaccine reactions from free-text clinical notes.
6. AI for Post-Marketing Surveillance
Post-marketing surveillance is a critical phase of vaccine safety monitoring, as it assesses vaccine performance in real-world conditions. AI enhances post-marketing surveillance by automating the detection of safety signals and analyzing data from various sources, including spontaneous reporting systems, EHRs, and wearable devices.
AI-powered tools can automatically detect trends and anomalies in post-marketing data that might indicate a safety issue. For instance, if an unusually high number of adverse events are reported in a specific region, AI algorithms can flag the data for further investigation. This helps regulators respond swiftly to emerging safety concerns, potentially preventing widespread adverse events.
7. AI-Powered Predictive Risk Models
One of the most significant applications of AI in vaccine safety monitoring is the development of predictive risk models. These models use data from previous vaccinations, population demographics, and environmental factors to predict the likelihood of adverse events in different groups.
For example, AI-powered models can predict how a new vaccine will perform in specific populations, such as children, the elderly, or people with chronic health conditions. By understanding the risk profiles of different groups, healthcare providers can make more informed decisions about vaccine distribution and administration.
Predictive models also help regulators make informed decisions about vaccine approval, monitoring, and recall. If a model predicts a high risk of adverse events in a particular group, regulators may require additional testing or impose restrictions on vaccine use in that group.
8. Improving Clinical Trial Design
AI can also improve the design of clinical trials for vaccines, making them more efficient and reliable. By analyzing historical trial data, AI can help researchers design trials that are better suited to detecting safety signals. For example, AI can help determine the optimal sample size for detecting rare adverse events or recommend specific population groups to include in the trial to ensure that it accurately reflects the real-world population.
Additionally, AI can monitor ongoing clinical trials in real time, identifying potential safety issues before they escalate. This allows researchers to adjust trial protocols as needed, ensuring the safety of participants and the reliability of the results.
Challenges and Limitations of AI in Predictive Modeling for Vaccine Safety
While AI holds tremendous potential for enhancing predictive modeling in vaccine safety monitoring, there are several challenges and limitations to consider:
Data Quality and Availability: AI models rely on high-quality data to make accurate predictions. Inconsistent or incomplete data can lead to incorrect conclusions. Ensuring the availability of high-quality data from diverse sources is essential for the success of AI in vaccine safety monitoring.
Ethical Concerns: The use of AI in healthcare raises ethical concerns, particularly related to patient privacy and data security. Regulatory frameworks must be in place to ensure that AI is used responsibly and that patient data is protected.
Interpretability: AI models, particularly deep learning models, can be difficult to interpret. Healthcare providers and regulators may be hesitant to rely on predictions from models they do not fully understand. Developing interpretable AI models is crucial for gaining trust and ensuring regulatory compliance.
Regulatory Hurdles: The use of AI in vaccine safety monitoring is still in its early stages, and regulatory frameworks are evolving. Clear guidelines on how AI models should be validated and used in safety monitoring are needed to ensure their widespread adoption.
Conclusion
Artificial Intelligence is revolutionizing predictive modeling for vaccine safety monitoring by enabling real-time data analysis, identifying patterns in vast datasets, and predicting adverse events before they occur. From enhancing post-marketing surveillance to improving clinical trial design, AI has the potential to significantly improve vaccine safety. However, challenges related to data quality, ethics, and regulatory oversight must be addressed to fully realize the benefits of AI in this field. By leveraging AI responsibly and effectively, healthcare providers and regulators can ensure that vaccines remain one of the safest and most effective tools in public health.
Comments