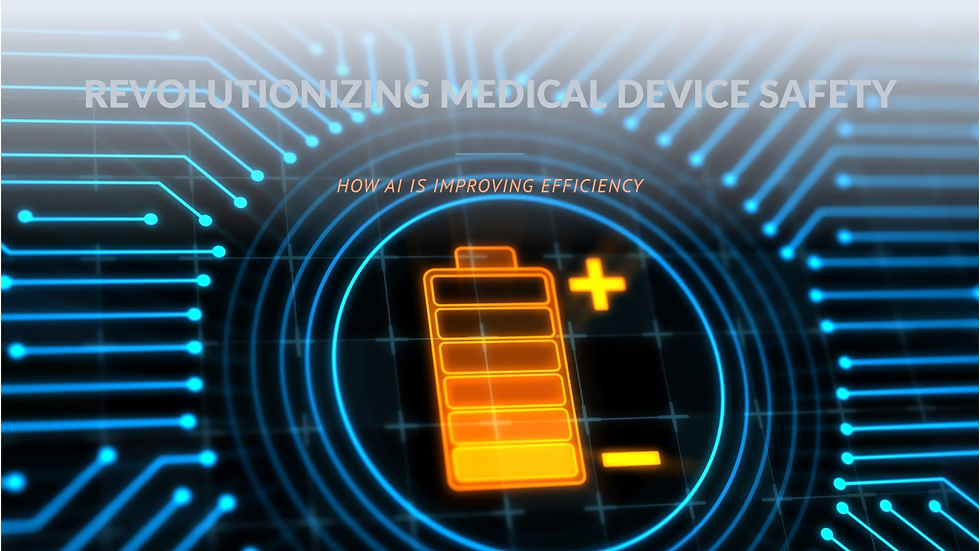
In the modern healthcare landscape, medical device safety is paramount. Medical devices range from simple tools like thermometers to complex machines such as MRI scanners and life-saving equipment like pacemakers. Ensuring the safety and reliability of these devices is crucial for patient health and trust in healthcare systems. Traditionally, managing the vast amounts of data associated with these devices has been labor-intensive and prone to human error. However, the advent of Artificial Intelligence (AI) is revolutionizing this field, significantly improving the efficiency, accuracy, and reliability of medical device safety databases.
Understanding Medical Device Safety Databases:
Medical device safety databases are repositories that collect and store data on the performance, usage, and safety of medical devices. This data includes:
Device performance metrics: Information on how well a device functions under various conditions.
Incident reports: Records of any malfunctions, failures, or adverse events associated with a device.
Maintenance records: Logs of repairs, updates, and routine maintenance activities.
Regulatory compliance documentation: Records ensuring that devices meet the necessary legal and safety standards.
These databases are essential for monitoring device safety, identifying potential issues, and ensuring compliance with regulatory bodies such as the FDA and the European Medicines Agency (EMA).
The Role of AI in Enhancing Efficiency:
AI brings a multitude of capabilities to the table that enhance the efficiency of medical device safety databases. These capabilities include data analysis, pattern recognition, predictive analytics, natural language processing (NLP), and automation. Let's explore how these AI technologies are applied to improve various aspects of managing medical device safety data.
1. Data Collection and Integration
One of the primary challenges in maintaining medical device safety databases is the sheer volume and diversity of data sources. AI can streamline the collection and integration of data from multiple sources, such as:
Electronic Health Records (EHRs): AI algorithms can extract relevant data from EHRs and integrate it into the safety database.
Device Logs: Many modern medical devices generate logs and usage data. AI can automate the extraction and aggregation of this data.
Incident Reports: AI can parse and categorize incident reports, even when they are in unstructured formats like free-text entries.
By automating data collection and integration, AI reduces the time and effort required to maintain comprehensive and up-to-date databases.
2. Data Cleaning and Normalization
Data from different sources often come in various formats and may contain inconsistencies or errors. AI-driven data cleaning and normalization ensure that the data is accurate and uniform, which is essential for reliable analysis. Techniques used include:
Anomaly Detection: AI algorithms can identify and correct anomalies or outliers in the data.
Standardization: AI can standardize data formats and units, ensuring consistency across the database.
Error Correction: AI tools can detect and correct common data entry errors, such as misspellings or incorrect dates.
This process improves the quality of the data, making it more useful for subsequent analysis.
3. Advanced Data Analysis and Pattern Recognition
AI excels at analyzing large datasets to identify patterns and trends that might be missed by human analysts. In the context of medical device safety, this capability is invaluable for:
Trend Analysis: AI can identify emerging trends in device performance or adverse events, allowing for proactive interventions.
Risk Assessment: By analyzing historical data, AI can predict the likelihood of future device failures or safety issues.
Comparative Analysis: AI can compare the performance of different devices or models, identifying which ones are more reliable or prone to issues.
These insights help healthcare providers and manufacturers improve device design, usage, and maintenance practices.
