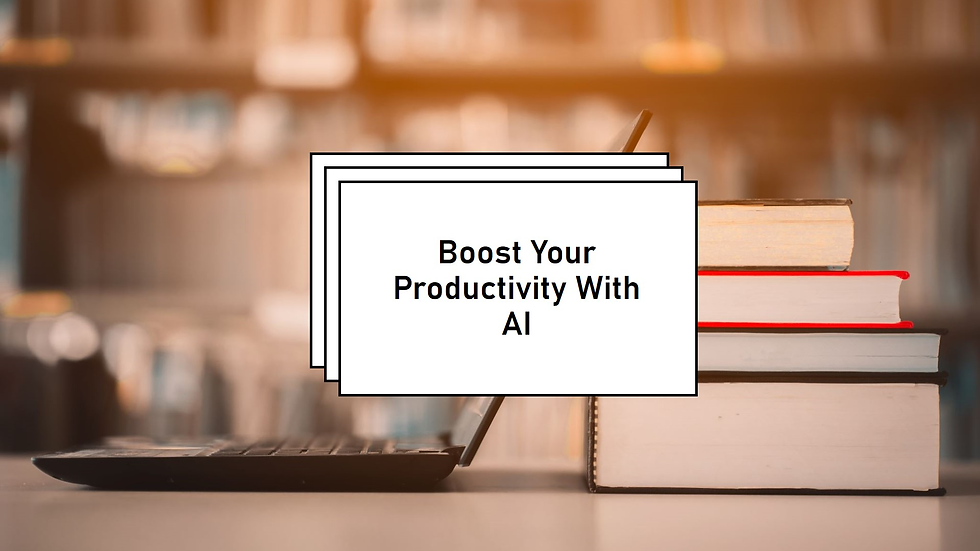
In today's fast-paced world, the sheer volume of published literature across various fields is staggering. For researchers, healthcare professionals, and academics, keeping up with the latest studies, articles, and papers is both essential and daunting. Literature monitoring, the process of systematically tracking and reviewing newly published works in a specific field, is critical for staying updated. However, traditional methods of literature monitoring are often time-consuming, labor-intensive, and prone to human error. Enter Artificial Intelligence (AI), a transformative technology that is revolutionizing the way we approach literature monitoring. AI-driven tools are enhancing productivity, accuracy, and efficiency in literature monitoring, making it easier for professionals to stay informed and make data-driven decisions.
The Traditional Challenges of Literature Monitoring:
Before delving into how AI improves productivity, it is essential to understand the challenges associated with traditional literature monitoring. These challenges include:
Volume of Information: The sheer quantity of new publications in any given field can overwhelm even the most diligent researcher. Manually sifting through hundreds or thousands of articles to find relevant information is both time-consuming and exhausting.
Relevance Filtering: Not all published material is relevant to every research query or area of interest. Identifying which articles are pertinent to a specific topic requires careful reading and analysis, which is not only labor-intensive but also requires significant expertise.
Timeliness: The rapid pace of new research means that by the time a human reader has reviewed the relevant literature, new studies may have already been published. This lag can delay the application of new knowledge in practical settings, particularly in fields like medicine or technology.
Human Error: Even the most experienced professionals can overlook critical studies or misinterpret data, leading to gaps in knowledge or incorrect conclusions. The human brain, while powerful, is not infallible, and the risk of error increases with fatigue and workload.
Bias: Human researchers may unintentionally introduce bias into their literature monitoring by focusing on familiar sources or overlooking certain types of studies. This can result in a skewed understanding of the current state of knowledge.
The Role of AI in Literature Monitoring:
Artificial Intelligence offers a solution to many of the challenges inherent in traditional literature monitoring. AI algorithms can process vast amounts of data quickly and accurately, identifying relevant studies, filtering out irrelevant material, and even summarizing findings. Here are some ways AI is transforming literature monitoring:
1. Automated Search and Retrieval
One of the most significant advantages of AI in literature monitoring is its ability to automate the search and retrieval of relevant studies. AI-driven tools can scan databases, journals, and online repositories to identify articles that match specific keywords or topics. This automation not only saves time but also ensures that no relevant studies are missed.
For example, AI can be used to create automated alerts that notify researchers when new studies in their field are published. These alerts can be customized to focus on specific topics, keywords, or authors, ensuring that researchers receive only the most relevant information. This level of automation significantly reduces the time spent on manual searches and allows researchers to focus on analyzing the data rather than finding it.
2. Advanced Text Mining and Natural Language Processing (NLP)
Text mining and Natural Language Processing (NLP) are critical components of AI that enhance literature monitoring. NLP allows AI to understand and interpret human language, making it possible to analyze the content of articles rather than just the metadata. This capability is particularly valuable for extracting relevant information from large volumes of text.
For instance, NLP can be used to identify key concepts, themes, and relationships within a body of literature. AI-driven tools can automatically categorize articles based on their content, making it easier for researchers to find studies that address specific questions. Moreover, NLP can help in detecting trends and patterns in the literature, providing insights that might not be immediately apparent through manual review.
3. Relevance Ranking and Filtering
AI can improve the relevance of literature monitoring by ranking articles based on their importance to a specific research query. This relevance ranking is achieved through algorithms that assess various factors, such as the number of citations, the prominence of the authors, and the impact factor of the journal in which the article was published.
