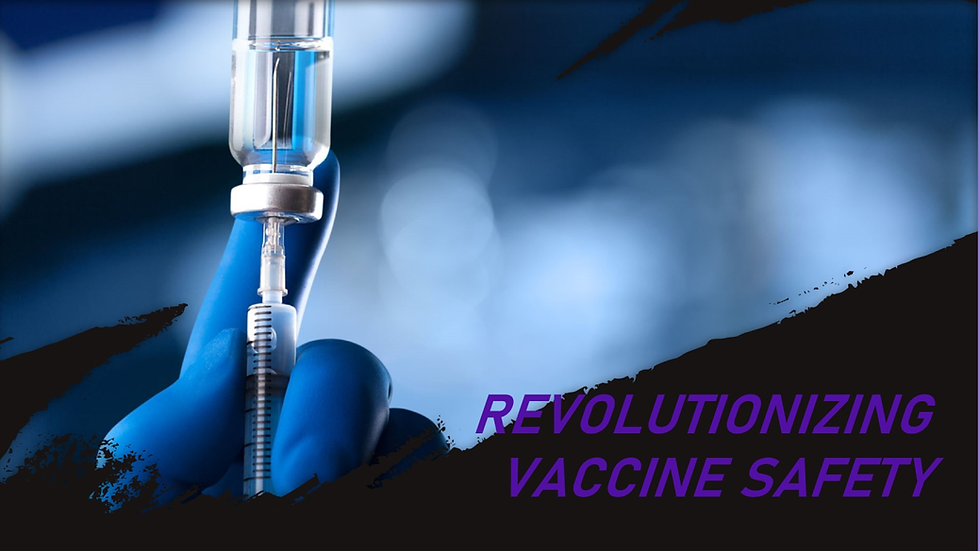
The advent of artificial intelligence (AI) has revolutionized numerous industries, and healthcare is no exception. One area where AI is making significant strides is in the management and analysis of vaccine safety databases. These databases are crucial for monitoring adverse events following immunization (AEFI) and ensuring that vaccines remain safe and effective post-licensure. However, managing these databases can be challenging due to the sheer volume of data and the complexity of identifying meaningful patterns. AI addresses these challenges by enhancing efficiency, accuracy, and responsiveness in vaccine safety surveillance. This blog explores how AI is transforming vaccine safety databases and the broader implications for public health.
The Role of Vaccine Safety Databases:
Before delving into AI's contributions, it's essential to understand the role of vaccine safety databases. These systems collect and analyze reports of adverse events following vaccination to detect potential safety issues. Key databases include:
Vaccine Adverse Event Reporting System (VAERS) in the United States.
Vaccine Safety Datalink (VSD), also in the United States.
Australia’s Adverse Drug Reactions Reporting System (ADRS).
These databases help regulatory agencies, healthcare providers, and researchers monitor vaccine safety, identify adverse events, and ensure vaccines' continued safety and efficacy. However, traditional methods of managing and analyzing data in these systems can be time-consuming and prone to errors. This is where AI comes into play.
Enhancing Data Collection and Entry:
AI significantly improves the efficiency of data collection and entry in vaccine safety databases. Traditional methods often rely on manual reporting and data entry, which can be slow and error-prone. AI automates these processes, ensuring that data is collected and entered accurately and quickly.
Automated Reporting Systems
AI-powered automated reporting systems streamline the process of collecting data from various sources. These systems can:
Extract Data from Electronic Health Records (EHRs): AI algorithms can automatically extract relevant data from EHRs, reducing the need for manual data entry and minimizing errors.
Natural Language Processing (NLP): NLP techniques enable AI to understand and process unstructured text data from various reports, converting it into structured data for analysis.
Real-Time Data Collection: AI systems can collect and process data in real-time, ensuring that vaccine safety databases are always up-to-date and reflective of the latest information.
Reducing Human Error
Manual data entry is susceptible to human error, which can compromise the integrity of vaccine safety databases. AI reduces these errors by:
Standardizing Data Entry: AI systems can standardize data entry processes, ensuring consistency and accuracy across all reports.
Validating Data: AI can validate data entries against predefined criteria, flagging any discrepancies or errors for further review.
Automated Data Cleaning: AI algorithms can identify and correct errors in the data, ensuring high-quality and reliable datasets.
Advanced Data Analysis and Signal Detection:
One of the most significant contributions of AI to vaccine safety databases is in the realm of data analysis and signal detection. Traditional methods of analyzing vaccine safety data can be labor-intensive and may not efficiently identify subtle patterns or rare adverse events. AI enhances these processes through advanced analytics and machine learning techniques.
Machine Learning for Signal Detection:
Machine learning (ML) algorithms excel at detecting patterns and anomalies in large datasets. In vaccine safety databases, ML can:
Identify Adverse Event Patterns: ML algorithms can analyze vast amounts of data to identify patterns and correlations between vaccines and adverse events, even those that are rare or occur over long periods.
Predictive Analytics: ML models can predict potential adverse events based on historical data, enabling proactive measures to be taken to mitigate risks.
Risk Stratification: AI can stratify patients based on their risk of experiencing adverse events, allowing for targeted monitoring and intervention.