4. Predictive Maintenance
Predictive maintenance is a powerful application of AI in the realm of medical device safety. By analyzing data from device performance logs and maintenance records, AI can predict when a device is likely to fail or require maintenance. This allows for:
Preemptive Repairs: Scheduling maintenance before a device fails, reducing downtime and improving patient safety.
Resource Optimization: Ensuring that maintenance resources are used efficiently, focusing on devices that are most likely to need attention.
Extended Device Lifespan: Preventing failures that could shorten the lifespan of a device, thereby saving costs.
Predictive maintenance transforms reactive maintenance approaches into proactive strategies, enhancing overall device reliability.
5. Natural Language Processing (NLP) for Incident Reporting
Incident reports often contain valuable information but are written in unstructured, free-text formats. NLP, a branch of AI, can analyze and extract relevant information from these reports. Applications of NLP in this context include:
Automated Categorization: Classifying incident reports into predefined categories based on their content.
Sentiment Analysis: Assessing the sentiment or urgency of reports to prioritize responses.
Information Extraction: Extracting key details such as device names, symptoms, and outcomes from free-text reports.
NLP enhances the efficiency of processing incident reports, ensuring that critical information is quickly identified and addressed.
6. Regulatory Compliance and Reporting
Maintaining compliance with regulatory requirements is a significant aspect of managing medical device safety databases. AI can assist in this area by:
Automating Documentation: Generating and updating compliance documentation based on the latest data.
Ensuring Consistency: Verifying that all data entries and reports meet regulatory standards.
Facilitating Audits: Preparing data and reports for regulatory audits, ensuring that all necessary information is readily available.
By automating these tasks, AI reduces the administrative burden and ensures continuous compliance with evolving regulations.
7. Real-Time Monitoring and Alerts
AI enables real-time monitoring of device performance and safety data. By continuously analyzing incoming data, AI systems can detect anomalies or potential safety issues as they occur. This capability allows for:
Immediate Alerts: Notifying relevant personnel of potential safety issues or device malfunctions in real-time.
Rapid Response: Facilitating swift interventions to address issues before they escalate.
Continuous Improvement: Providing ongoing feedback to improve device design and usage practices.
Real-time monitoring enhances the responsiveness and effectiveness of safety management processes.
Case Studies and Examples:
Case Study 1: AI in Cardiac Device Monitoring
A leading manufacturer of cardiac devices implemented an AI-driven system to monitor device performance data from implanted pacemakers and defibrillators. The system analyzed data from millions of devices, identifying patterns indicative of potential malfunctions. As a result, the company could predict and address device failures before they affected patients, significantly improving patient safety and device reliability.
Case Study 2: NLP for Incident Report Analysis
A major hospital network deployed an NLP-based system to analyze incident reports related to medical devices. The system automatically categorized reports, extracted key details, and identified high-priority issues. This streamlined the incident reporting process, allowing the hospital to address safety concerns more quickly and efficiently.
Case Study 3: Predictive Maintenance in Imaging Equipment
A healthcare provider used AI to analyze performance and maintenance data from MRI and CT scanners. The AI system predicted when machines were likely to require maintenance, allowing the provider to schedule preventive maintenance and avoid unexpected downtime. This improved the availability and reliability of critical imaging equipment.
Future Trends and Developments:
The integration of AI in medical device safety databases is still evolving. Future trends and developments include:
1. Enhanced Interoperability
AI systems will increasingly support interoperability between different medical devices and databases, allowing for seamless data exchange and integration across platforms.
2. More Sophisticated Predictive Models
Advances in machine learning will lead to more sophisticated predictive models, improving the accuracy and reliability of predictions related to device performance and maintenance needs.
3. Greater Emphasis on Explain ability
As AI systems become more integral to safety management, there will be a greater emphasis on explain ability, ensuring that AI-driven decisions are transparent and understandable to human users.
4. Integration with Blockchain
Combining AI with blockchain technology could enhance data integrity and security, providing immutable records of device performance and safety data.
5. Personalized Device Monitoring
AI will enable more personalized monitoring and maintenance of medical devices, taking into account individual patient data and usage patterns to optimize device performance and safety.
Conclusion:
AI is transforming the management of medical device safety databases, bringing unprecedented efficiency, accuracy, and reliability. By automating data collection, cleaning, and analysis, AI reduces the administrative burden and enhances the quality of safety data. Predictive maintenance, real-time monitoring, and NLP for incident reporting further improve the management of medical device safety. As AI technology continues to evolve, it will play an increasingly vital role in ensuring the safety and reliability of medical devices, ultimately improving patient outcomes and trust in healthcare systems.
The integration of AI in medical device safety databases is not just a technological advancement but a crucial step towards a safer and more efficient healthcare environment. By harnessing the power of AI, healthcare providers and device manufacturers can ensure that medical devices continue to serve patients effectively and safely.
Commentaires