How AI Simplifies Literature Review in Pharmacovigilance?
- Chaitali Gaikwad
- Aug 20, 2024
- 6 min read
Updated: Aug 21, 2024
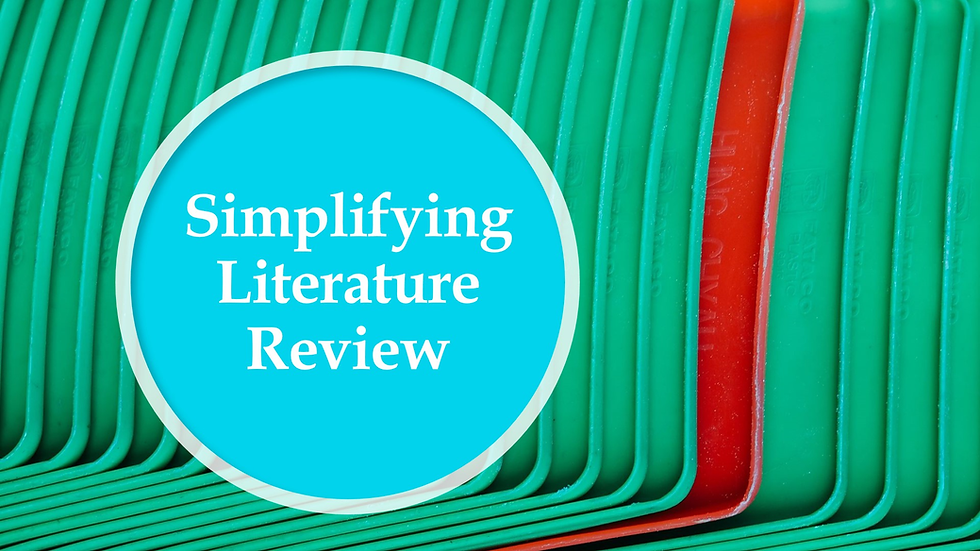
Pharmacovigilance is a crucial element in the lifecycle of pharmaceutical products, encompassing the detection, assessment, understanding, and prevention of adverse effects or any other drug-related problems. With the exponential growth of biomedical literature, ensuring compliance with pharmacovigilance regulations has become increasingly challenging. Traditional methods of literature review are often time-consuming and labor-intensive, posing a risk of missing critical safety signals that could have serious implications for public health and regulatory compliance.
Enter AI-driven literature review—a transformative approach that leverages artificial intelligence to streamline and enhance the process of monitoring and analyzing biomedical literature for pharmacovigilance purposes. By automating the review process and improving the accuracy of data extraction, AI-driven literature review offers a more efficient, reliable, and compliant solution for pharmacovigilance activities.
The Role of Pharmacovigilance in Drug Safety:
Pharmacovigilance plays a vital role in ensuring the safety of pharmaceutical products once they reach the market. It involves continuous monitoring of the safety profile of drugs, including the identification of adverse drug reactions (ADRs), interactions with other medications, and any other potential risks to patients. Regulatory authorities, such as the U.S. Food and Drug Administration (FDA) and the European Medicines Agency (EMA), mandate rigorous pharmacovigilance practices to protect public health.
One of the key components of pharmacovigilance is the systematic review of scientific literature. This includes monitoring peer-reviewed journals, conference abstracts, and other sources of medical information for new safety data related to a drug. The sheer volume of literature, combined with the need for timely and accurate analysis, makes this task increasingly complex.
Challenges of Traditional Literature Review in Pharmacovigilance:
Traditional literature review methods in pharmacovigilance are largely manual and rely on the expertise of trained professionals to identify relevant articles, extract data, and assess the significance of findings. These methods, while effective, are fraught with challenges:
Volume and Complexity of Data: The biomedical literature is growing at an unprecedented rate, with thousands of new articles published every day. This makes it difficult for human reviewers to keep up with the volume of information and identify all relevant safety data.
Time-Consuming Process: Manually reviewing literature is a labor-intensive and time-consuming process. It involves searching databases, screening articles, extracting relevant data, and analyzing the findings. This can lead to delays in identifying safety signals and reporting them to regulatory authorities.
Human Error: The manual nature of traditional literature review increases the risk of human error. Important safety information may be overlooked or misinterpreted, leading to incomplete or inaccurate safety assessments.
Inconsistent Results: Different reviewers may interpret the same data in different ways, leading to inconsistencies in the assessment of safety signals. This can result in variations in the reporting of adverse events and potentially compromise patient safety.
Regulatory Compliance: Ensuring compliance with regulatory requirements is critical in pharmacovigilance. However, the complexity of the literature review process and the potential for human error make it challenging to maintain consistent and accurate reporting.
The Emergence of AI-Driven Literature Review:
Artificial intelligence has emerged as a powerful tool to address the challenges associated with traditional literature review methods in pharmacovigilance. AI-driven literature review leverages machine learning algorithms, natural language processing (NLP), and other advanced technologies to automate and enhance the process of identifying and analyzing relevant scientific literature.
AI-driven literature review offers several key advantages over traditional methods:
Efficiency and Speed: AI can process vast amounts of data at a speed that far exceeds human capabilities. Machine learning algorithms can quickly search and screen large volumes of literature, identifying relevant articles and extracting key data points in a fraction of the time it would take a human reviewer.
