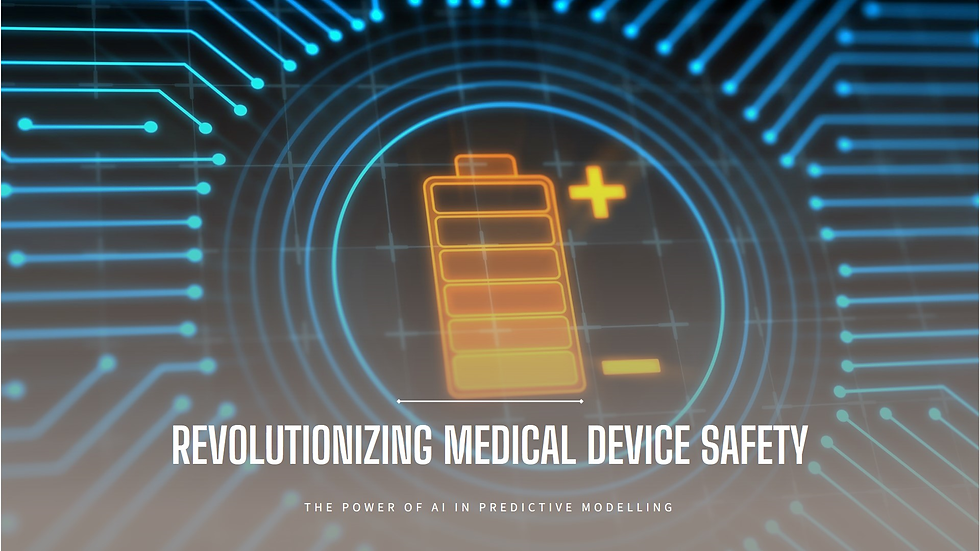
In the rapidly advancing world of healthcare, medical devices play an increasingly critical role in patient care. From simple instruments like thermometers to complex machinery like MRI scanners and pacemakers, medical devices are integral to diagnosing, monitoring, and treating various health conditions. However, with the growing complexity and use of these devices, ensuring their safety has become a paramount concern. Predictive modeling, powered by artificial intelligence (AI), is emerging as a transformative tool in this field, enhancing the ability to foresee and mitigate risks associated with medical devices.
The Importance of Medical Device Safety:
Medical device safety is crucial because these devices are directly involved in patient care. A failure or malfunction in a medical device can lead to serious consequences, including incorrect diagnoses, ineffective treatments, or even life-threatening situations. Regulatory bodies such as the U.S. Food and Drug Administration (FDA) and the European Medicines Agency (EMA) enforce stringent regulations to ensure that medical devices are safe for use.
Traditionally, medical device safety has been managed through reactive measures such as post-market surveillance and incident reporting. However, these approaches often come into play only after a problem has occurred. The need for a more proactive approach, one that can predict and prevent issues before they happen, is where predictive modeling enhanced by AI comes into focus.
What is Predictive Modeling?
Predictive modeling is a statistical technique used to create models that predict future outcomes based on historical data. In the context of medical device safety, predictive modeling involves analyzing data from various sources—such as device usage data, patient outcomes, and adverse event reports—to identify patterns that may indicate potential safety risks.
By analyzing these patterns, predictive models can forecast the likelihood of device failures, adverse events, or other safety-related issues. This allows manufacturers, healthcare providers, and regulators to take preventive measures, such as modifying device designs, adjusting usage protocols, or issuing warnings to users.
The Role of AI in Predictive Modeling:
Artificial intelligence (AI) significantly enhances predictive modeling by enabling the analysis of vast and complex datasets at speeds and accuracies that far exceed human capabilities. AI, particularly through machine learning (ML) and deep learning (DL) techniques, allows for more sophisticated and precise models that can identify subtle patterns and correlations in the data that traditional methods might miss.
Here are some of the ways AI enhances predictive modeling in medical device safety:
1. Improving Data Analysis Capabilities
AI can process and analyze enormous amounts of data from multiple sources, such as electronic health records (EHRs), medical device logs, clinical trials, and real-world evidence. This data is often unstructured and heterogeneous, making it difficult to analyze using traditional statistical methods. AI, particularly natural language processing (NLP) and machine learning, can sift through this data, extract relevant information, and identify patterns that indicate potential safety risks.
For example, AI can analyze data from thousands of patients using a particular medical device and identify common factors in cases where adverse events occurred. This might include patient demographics, device usage patterns, or environmental conditions. By understanding these factors, predictive models can better forecast potential risks and help manufacturers improve device designs or usage guidelines.
2. Enhancing Model Accuracy and Precision
Machine learning algorithms, particularly those based on deep learning, can improve the accuracy and precision of predictive models. Deep learning models, such as neural networks, can learn complex patterns in the data that simpler models might miss. This is particularly useful in medical device safety, where the relationships between variables can be highly complex and non-linear.
For instance, in predicting the failure of an implantable device like a pacemaker, a deep learning model might identify a combination of factors—such as battery wear, patient activity levels, and device settings—that together increase the risk of failure. This level of precision allows for more accurate risk predictions and more targeted interventions.
3. Real-Time Monitoring and Prediction
One of the most significant advantages of AI-enhanced predictive modeling is its ability to operate in real-time. By continuously monitoring data from connected medical devices and other sources, AI can provide real-time predictions about potential safety risks. This is particularly valuable in critical care settings, where immediate action is often necessary to prevent harm.
For example, AI algorithms can monitor real-time data from ventilators or infusion pumps and predict potential malfunctions before they occur. This allows healthcare providers to intervene before a device fails, reducing the risk of harm to the patient.