Natural Language Processing (NLP) for Unstructured Data:
Vaccine safety databases often contain unstructured data, such as free-text reports from healthcare providers and patients. NLP techniques enable AI to:
Extract Meaningful Information: NLP algorithms can extract relevant information from unstructured text, such as symptoms, severity, and timing of adverse events.
Sentiment Analysis: NLP can assess the sentiment of reports, helping to identify trends and concerns that may not be immediately apparent from structured data alone.
Automated Coding: NLP can automate the process of coding adverse events according to standardized medical terminologies, such as the Medical Dictionary for Regulatory Activities (MedDRA).
Enhancing Efficiency in Signal Evaluation and Causality Assessment:
Once potential safety signals are detected, they need to be evaluated to determine if there is a causal relationship between the vaccine and the adverse event. This process traditionally requires significant human expertise and time. AI can enhance efficiency in this area through automated causality assessment and advanced analytics.
Automated Causality Assessment
AI can streamline the causality assessment process by:
Algorithmic Causality Models: Using predefined algorithms, AI can assess the likelihood of a causal relationship between a vaccine and an adverse event based on established criteria and historical data.
Bayesian Networks: These probabilistic models can evaluate complex relationships and dependencies between variables, providing a nuanced assessment of causality.
Expert Systems: AI can incorporate expert knowledge into its assessments, mimicking the decision-making process of human experts to provide reliable causality evaluations.
Advanced Analytics for In-Depth Analysis:
AI enables advanced analytics techniques that can provide deeper insights into vaccine safety data:
Survival Analysis: AI can perform survival analysis to evaluate the time-to-event data, helping to understand the timing and risk factors associated with adverse events.
Cluster Analysis: AI can identify clusters of similar adverse events, revealing patterns that may indicate underlying issues with specific vaccines or batches.
Data Visualization: AI-powered data visualization tools can present complex data in intuitive and interactive formats, making it easier for stakeholders to understand and act on the findings.
Improving Regulatory Decision-Making and Public Health Response:
AI’s ability to process and analyze vast amounts of data quickly and accurately has significant implications for regulatory decision-making and public health responses.
Real-Time Monitoring and Alerts
AI enables real-time monitoring of vaccine safety data, providing timely alerts when potential safety issues are detected. This allows regulatory agencies to:
Respond Quickly: Rapid detection and assessment of safety signals enable swift action to mitigate risks, such as issuing safety warnings or temporarily suspending vaccine batches.
Proactive Measures: Predictive analytics can identify potential risks before they become significant issues, allowing for proactive measures to protect public health.
Informed Decision-Making:
AI enhances the ability of regulatory agencies to make informed decisions by providing:
Comprehensive Risk Assessments: AI can integrate data from multiple sources to provide a comprehensive assessment of the risks associated with vaccines.
Scenario Analysis: AI can simulate different scenarios to evaluate the potential impact of various regulatory actions, helping to identify the most effective strategies.
Evidence-Based Recommendations: AI supports evidence-based decision-making by providing robust and reliable data analysis, ensuring that regulatory actions are based on the best available evidence.
Enhancing Transparency and Public Trust:
Transparency is crucial for maintaining public trust in vaccination programs. AI can enhance transparency by:
Open Data Access: AI can facilitate the sharing of anonymized data and analysis results with the public, researchers, and other stakeholders, promoting transparency and accountability.
Clear Communication: AI-powered natural language generation (NLG) can help translate complex data and analysis into clear and understandable language, improving communication with the public.
Engaging Visualizations: AI-driven data visualization tools can present data in engaging and accessible formats, helping to build trust by making information more comprehensible.
Challenges and Future Directions:
While AI offers significant benefits for vaccine safety databases, it also presents challenges that need to be addressed:
Data Quality and Integration: AI systems rely on high-quality data from diverse sources. Ensuring data quality and integrating data from various systems can be challenging but is crucial for effective AI implementation.
Algorithm Transparency: Ensuring that AI algorithms are transparent and explainable is essential for maintaining trust and accountability in AI-driven decision-making processes.
Ethical Considerations: The use of AI in vaccine safety databases must consider ethical issues, such as data privacy, informed consent, and potential biases in AI models.
Future Directions:
Advanced Machine Learning Techniques: Continued advancements in machine learning techniques, such as deep learning and reinforcement learning, will further enhance AI’s capabilities in vaccine safety surveillance.
Collaborative Platforms: Developing collaborative platforms that integrate AI with traditional epidemiological methods will improve the robustness and reliability of vaccine safety monitoring.
Global Data Sharing: Promoting global data sharing and collaboration will enable more comprehensive and effective monitoring of vaccine safety worldwide.
Conclusion:
AI is transforming the landscape of vaccine safety databases by enhancing efficiency, accuracy, and responsiveness in monitoring and analyzing adverse events following immunization. By automating data collection, improving data analysis, streamlining causality assessments, and supporting informed decision-making, AI significantly contributes to the safety and efficacy of vaccines. As AI technology continues to evolve, it will play an increasingly vital role in ensuring the success of vaccination programs and protecting public health globally. Embracing AI’s potential while addressing its challenges will be key to realizing its full benefits in vaccine safety surveillance.
Comentarios