Accuracy and Consistency: AI-driven literature review reduces the risk of human error by automating the data extraction and analysis process. Machine learning models can be trained to identify specific safety signals and extract relevant information with a high degree of accuracy. This ensures consistent results and reduces the likelihood of missing critical safety information.
Scalability: AI-driven literature review is highly scalable, making it possible to monitor a large number of drugs and therapeutic areas simultaneously. This is particularly important for pharmaceutical companies with extensive product portfolios and global operations.
Real-Time Monitoring: AI can be used to continuously monitor new publications in real-time, ensuring that safety data is captured and analyzed as soon as it becomes available. This enables faster identification of safety signals and more timely reporting to regulatory authorities.
Regulatory Compliance: AI-driven literature review can be configured to adhere to specific regulatory requirements, ensuring that all relevant safety data is captured and reported in accordance with guidelines. This helps to maintain compliance and reduces the risk of regulatory penalties.
How AI-Driven Literature Review Works:
AI-driven literature review involves several key steps, each of which is enhanced by the application of artificial intelligence:
Literature Search: AI algorithms can be trained to search multiple databases and sources of biomedical literature simultaneously. These algorithms can be configured to identify relevant articles based on specific keywords, drug names, therapeutic areas, and other criteria. This reduces the time and effort required to perform comprehensive literature searches.
Screening and Selection: Once the relevant articles have been identified, AI can assist in screening and selecting the most pertinent studies. Machine learning models can be trained to recognize specific types of studies (e.g., clinical trials, case reports) and prioritize them based on their relevance to pharmacovigilance.
Data Extraction: AI-driven literature review tools can automatically extract key data points from the selected articles, such as information on adverse drug reactions, patient demographics, dosage, and treatment outcomes. Natural language processing (NLP) techniques are particularly useful for extracting data from unstructured text.
Safety Signal Detection: AI algorithms can analyze the extracted data to identify potential safety signals. This may involve comparing the frequency and severity of adverse events across different studies or identifying patterns of drug interactions that could pose a risk to patients.
Reporting and Compliance: The results of the AI-driven literature review can be automatically compiled into reports that are formatted in accordance with regulatory requirements. These reports can be generated in real-time, ensuring that safety data is promptly reported to regulatory authorities.
Case Studies and Applications:
Several pharmaceutical companies and regulatory agencies have already begun to implement AI-driven literature review in their pharmacovigilance processes. Here are a few examples:
Crypta: Leveraging state-of-the-art Generative AI, Crypta is designed to streamline and automate critical processes in pharmacovigilance and medical literature management. Its innovative capabilities have positioned it as a leading tool in the industry, offering a comprehensive solution that enhances efficiency, accuracy, and compliance. It offers Automated Import of Literature Articles, Automated ICSR Detection, Auto-Generation of Summary of Analysis, Automated Translation of Abstracts and Full-Text Articles, Automated Highlighting of Key Details, Automated Highlighting of Key Details, Comprehensive Literature Review Support, Highly Customizable Workflows, Multilingual Support, Audit Trail and 21 CFR Part 11 Compliance.
GSK and IBM Watson: GlaxoSmithKline (GSK) partnered with IBM Watson to develop an AI-driven literature review tool for pharmacovigilance. The tool uses machine learning and NLP to analyze scientific literature and identify potential safety signals. GSK reported a significant reduction in the time required to perform literature reviews, as well as improvements in the accuracy and consistency of their safety assessments.
EMA’s Adaptive Signal Detection: The European Medicines Agency (EMA) has implemented an AI-based adaptive signal detection system that uses machine learning algorithms to analyze large volumes of safety data from various sources, including scientific literature. The system is designed to improve the detection of emerging safety signals and enhance the agency’s ability to respond to potential risks.
Bayer’s AI-Powered Pharmacovigilance Platform: Bayer has developed an AI-powered pharmacovigilance platform that integrates literature review, case management, and safety signal detection into a single system. The platform uses machine learning algorithms to automate the extraction of safety data from scientific literature and other sources, enabling faster and more accurate safety assessments.
The Future of AI-Driven Literature Review in Pharmacovigilance:
As AI technology continues to advance, the potential for AI-driven literature review in pharmacovigilance will only grow. Future developments may include:
Enhanced NLP Capabilities: Advances in natural language processing will enable AI to better understand and interpret complex biomedical text, improving the accuracy and reliability of data extraction and analysis.
Integration with Other Data Sources: AI-driven literature review could be integrated with other data sources, such as electronic health records (EHRs), social media, and real-world evidence, to provide a more comprehensive view of drug safety.
Predictive Analytics: AI-driven literature review could evolve to include predictive analytics, enabling pharmaceutical companies and regulatory agencies to anticipate potential safety issues before they arise and take proactive measures to mitigate risks.
Global Collaboration: As AI-driven literature review becomes more widely adopted, there will be opportunities for greater collaboration between pharmaceutical companies, regulatory agencies, and academic institutions. This could lead to the development of shared AI models and databases, further enhancing the efficiency and effectiveness of pharmacovigilance efforts.
Conclusion:
AI-driven literature review is revolutionizing the field of pharmacovigilance by addressing the challenges associated with traditional methods and ensuring compliance with regulatory requirements. By automating the process of identifying and analyzing relevant scientific literature, AI offers a more efficient, accurate, and scalable solution for monitoring drug safety. As AI technology continues to evolve, its role in pharmacovigilance will only become more critical, helping to protect public health and ensure the safe use of pharmaceutical products worldwide.
If you are interested to know more about Crypta, please book a demo.
Comments