Additionally, AI can filter out irrelevant or low-quality studies, ensuring that researchers only spend time on the most pertinent literature. This filtering process can be particularly useful in fields where the volume of published material is high, as it allows researchers to focus on the most significant contributions to their field.
4. Summarization and Data Extraction
One of the most time-consuming aspects of literature monitoring is reading and summarizing articles. AI can automate this process by generating summaries of studies, highlighting key findings, and extracting relevant data. This capability is particularly valuable for professionals who need to stay updated on the latest research but do not have the time to read every article in full.
AI-driven summarization tools use advanced algorithms to identify the most critical information within a text, such as the main conclusions, methods, and results. These summaries can be tailored to the needs of the user, providing a quick overview of the study's relevance to their research.
5. Trend Analysis and Predictive Analytics
AI is not only capable of monitoring current literature but can also analyze trends over time. By examining the evolution of research topics, AI can provide insights into emerging areas of interest and predict future developments in the field. This capability is particularly useful for researchers who need to anticipate changes in their domain and adapt their work accordingly.
Predictive analytics, powered by AI, can identify potential gaps in the literature and suggest areas where further research is needed. This foresight allows researchers to stay ahead of the curve and contribute to the advancement of their field.
6. Enhanced Collaboration and Knowledge Sharing
AI-driven tools can facilitate collaboration among researchers by providing platforms for sharing and discussing literature. These platforms can automatically recommend relevant studies to users based on their research interests and connect them with other researchers working on similar topics.
Moreover, AI can assist in the creation of shared databases and repositories, where researchers can contribute and access annotated bibliographies, reviews, and other resources. This collaborative approach to literature monitoring not only enhances productivity but also fosters a sense of community within the research field.
7. Reducing Bias and Increasing Objectivity
AI can help mitigate bias in literature monitoring by providing a more objective analysis of the literature. Algorithms can be designed to evaluate studies based on standardized criteria, reducing the influence of personal preferences or preconceived notions. This objectivity ensures that researchers have a more balanced and comprehensive understanding of the current state of knowledge.
Furthermore, AI can identify and correct for biases in the literature itself, such as publication bias or the overrepresentation of certain types of studies. By providing a more accurate picture of the research landscape, AI contributes to more informed and reliable decision-making.
Case Studies: AI in Action:
To illustrate the impact of AI on literature monitoring, let's explore a few real-world examples:
1. Healthcare and Medical Research
In the healthcare industry, staying updated on the latest research is critical for providing evidence-based care. AI-powered literature monitoring tools like IBM Watson for Health are being used to scan medical journals, clinical trial databases, and other sources of information. These tools can identify relevant studies, extract key data, and provide healthcare professionals with actionable insights.
For example, during the COVID-19 pandemic, AI-driven tools were used to monitor the rapidly evolving body of research on the virus. These tools helped healthcare providers stay informed about the latest treatments, vaccine developments, and public health recommendations, ultimately improving patient outcomes.
2. Pharmaceutical Industry
The pharmaceutical industry relies heavily on literature monitoring to track developments in drug research, safety, and regulatory compliance. AI-driven tools like Cortellis from Clarivate Analytics are used to monitor scientific literature, clinical trial results, and patent filings. These tools help pharmaceutical companies stay ahead of the competition by identifying potential opportunities and risks in the market.
By automating the process of literature monitoring, AI has enabled pharmaceutical companies to bring new drugs to market faster and more efficiently, ultimately benefiting patients and healthcare providers.
3. Academic Research
In academia, literature monitoring is essential for conducting comprehensive literature reviews and staying informed about the latest developments in a specific field. AI-driven tools like Researcher and EndNote are being used by academics to streamline the process of literature monitoring. These tools can automatically identify relevant studies, organize references, and generate bibliographies, saving researchers valuable time and effort.
By leveraging AI, academics can focus more on analyzing and interpreting data rather than spending hours on manual literature searches. This increased efficiency has the potential to accelerate the pace of scientific discovery and innovation.
The Future of AI in Literature Monitoring:
As AI continues to advance, its role in literature monitoring is expected to grow even more significant. Future developments in AI technology may include:
Enhanced Personalization: AI-driven tools will become increasingly adept at tailoring literature monitoring to the specific needs and preferences of individual researchers. This personalization will result in even more relevant and actionable insights.
Integration with Other Technologies: AI will likely be integrated with other technologies, such as blockchain for secure data sharing and virtual reality for immersive literature reviews. These integrations will further enhance the capabilities of AI-driven literature monitoring.
Continuous Learning: AI algorithms will continue to improve through continuous learning, becoming more accurate and efficient over time. This ongoing improvement will ensure that AI remains a valuable tool for literature monitoring in the years to come.
Ethical Considerations: As AI plays a more prominent role in literature monitoring, there will be an increased focus on ethical considerations, such as data privacy, algorithmic transparency, and the potential for AI to reinforce existing biases. Addressing these concerns will be critical to ensuring the responsible use of AI in literature monitoring.
Conclusion:
AI is revolutionizing the way we approach literature monitoring, offering significant improvements in productivity, accuracy, and efficiency. By automating time-consuming tasks, reducing human error.
Comments