4. Personalizing Risk Predictions
AI can also personalize predictive models to individual patients, taking into account their unique characteristics, such as genetics, medical history, and lifestyle. This personalized approach to predictive modeling can enhance medical device safety by providing more accurate risk assessments for specific patients.
For instance, an AI model might predict that a particular patient is at higher risk of complications with a certain type of implant due to their medical history or genetic predispositions. This information can guide healthcare providers in selecting the most appropriate device for that patient, potentially preventing adverse outcomes.
5. Detecting Rare Adverse Events
Rare adverse events can be particularly challenging to predict due to their infrequency and the limited data available. However, AI’s ability to analyze large datasets and identify subtle patterns can help in detecting these rare events.
By aggregating data from multiple sources and using advanced algorithms, AI can identify correlations that might indicate a rare but serious adverse event associated with a particular device. This capability is crucial for ensuring the safety of medical devices, especially those used in critical or high-risk situations.
6. Continuous Learning and Model Adaptation
AI models are not static; they can continuously learn and adapt as new data becomes available. This means that predictive models can be regularly updated to reflect the latest information on device performance and safety.
For example, as new data on device failures or adverse events is reported, AI models can incorporate this information and refine their predictions. This continuous learning process ensures that predictive models remain accurate and relevant over time, improving the long-term safety of medical devices.
7. Supporting Regulatory Compliance
Regulatory bodies are increasingly recognizing the value of AI in enhancing medical device safety. Predictive models powered by AI can support regulatory compliance by providing manufacturers with insights into potential safety issues before they reach the market. This proactive approach can reduce the likelihood of costly recalls or regulatory penalties.
Moreover, AI can help manufacturers prepare for regulatory submissions by providing robust data analysis and predictive insights that demonstrate the safety and efficacy of their devices. This can streamline the approval process and ensure that devices meet the highest safety standards.
Case Studies: AI in Action:
Case Study 1: Predicting Cardiac Device Failures
One notable example of AI enhancing predictive modeling in medical device safety is in the monitoring of implantable cardiac devices, such as pacemakers and defibrillators. These devices are critical for patients with heart conditions, but they also pose significant risks if they malfunction.
AI models have been developed to analyze data from these devices, including heart rhythms, device settings, and patient activity levels. By identifying patterns that precede device failures, these models can predict potential issues before they occur. For instance, AI can detect subtle changes in heart rhythms or device performance that might indicate a battery failure or lead malfunction.
Healthcare providers can use these predictions to intervene early, such as by scheduling device replacements or adjusting settings to prevent malfunctions. This not only enhances patient safety but also improves the overall reliability of the devices.
Case Study 2: Enhancing MRI Safety:
Magnetic resonance imaging (MRI) machines are essential diagnostic tools, but they can pose safety risks, especially for patients with implants or other medical devices. AI has been used to enhance predictive modeling in MRI safety by analyzing data from previous scans and identifying factors that could lead to safety issues.
For example, AI models can predict the likelihood of an adverse event based on the type of implant, its location, and the specific settings used during the MRI scan. This allows radiologists to adjust protocols and settings to minimize risks, ensuring that patients receive safe and effective imaging.
Case Study 3: Monitoring Insulin Pumps:
Insulin pumps are life-saving devices for individuals with diabetes, but they require careful monitoring to ensure they deliver the correct dosage. AI-enhanced predictive models have been developed to monitor data from insulin pumps, including glucose levels, dosage history, and patient behavior.
These models can predict potential issues, such as pump malfunctions or incorrect dosages, before they lead to serious complications. For instance, AI can detect patterns in glucose level fluctuations that suggest the pump is delivering too much or too little insulin. This allows patients and healthcare providers to adjust treatment before an adverse event occurs.
The Future of AI in Medical Device Safety:
The use of AI in predictive modeling for medical device safety is still in its early stages, but it holds immense potential. As AI technologies continue to evolve, we can expect even more sophisticated models that provide more accurate and timely predictions.
Integration with IoT and Big Data:
The integration of AI with the Internet of Things (IoT) and big data analytics will further enhance predictive modeling capabilities. Connected medical devices can continuously generate and transmit data, which AI can analyze in real-time to predict and prevent safety issues.
For example, in a hospital setting, AI could monitor data from a network of connected devices, such as ventilators, infusion pumps, and monitors, to predict potential failures or adverse events. This could enable healthcare providers to take preventive action across the entire facility, improving patient safety on a broader scale.
Regulatory Acceptance and Standardization:
As AI becomes more integral to medical device safety, regulatory bodies are likely to establish standards and guidelines for the use of AI in predictive modeling. This will ensure that AI models meet rigorous safety and ethical standards, providing a framework for manufacturers to develop and deploy AI-enhanced predictive models.
Challenges and Considerations:
Despite its potential, the use of AI in predictive modeling for medical device safety also presents challenges. These include issues related to data privacy, the transparency of AI algorithms, and the need for robust validation and testing of AI models. Addressing these challenges will be crucial for the widespread adoption and success of AI in this field.
Conclusion:
AI is revolutionizing predictive modeling in medical device safety, offering new ways to predict and prevent risks before they become critical issues. By enhancing data analysis capabilities, improving model accuracy, enabling real-time monitoring, and supporting personalized risk predictions, AI is transforming how manufacturers and healthcare providers ensure the safety of medical devices.
As the healthcare industry continues to embrace AI, the potential for improving patient outcomes and reducing safety risks will only grow. AI-enhanced predictive modeling represents a significant step forward in the ongoing effort to ensure that medical devices are as safe and effective as possible, ultimately benefiting patients worldwide.
Comments